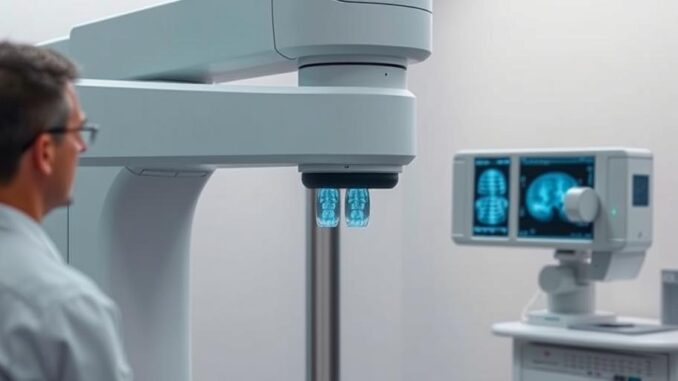
Abstract
Mammography stands as a cornerstone in breast cancer screening, credited with significant reductions in mortality rates. However, its limitations, including inter-reader variability, false positives, and false negatives, necessitate ongoing refinement and innovation. This research report comprehensively examines the current state of mammography, exploring advancements in imaging modalities, risk stratification approaches, and the integration of artificial intelligence (AI). We delve into the trade-offs between sensitivity and specificity across different screening strategies, discuss the impact of breast density, and analyze the psychosocial implications of screening programs. Furthermore, we assess the challenges associated with equitable access to mammography, the complexities of personalized screening protocols, and the evolving regulatory landscape. This report synthesizes current research, identifies areas of ongoing debate, and proposes directions for future research aimed at optimizing breast cancer screening programs and ultimately improving patient outcomes.
Many thanks to our sponsor Esdebe who helped us prepare this research report.
1. Introduction
Breast cancer remains a significant global health challenge, representing the most frequently diagnosed cancer and a leading cause of cancer-related mortality among women [1]. Early detection through screening programs is widely recognized as a critical strategy for improving survival rates and reducing the morbidity associated with advanced disease. Mammography, utilizing low-dose X-rays to image the breast, has been the primary screening modality for decades and its use is directly linked to a significant decline in breast cancer mortality [2]. However, mammography is not without its limitations. These include a relatively high rate of false-positive results, leading to unnecessary biopsies and patient anxiety, and a non-negligible false-negative rate, particularly in women with dense breast tissue, potentially delaying diagnosis and treatment [3]. Additionally, the interpretation of mammograms is subjective, leading to inter-reader variability and potentially affecting the accuracy of diagnosis.
Given these challenges, there is ongoing research and development aimed at enhancing the effectiveness and efficiency of mammography. This includes advancements in imaging technology, such as digital breast tomosynthesis (DBT), also known as 3D mammography, and contrast-enhanced mammography (CEM), as well as the integration of risk assessment models and personalized screening strategies. Furthermore, the application of artificial intelligence (AI) and machine learning (ML) techniques holds considerable promise for improving the accuracy and efficiency of mammogram interpretation, reducing the burden on radiologists, and ultimately leading to better patient outcomes.
This report provides a comprehensive overview of the current state of mammography, examining the latest advancements, addressing the persistent challenges, and exploring the future directions of breast cancer screening. It will delve into the technical aspects of various imaging modalities, analyze the impact of risk factors and breast density, and assess the role of AI in transforming mammography practice. The report will also address the ethical and societal implications of screening programs, including issues related to access, equity, and the psychosocial impact of screening results.
Many thanks to our sponsor Esdebe who helped us prepare this research report.
2. Mammography Techniques: Evolution and Current Status
2.1. Standard 2D Mammography
Conventional 2D mammography remains the most widely used screening modality. It involves acquiring two X-ray images of each breast, typically in the craniocaudal (CC) and mediolateral oblique (MLO) views. These images are then reviewed by radiologists to identify potential abnormalities, such as masses, microcalcifications, and architectural distortions. While 2D mammography has proven effective in detecting breast cancer, it suffers from limitations due to the superimposition of breast tissue, which can obscure underlying lesions or mimic the appearance of cancer [4]. This superimposition is a major contributor to both false-positive and false-negative results.
2.2. Digital Breast Tomosynthesis (DBT)
Digital breast tomosynthesis (DBT), also known as 3D mammography, addresses the limitations of 2D mammography by acquiring multiple low-dose X-ray images of the breast from different angles. These images are then reconstructed to create a three-dimensional representation of the breast tissue. DBT reduces the problem of tissue overlap, allowing for a more accurate visualization of breast structures and improving the detection of subtle abnormalities [5]. Studies have consistently demonstrated that DBT, compared to 2D mammography, leads to a higher cancer detection rate and a lower false-positive rate, particularly in women with dense breast tissue [6]. DBT is now widely accepted as a superior screening modality, although it comes with the caveat of slightly increased radiation exposure (although this is still within acceptable limits) and a slightly longer reading time for radiologists.
2.3. Contrast-Enhanced Mammography (CEM)
Contrast-enhanced mammography (CEM) involves injecting an intravenous contrast agent before acquiring mammographic images. The contrast agent highlights areas of increased blood flow, which is characteristic of malignant tumors. CEM can improve the detection of breast cancer, particularly in women with dense breast tissue or those at high risk of developing the disease [7]. CEM is generally used as a problem-solving tool, for example to assess equivocal findings on standard mammography or ultrasound, rather than as a primary screening modality. CEM involves intravenous contrast agent administration, which carries the risk of allergic reactions and is contraindicated in patients with severe renal impairment.
2.4. Other Emerging Techniques
Beyond these established techniques, research continues on other promising imaging modalities. Molecular breast imaging (MBI) uses radioactive tracers to detect metabolically active cancer cells, offering improved sensitivity in dense breasts but with higher radiation exposure. Breast MRI, while highly sensitive, is costly and often reserved for high-risk women or problem-solving. Photoacoustic imaging is another area of active research, offering potential for high-resolution, non-ionizing imaging based on the absorption of laser light by hemoglobin.
Many thanks to our sponsor Esdebe who helped us prepare this research report.
3. Breast Density and its Impact on Screening
Breast density refers to the amount of fibroglandular tissue compared to fatty tissue in the breast. Women with dense breasts have a higher proportion of fibroglandular tissue, which appears white on mammograms, similar to cancerous lesions. This makes it more difficult to detect cancer in dense breasts, leading to a higher false-negative rate. Furthermore, dense breast tissue is an independent risk factor for breast cancer [8].
Several states have enacted laws requiring that women be notified if they have dense breasts after a mammogram. This notification typically includes information about the impact of breast density on screening and the availability of supplemental screening modalities, such as ultrasound or MRI [9]. Supplemental screening is particularly valuable in women with heterogeneously or extremely dense breast tissue. Breast ultrasound is a common supplemental screening tool due to its ability to visualize masses that may be obscured on mammography. However, ultrasound also has a higher false-positive rate than mammography, leading to more biopsies.
The optimal screening strategy for women with dense breasts remains a topic of ongoing research. DBT has been shown to improve cancer detection rates in dense breasts compared to 2D mammography. The combination of DBT and supplemental screening may further enhance detection, but this approach needs to be balanced against the increased cost and potential for false-positive results.
Many thanks to our sponsor Esdebe who helped us prepare this research report.
4. Risk Stratification and Personalized Screening
Traditional mammography screening guidelines often recommend annual or biennial screening for all women over a certain age, regardless of their individual risk factors. However, this one-size-fits-all approach may not be optimal, as women have varying risks of developing breast cancer based on factors such as age, family history, genetics, and lifestyle.
Risk stratification involves assessing an individual’s risk of developing breast cancer based on these risk factors. Several risk assessment models are available, including the Gail model, the Claus model, and the Tyrer-Cuzick model [10]. These models use different combinations of risk factors to estimate a woman’s 5-year or lifetime risk of breast cancer. Individuals identified as being at high risk, such as those with a strong family history of breast cancer or carrying BRCA1/2 mutations, may benefit from more intensive screening, such as annual mammography starting at a younger age, or the addition of breast MRI.
Personalized screening involves tailoring the screening strategy to an individual’s risk profile. This may involve adjusting the frequency and type of screening tests based on their estimated risk. Personalized screening has the potential to improve the effectiveness and efficiency of breast cancer screening by focusing resources on those at highest risk while avoiding unnecessary screening in low-risk individuals [11]. However, the implementation of personalized screening requires accurate risk assessment tools, robust data infrastructure, and effective communication between healthcare providers and patients.
Many thanks to our sponsor Esdebe who helped us prepare this research report.
5. Artificial Intelligence in Mammography: Enhancing Accuracy and Efficiency
The application of artificial intelligence (AI) and machine learning (ML) techniques to mammography holds immense potential for improving the accuracy, efficiency, and accessibility of breast cancer screening. AI algorithms can be trained to detect subtle abnormalities on mammograms that may be missed by human readers, reducing the rate of false negatives. AI can also help to reduce the rate of false positives by improving the specificity of mammogram interpretation. Furthermore, AI can automate certain tasks, such as image processing and report generation, freeing up radiologists to focus on more complex cases [12].
5.1. Deep Learning and Convolutional Neural Networks (CNNs)
Deep learning, a subset of machine learning, has emerged as a particularly powerful tool for image analysis. Convolutional neural networks (CNNs) are a type of deep learning architecture that is well-suited for processing images. CNNs can be trained to recognize patterns in mammograms that are indicative of breast cancer, such as masses, microcalcifications, and architectural distortions. Several studies have shown that AI algorithms based on CNNs can achieve performance comparable to or even surpassing that of human radiologists in detecting breast cancer on mammograms [13].
5.2. Clinical Implementation and Challenges
Despite the promising results of AI research, there are several challenges to overcome before AI can be widely implemented in clinical practice. One challenge is the need for large, high-quality datasets to train AI algorithms. These datasets must be representative of the diverse patient populations that are screened for breast cancer. Another challenge is the need for rigorous validation studies to ensure that AI algorithms are safe and effective in real-world clinical settings. Regulatory hurdles, such as obtaining FDA approval, also need to be addressed. Furthermore, there are concerns about the potential for AI to exacerbate existing biases in healthcare, such as disparities in access to screening and treatment [14].
5.3. Future Directions
The future of AI in mammography is bright. As AI algorithms become more sophisticated and robust, they are likely to play an increasingly important role in breast cancer screening. AI can be used to improve the accuracy of mammogram interpretation, reduce the workload on radiologists, and personalize screening strategies. AI can also be used to develop new biomarkers for breast cancer risk assessment and early detection. Ultimately, the goal of AI in mammography is to improve patient outcomes and reduce the burden of breast cancer.
Many thanks to our sponsor Esdebe who helped us prepare this research report.
6. Psychosocial Impact of Mammography Screening
While mammography screening is an effective tool for early detection of breast cancer, it can also have a significant psychosocial impact on women. False-positive results can lead to anxiety, distress, and unnecessary biopsies [15]. False-negative results can provide a false sense of security and delay diagnosis, potentially leading to more advanced disease at the time of detection. Furthermore, the screening process itself can be stressful, particularly for women with a family history of breast cancer or those who have had previous breast abnormalities.
Effective communication between healthcare providers and patients is crucial for mitigating the psychosocial impact of mammography screening. Women need to be informed about the benefits and risks of screening, as well as the potential for false-positive and false-negative results. They should also be given the opportunity to ask questions and express their concerns. Providing clear and concise information about screening results, along with emotional support, can help to reduce anxiety and distress [16].
Many thanks to our sponsor Esdebe who helped us prepare this research report.
7. Access and Equity in Mammography Screening
Access to mammography screening is not uniform across all populations. Women in underserved communities, such as those who are low-income, uninsured, or live in rural areas, are less likely to receive regular mammograms [17]. This disparity in access can lead to delayed diagnosis and poorer outcomes for these women. Several factors contribute to these disparities, including lack of transportation, language barriers, cultural beliefs, and limited availability of screening facilities.
Efforts to improve access to mammography screening should focus on addressing these barriers. This may involve providing mobile mammography units in underserved communities, offering financial assistance for screening, translating educational materials into multiple languages, and training community health workers to promote breast cancer screening [18]. Furthermore, it is important to ensure that screening facilities are culturally sensitive and welcoming to all women.
Many thanks to our sponsor Esdebe who helped us prepare this research report.
8. Regulatory Landscape and Quality Assurance
Mammography screening is subject to strict regulatory oversight to ensure the quality and safety of the process. In the United States, the Mammography Quality Standards Act (MQSA) establishes minimum standards for mammography facilities, including requirements for equipment, personnel, and quality control [19]. The MQSA also requires that mammography facilities be accredited by an approved accreditation body and undergo regular inspections. These regulations help to ensure that mammography screening is performed safely and effectively.
Continuous quality improvement is essential for maintaining high standards in mammography screening. This involves monitoring key performance indicators, such as cancer detection rates, false-positive rates, and interval cancer rates, and implementing strategies to improve performance. Regular audits of mammography practice can help to identify areas for improvement and ensure that facilities are adhering to best practices [20].
Many thanks to our sponsor Esdebe who helped us prepare this research report.
9. Conclusion
Mammography remains a vital tool in the fight against breast cancer. Despite its limitations, it has been instrumental in reducing breast cancer mortality rates. Ongoing advancements in imaging technology, risk stratification, and artificial intelligence hold the promise of further improving the effectiveness and efficiency of mammography screening. Addressing the challenges related to breast density, psychosocial impact, access, and equity is crucial for ensuring that all women benefit from the life-saving potential of mammography. Future research should focus on optimizing personalized screening strategies, validating AI algorithms in diverse populations, and developing new biomarkers for early detection. By embracing innovation and addressing the persistent challenges, we can continue to improve breast cancer screening programs and ultimately reduce the burden of this disease.
Many thanks to our sponsor Esdebe who helped us prepare this research report.
References
[1] Sung H, Ferlay J, Siegel RL, et al. Global Cancer Statistics 2020: GLOBOCAN Estimates of Incidence and Mortality Worldwide for 36 Cancers in 185 Countries. CA Cancer J Clin. 2021;71(3):209-249.
[2] Tabár L, Vitak B, Chen THH, et al. Swedish Two-County Trial: Impact of Mammographic Screening on Breast Cancer Mortality during 3 Decades. Radiology. 2011;260(3):658-665.
[3] Boyd NF, Guo H, Martin LJ, et al. Mammographic Density and the Risk and Detection of Breast Cancer. N Engl J Med. 2007;356(3):227-236.
[4] Pisano ED, Gatsonis C, Hendrick E, et al. Diagnostic Performance of Digital Versus Film Mammography for Breast-Cancer Screening. N Engl J Med. 2005;353(17):1773-1783.
[5] Friedewald SM, Rafferty EA, Rose AM, et al. Breast Cancer Screening Using Tomosynthesis in Combination With Digital Mammography. JAMA. 2014;311(24):2499-2507.
[6] Ciatto S, Houssami N, Bernardi D, et al. Integration of 3D digital mammography with tomosynthesis for population breast-cancer screening (STORM): a prospective comparison study. Lancet Oncol. 2013;14(7):583-589.
[7] Fallenberg EM, Dromain C, Diekmann F, et al. Contrast-enhanced mammography: current applications. Eur Radiol. 2017;27(1):158-166.
[8] McCormack VA, dos Santos Silva I. Breast density and parenchymal patterns as markers of breast cancer risk: a meta-analysis. Cancer Epidemiol Biomarkers Prev. 2006;15(6):1159-1169.
[9] Hooley RJ, Scoutt LM, Philpotts LE. Breast density notification laws: what should women know?. AJR Am J Roentgenol. 2014;203(2):438-441.
[10] Gail MH, Brinton LA, Byar DP, et al. Projecting individualized probabilities of developing breast cancer for white females who are being screened annually. J Natl Cancer Inst. 1989;81(24):1879-1886.
[11] Brentnall AR, Cuzick J. Personalizing breast cancer screening based on risk. Breast Cancer Res. 2010;12(3):207.
[12] McKinney SM, Sieniek M, Godbole V, et al. International evaluation of an AI system for breast cancer screening. Nature. 2020;577(7788):86-94.
[13] Esteva A, Kuprel B, Novoa RA, et al. Dermatologist-level classification of skin cancer with deep neural networks. Nature. 2017;542(7639):115-118.
[14] Obermeyer Z, Powers B, Vogeli C, Mullainathan S. Dissecting racial bias in an algorithm used to manage the health of populations. Science. 2019;366(6464):447-453.
[15] Brodersen J, Siersma VD. Long-term psychosocial consequences of false-positive screening mammography. Ann Fam Med. 2013;11(6):539-546.
[16] Duric N, Littrup P, Li L, et al. Development of breast cancer screening protocol using ultrasound tomography. Med Phys. 2008;35(11):4752-4766.
[17] DeSantis CE, Ma J, Gaudet MM, et al. Breast cancer statistics, 2019. CA Cancer J Clin. 2019;69(6):438-451.
[18] Bastani R, Maxwell AE, Das IP, et al. Tailoring breast cancer education for Vietnamese American women. J Health Commun. 2002;7(4):319-339.
[19] U.S. Food and Drug Administration. Mammography Quality Standards Act (MQSA). Accessed March 8, 2024. https://www.fda.gov/radiation-emitting-products/mammography-quality-standards-act-mqsa/
[20] Smith RA, Manassaram-Baptiste D, Brooks D, et al. Breast cancer screening (United States). CA Cancer J Clin. 2015;65(1):30-54.
Given the increasing sophistication of AI in mammography, how might these technologies be leveraged to address disparities in access to screening for underserved communities?
That’s a crucial point! AI could revolutionize access. Imagine AI-powered mobile mammography units in rural areas, offering quicker scans and immediate analysis. AI can also aid in teleradiology, enabling remote experts to review images, bridging the gap in underserved areas where specialists are not common. Let’s explore funding models for these solutions!
Editor: MedTechNews.Uk
Thank you to our Sponsor Esdebe
Given the report’s focus on improving screening programs, what specific methodologies or frameworks are most effective in evaluating the overall cost-benefit ratio of implementing AI-driven mammography enhancements across diverse healthcare settings?