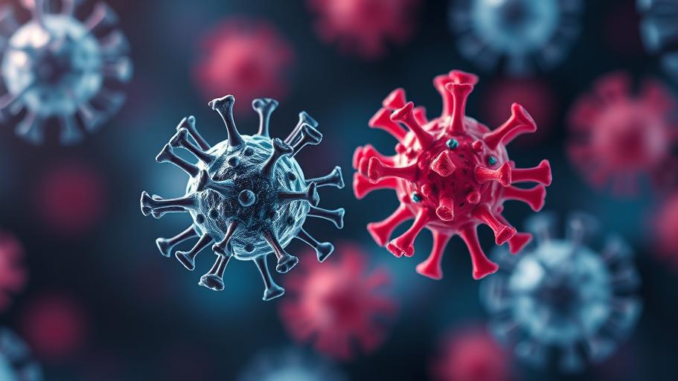
Abstract
Neoantigens, arising from tumor-specific mutations, have emerged as promising targets for personalized cancer immunotherapies. These unique antigens, absent in healthy cells, offer the potential to elicit highly specific T-cell responses against cancer, minimizing off-target toxicity. This review provides a comprehensive overview of the neoantigen landscape, encompassing their origin, types, and the intricate processes involved in their identification and validation. We delve into the immunogenicity of neoantigens, exploring the factors influencing their ability to stimulate robust T-cell responses. Furthermore, we critically examine the challenges associated with neoantigen-based immunotherapies, including prediction accuracy, tumor heterogeneity, and mechanisms of immune resistance. Finally, we survey the latest advancements in neoantigen prediction algorithms, high-throughput validation techniques, and clinical trial data highlighting the efficacy and limitations of neoantigen-based vaccines and adoptive T-cell therapies. We also discuss future directions and potential strategies to overcome current hurdles in the field, paving the way for more effective and personalized cancer treatments.
Many thanks to our sponsor Esdebe who helped us prepare this research report.
1. Introduction
The advent of next-generation sequencing technologies has revolutionized our understanding of cancer biology, revealing the extensive genetic heterogeneity within and between tumors. A key finding from these studies is the pervasive presence of somatic mutations, which give rise to novel peptides, termed neoantigens. Unlike self-antigens, which are shared between cancer and normal cells and are often subject to immune tolerance, neoantigens are tumor-specific and can be recognized by the immune system as foreign. This unique characteristic makes them ideal targets for cancer immunotherapies, offering the potential for highly specific and effective antitumor responses. The concept of personalized cancer vaccines, tailored to an individual’s tumor-specific neoantigen landscape, has gained significant traction in recent years, fueled by promising preclinical and clinical data. However, the field faces numerous challenges, including the accurate identification of immunogenic neoantigens, the development of robust delivery platforms, and the circumvention of immune resistance mechanisms. This review aims to provide a comprehensive overview of the neoantigen landscape, highlighting recent advances and challenges in the development of neoantigen-based immunotherapies.
Many thanks to our sponsor Esdebe who helped us prepare this research report.
2. The Origin and Types of Neoantigens
Neoantigens arise from a variety of genetic alterations within tumor cells, primarily somatic mutations. These mutations can occur in coding or non-coding regions of the genome, leading to the generation of novel peptides with the potential to be presented on major histocompatibility complex (MHC) molecules. Understanding the different types of mutations and their impact on neoantigen presentation is crucial for effective neoantigen-based immunotherapy.
2.1 Mutation-Derived Neoantigens
These represent the most commonly studied type of neoantigen. Single nucleotide variants (SNVs), insertions, and deletions (indels) within coding regions can lead to amino acid substitutions, frameshift mutations, or premature stop codons, resulting in the generation of novel peptides. Missense mutations, which result in a single amino acid change, are the most frequent source of neoantigens. Frameshift mutations, caused by indels, often lead to the generation of entirely novel peptide sequences with potentially high immunogenicity due to their significant deviation from self. In addition to SNVs and indels, structural variants such as gene fusions can also generate neoantigens, particularly in certain cancer types.
2.2 RNA Splicing-Derived Neoantigens
Aberrant RNA splicing, a common feature of cancer cells, can generate novel transcripts that encode unique peptides. Splicing mutations can lead to exon skipping, intron retention, or the use of alternative splice sites, resulting in the creation of novel exon-exon junctions. These junctional peptides, if presented on MHC molecules, can serve as neoantigens. The identification of splicing-derived neoantigens presents a unique challenge, as it requires the integration of RNA sequencing data with genomic information.
2.3 Post-translational Modification-Derived Neoantigens
While less extensively studied, post-translational modifications (PTMs) can also contribute to neoantigen diversity. PTMs such as glycosylation, phosphorylation, and ubiquitination can alter the structure and immunogenicity of peptides. Aberrant expression or activity of enzymes involved in PTMs can lead to the generation of tumor-specific PTM patterns, creating novel epitopes that can be recognized by the immune system. Further research is needed to fully elucidate the role of PTMs in neoantigen generation and their potential as therapeutic targets.
2.4 Cancer/Testis Antigens
Although not strictly neoantigens derived from somatic mutations, Cancer/Testis Antigens (CTAs) are a class of proteins normally expressed only in germ cells of the testes but are aberrantly reactivated in various cancers. Due to their restricted expression in normal tissues, CTAs are often considered tumor-associated antigens and can elicit spontaneous immune responses in cancer patients. While not truly neoantigens, their tumor-restricted expression makes them attractive targets for immunotherapy, often used in combination with true neoantigen targets.
Many thanks to our sponsor Esdebe who helped us prepare this research report.
3. Neoantigen Identification and Selection
The identification and selection of immunogenic neoantigens is a complex and multi-step process that relies on the integration of genomic, transcriptomic, and proteomic data. The accuracy and efficiency of this process are critical for the success of neoantigen-based immunotherapies.
3.1 Neoantigen Prediction Algorithms
Neoantigen prediction typically begins with the identification of somatic mutations through whole-exome sequencing (WES) or whole-genome sequencing (WGS) of tumor and matched normal samples. RNA sequencing (RNA-Seq) data is then used to confirm the expression of mutated genes. Next, computational algorithms predict the binding affinity of mutant peptides to MHC class I and class II molecules. These algorithms are based on machine learning models trained on large datasets of peptide-MHC binding data. Popular algorithms include NetMHCpan, MHCflurry, and MixMHCpred. However, it’s important to note that binding affinity is not the sole determinant of immunogenicity. Other factors, such as peptide processing, MHC stability, and T-cell receptor (TCR) avidity, also play important roles.
3.2 Prioritization Strategies
Given the large number of potential neoantigens identified in a typical tumor, prioritization strategies are essential to select the most promising candidates for vaccine development. Several factors are considered during prioritization, including:
- MHC Binding Affinity: Peptides with high predicted binding affinity to MHC molecules are generally prioritized.
- Expression Level: Neoantigens derived from highly expressed genes are more likely to be presented on the cell surface and recognized by T cells.
- Mutation Type: Frameshift mutations and mutations in highly conserved regions are often prioritized due to their greater potential to generate strong immune responses.
- Clonality: Neoantigens present in a high proportion of tumor cells (clonal neoantigens) are more likely to be effective targets, as they are less susceptible to immune escape.
- Location: Neoantigens derived from coding regions are favoured, though non-coding neoantigens derived from mutations in regulatory elements are increasingly recognised as potential targets.
3.3 Validation Techniques
While computational prediction is a crucial first step, experimental validation is necessary to confirm the immunogenicity of selected neoantigens. Several validation techniques are employed, including:
- Peptide-MHC Binding Assays: These assays directly measure the binding affinity of predicted neoantigens to purified MHC molecules.
- T Cell Activation Assays: These assays assess the ability of neoantigen-pulsed antigen-presenting cells (APCs) to stimulate T-cell activation and proliferation.
- ELISpot Assays: ELISpot assays measure the secretion of cytokines, such as IFN-γ, by T cells in response to neoantigen stimulation.
- Tetramer Staining: MHC-multimer (tetramer) staining allows for the direct identification and enumeration of T cells specific for a particular neoantigen.
- Mass Spectrometry: Mass spectrometry-based approaches can be used to directly identify neoantigens presented on the surface of tumor cells.
Mass spectrometry, while technically demanding and often requiring significant enrichment steps, offers the most direct method for validating neoantigen presentation. Newer approaches combine immunoprecipitation of MHC molecules with high-resolution mass spectrometry to identify naturally processed and presented neoantigens, bypassing the need for peptide synthesis and in vitro loading.
Many thanks to our sponsor Esdebe who helped us prepare this research report.
4. Immunogenicity of Neoantigens
The immunogenicity of neoantigens is a critical determinant of their efficacy as targets for cancer immunotherapy. Several factors influence the ability of neoantigens to elicit robust T-cell responses. Understanding these factors is essential for optimizing neoantigen-based immunotherapeutic strategies.
4.1 Factors Influencing Immunogenicity
- MHC Binding Affinity: As mentioned earlier, strong MHC binding affinity is generally associated with higher immunogenicity. However, optimal binding affinity is necessary. Peptides with extremely high binding affinity may not dissociate from MHC molecules efficiently, hindering T-cell receptor engagement.
- Peptide Stability: The stability of the peptide-MHC complex is important for sustained T-cell activation. Peptides that rapidly dissociate from MHC molecules may not provide sufficient signaling to trigger T-cell responses.
- TCR Avidity: The avidity of the TCR for the peptide-MHC complex is a critical determinant of T-cell activation. High-avidity TCRs are more likely to trigger strong T-cell responses.
- T-Cell Repertoire: The presence of a pre-existing T-cell repertoire capable of recognizing the neoantigen is essential for effective immune responses. Individuals lacking T cells specific for a particular neoantigen will not respond to neoantigen-based vaccination.
- Immune Context: The tumor microenvironment (TME) can significantly impact the immunogenicity of neoantigens. Immunosuppressive factors within the TME, such as regulatory T cells (Tregs) and myeloid-derived suppressor cells (MDSCs), can dampen T-cell responses.
- Co-stimulatory Signals: Adequate co-stimulation through molecules like CD28 on T cells and B7 on antigen-presenting cells is essential for optimal T-cell activation and proliferation.
- Neoantigen Structural Similarity to Self-Antigens: Paradoxically, while the ‘foreignness’ of neoantigens is their key advantage, excessive dissimilarity to self-antigens can lead to reduced TCR cross-reactivity with self-peptides, potentially hindering T cell priming. A balance between novelty and some degree of similarity may be optimal for robust and sustained immune responses.
4.2 Mechanisms of T-Cell Activation
Neoantigen-specific T cells are activated through the interaction of their TCR with the peptide-MHC complex on the surface of APCs. This interaction triggers a signaling cascade within the T cell, leading to the activation of transcription factors and the expression of effector molecules, such as cytokines and cytotoxic proteins. Effective T-cell activation requires not only TCR engagement but also co-stimulatory signals, which provide additional signals necessary for T-cell survival, proliferation, and differentiation. In the context of cancer immunotherapy, T-cell activation can occur in the tumor microenvironment, in draining lymph nodes, or in the circulation.
4.3 Immune Tolerance
While neoantigens are generally considered foreign, immune tolerance mechanisms can still limit their immunogenicity. Central tolerance, which occurs in the thymus, can eliminate T cells with high avidity for self-antigens. Peripheral tolerance mechanisms, such as anergy and T-cell exhaustion, can also suppress T-cell responses to neoantigens. The balance between immune activation and tolerance determines the overall immunogenicity of a particular neoantigen.
Many thanks to our sponsor Esdebe who helped us prepare this research report.
5. Challenges and Limitations of Neoantigen-Based Immunotherapy
Despite the immense promise of neoantigen-based immunotherapies, several challenges and limitations need to be addressed to improve their efficacy.
5.1 Prediction Accuracy
The accuracy of neoantigen prediction algorithms is a major challenge. While current algorithms can predict MHC binding affinity with reasonable accuracy, they often fail to accurately predict immunogenicity. Factors such as peptide processing, MHC stability, and TCR avidity are not fully accounted for in these algorithms. Furthermore, the limited availability of high-quality training data for machine learning models restricts the performance of these algorithms.
5.2 Tumor Heterogeneity
Tumor heterogeneity, both inter- and intra-tumoral, poses a significant challenge to neoantigen-based immunotherapies. Neoantigens present in a small fraction of tumor cells may not be effective targets, as the immune response may not be sufficient to eliminate the entire tumor. Furthermore, tumors can evolve and acquire new mutations over time, leading to the loss of targeted neoantigens and the emergence of resistant clones. Targeting clonal neoantigens, present in the majority of tumor cells, is a strategy to mitigate the impact of tumor heterogeneity, but even clonal neoantigens can be lost during tumor evolution.
5.3 Immune Resistance Mechanisms
Cancer cells can evade immune destruction through a variety of mechanisms, including downregulation of MHC expression, upregulation of inhibitory immune checkpoints (e.g., PD-1, CTLA-4), and secretion of immunosuppressive factors (e.g., TGF-β, IL-10). These immune resistance mechanisms can limit the efficacy of neoantigen-based immunotherapies. Combination therapies that target both neoantigens and immune checkpoints may be necessary to overcome these resistance mechanisms.
5.4 Delivery and Formulation
The effective delivery of neoantigens to APCs is crucial for generating robust T-cell responses. Various delivery platforms are being explored, including synthetic long peptides, mRNA vaccines, and viral vectors. Each platform has its own advantages and disadvantages in terms of immunogenicity, safety, and cost. Formulating neoantigens with appropriate adjuvants is also essential for enhancing immune responses. In the context of personalized vaccines, the logistical challenges of manufacturing patient-specific vaccines in a timely and cost-effective manner are also significant.
5.5 T Cell Exhaustion
Chronic exposure to antigen in the tumor microenvironment can lead to T cell exhaustion, characterized by the progressive loss of effector function and the upregulation of inhibitory receptors. Exhausted T cells may be unable to effectively eliminate tumor cells, even if they are specific for neoantigens. Strategies to reverse T cell exhaustion, such as blocking inhibitory immune checkpoints or providing co-stimulatory signals, may improve the efficacy of neoantigen-based immunotherapies.
Many thanks to our sponsor Esdebe who helped us prepare this research report.
6. Recent Advances and Clinical Trial Data
The field of neoantigen-based immunotherapy has witnessed significant progress in recent years, with advancements in neoantigen prediction, validation, and delivery. Several clinical trials have demonstrated the feasibility and potential efficacy of neoantigen-based vaccines and adoptive T-cell therapies.
6.1 Advances in Neoantigen Prediction and Validation Technologies
- Machine Learning Enhancements: Advances in machine learning algorithms, particularly deep learning, have improved the accuracy of MHC binding prediction and immunogenicity prediction. These algorithms are now able to incorporate more complex features, such as peptide processing and TCR-peptide-MHC interactions.
- High-Throughput Validation Platforms: High-throughput platforms for validating neoantigen immunogenicity have been developed, allowing for the rapid screening of large numbers of neoantigens. These platforms often involve the use of multiplexed assays, such as cytokine bead arrays and multi-parameter flow cytometry.
- Improved Mass Spectrometry Techniques: Advances in mass spectrometry techniques have enabled the more sensitive and accurate identification of neoantigens presented on the surface of tumor cells. These techniques are increasingly being used to validate neoantigen predictions and to monitor neoantigen presentation in response to immunotherapy.
- CRISPR-based functional screening: CRISPR-based technologies are increasingly employed for functional screening of neoantigen presentation and immunogenicity. These approaches allow researchers to systematically knockout genes involved in antigen processing and presentation pathways, enabling the identification of key factors required for effective neoantigen-specific T cell responses.
6.2 Clinical Trial Data
Several clinical trials have evaluated the safety and efficacy of neoantigen-based vaccines in patients with various types of cancer. These trials have generally shown that neoantigen-based vaccines are well-tolerated and can elicit neoantigen-specific T-cell responses. In some cases, these vaccines have been associated with clinical responses, including tumor regression and prolonged survival. However, the response rates have varied depending on the cancer type, the vaccine design, and the patient population.
Adoptive T-cell therapy (ACT) targeting neoantigens has also shown promise in clinical trials. In this approach, T cells are isolated from a patient’s blood, engineered to express TCRs specific for neoantigens, and then infused back into the patient. ACT targeting neoantigens has been associated with impressive clinical responses in some patients, particularly those with melanoma. However, ACT is a complex and expensive therapy, and its efficacy can be limited by factors such as T-cell trafficking and persistence.
Specific Clinical Trials:
Several notable clinical trials highlight the progress in neoantigen-based immunotherapy:
- GARDASIL-9 Success in HPV-related cancers: Although not strictly neoantigen-based, the success of GARDASIL-9 in preventing HPV-related cancers underscores the potential of prophylactic vaccination against tumor-associated antigens, paving the way for future neoantigen-based preventive strategies.
- Personalized mRNA Vaccines: Trials investigating personalized mRNA vaccines targeting neoantigens have demonstrated promising results in melanoma and other solid tumors. These vaccines have been shown to elicit robust neoantigen-specific T cell responses and, in some cases, lead to tumor regression or stabilization when combined with checkpoint inhibitors.
- Adoptive T-cell Therapy (ACT): Studies evaluating ACT targeting neoantigens have demonstrated impressive clinical responses in patients with heavily pre-treated solid tumors. However, the complexity and cost of ACT, along with challenges related to T cell trafficking and persistence, remain significant hurdles.
- Combination therapies: Clinical trials evaluating the combination of neoantigen-based vaccines with checkpoint inhibitors, such as anti-PD-1 or anti-CTLA-4 antibodies, have shown enhanced clinical efficacy compared to either therapy alone. These findings support the rationale for combining neoantigen-based immunotherapy with other modalities to overcome immune resistance mechanisms.
Many thanks to our sponsor Esdebe who helped us prepare this research report.
7. Future Directions
The field of neoantigen-based immunotherapy is rapidly evolving, with numerous opportunities for future research and development.
7.1 Improving Neoantigen Prediction
Future research should focus on improving the accuracy of neoantigen prediction algorithms. This will require the development of more sophisticated machine learning models that incorporate a wider range of factors influencing immunogenicity, such as peptide processing, MHC stability, and TCR avidity. Furthermore, the use of larger and more diverse training datasets will be essential for improving the performance of these algorithms. Incorporating information from the three-dimensional structure of the TCR-peptide-MHC complex could also significantly enhance prediction accuracy.
7.2 Targeting Intracellular Antigens
Strategies to target intracellular antigens, which are not naturally presented on MHC molecules, are also being explored. This can be achieved through the use of modified viruses or bacteria that deliver neoantigens directly into the cytoplasm of APCs, or through the use of bispecific antibodies that redirect T cells to tumor cells expressing intracellular neoantigens.
7.3 Overcoming Immune Resistance
Future research should focus on developing strategies to overcome immune resistance mechanisms. This may involve the use of combination therapies that target both neoantigens and immune checkpoints, or the development of novel immunomodulatory agents that enhance T-cell function and overcome immunosuppression within the tumor microenvironment. The use of oncolytic viruses to directly lyse tumor cells and release tumor antigens, in combination with neoantigen-based vaccines, is another promising strategy.
7.4 Personalized Immunotherapy Strategies
The development of personalized immunotherapy strategies, tailored to the individual characteristics of each patient’s tumor and immune system, is a major goal of the field. This will require the integration of multi-omics data, including genomic, transcriptomic, proteomic, and immunomic data, to identify the most promising neoantigens and to predict the likelihood of response to immunotherapy. Single-cell sequencing technologies are playing an increasingly important role in characterizing the tumor microenvironment and identifying potential targets for immunotherapy.
7.5 Prophylactic Neoantigen Vaccines
While currently focused on treating existing cancers, the concept of prophylactic neoantigen vaccines is gaining traction. This approach aims to prevent cancer development in high-risk individuals by vaccinating them against neoantigens that are likely to arise in specific cancer types. This approach presents significant challenges, including the identification of relevant neoantigens and the long-term monitoring of vaccine efficacy.
Many thanks to our sponsor Esdebe who helped us prepare this research report.
8. Conclusion
Neoantigens represent a promising class of targets for personalized cancer immunotherapies. The unique nature of these antigens, coupled with the ability to elicit highly specific T-cell responses, offers the potential for effective and safe cancer treatments. While significant progress has been made in the field, several challenges remain, including the accurate prediction of immunogenic neoantigens, the circumvention of immune resistance mechanisms, and the development of robust delivery platforms. Ongoing research efforts are focused on addressing these challenges and developing more effective neoantigen-based immunotherapies. As our understanding of the neoantigen landscape continues to evolve, we can expect to see further advancements in the field, ultimately leading to improved outcomes for cancer patients.
Many thanks to our sponsor Esdebe who helped us prepare this research report.
References
- Schumacher, T. N., & Schreiber, R. D. (2015). Neoantigens in cancer immunotherapy. Science, 348(6230), 69-74.
- Yadav, M., Jhunjhunwala, S., Phung, Q. T., Lupardus, P., & Bhatia, S. (2014). Predicting immunogenic tumour mutations by combining mass spectrometry and exome sequencing. Nature, 515(7528), 572-576.
- Ott, P. A., Hu, Z., Keskin, D. B., Shukla, S. A., Kim, P. S., Wang, J., … & Wu, C. J. (2017). An immunogenic personal neoantigen vaccine for patients with melanoma. Nature, 547(7662), 217-221.
- Sahin, U., Oehm, P., Derhovanessian, E., Jabulowsky, R. A., Vornlocher, H. P., Kranz, L. M., … & Tureci, O. (2017). Personalized RNA mutanome vaccines mobilize poly-specific therapeutic immunity against cancer. Nature, 547(7662), 222-226.
- Carreno, B. M., Magrini, V., Becker-Hapak, M., Kaabinejadian, S., Hundal, J., Petti, A. A., … & Mardis, E. R. (2015). Cancer immunotherapy. A dendritic cell vaccine increases the breadth and diversity of melanoma neoantigen-specific T cells. Science, 348(6236), 803-808.
- Strønen, E., Toebes, M., Kelderman, S., van Buuren, M. M., Kessler, J. H., Rodenburg, C. M., … & Schumacher, T. N. (2016). Neoantigen sensitivity varies widely between individuals. Nature, 534(7607), 399-403.
- Łuksza, M., Riaz, N., Makarov, V., Balachandran, V. P., Hellmann, M. D., Solovyov, A., … & Merghoub, T. (2017). Neoantigen quality predicts immunoediting in response to checkpoint blockade. Nature, 550(7674), 128-132.
- Peng, M., Chen, O., Metselaar, R., & Storm, G. (2019). Neoantigen-based cancer vaccines: the Holy Grail of personalized immunotherapy. Vaccine, 37(9), 1152-1160.
- Duperret, E. K., Traugh, N., Amaria, R. N., Tanyi, J. L., Disis, M. L., & Ali, S. M. (2019). Neoantigen landscape and immune responses in solid tumors. Annals of Oncology, 30(8), 1223-1234.
- Ott, P. A., & Bhardwaj, N. (2021). Personal Neoantigen Vaccines-Ready for Prime Time?. Clinical Cancer Research, 27(19), 5253-5255.
- Hugo, W., Zaretsky, J. M., Sun, L., Song, C., Moreno, B. H., Hu-Lieskovan, S., … & Ribas, A. (2016). Genomic and transcriptomic characterization of primary and acquired resistance to anti-PD-1 therapy in melanoma. Cell, 165(1), 35-47.
- Miao, D., Margolis, C. A., Gao, W., Vosseller, J. T., Gadwa, J. E., Littlefield, K., … & Wagle, N. (2018). Genomic correlates of response to immune checkpoint blockade in microsatellite-stable solid tumors. Nature Genetics, 50(9), 1271-1281.
- Tran, E., Robbins, P. F., Lu, Y. C., Prickett, T. D., Gartner, J. J., Jia, L., … & Rosenberg, S. A. (2016). T-cell transfer therapy targeting mutant KRAS in cancer. New England Journal of Medicine, 375(23), 2255-2262.
- Rosenberg, S. A., & Restifo, N. P. (2015). Adoptive cell transfer as personalized immunotherapy for human cancer. Science, 348(6230), 62-68.
- Castle, J. C., Kreiter, S., Diekmann, J., Löwer, M., van de Roemer, N., de Graaf, J., … & Sahin, U. (2012). Exploiting the mutanome for tumor vaccination. Cancer Research, 72(5), 1081-1091.
Be the first to comment