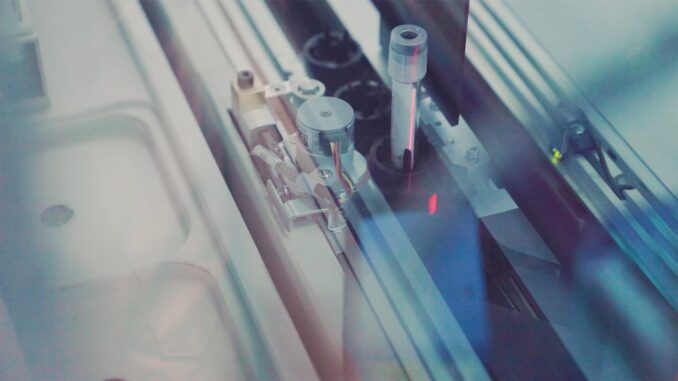
Abstract
Artificial Intelligence (AI) is rapidly transforming various sectors, and cardiology is no exception. This research report explores the multifaceted applications of AI in cardiology, extending beyond the well-documented area of atrial fibrillation (AFib) risk prediction. We delve into AI-driven diagnostics for a wide range of cardiovascular diseases, including heart failure, coronary artery disease, and valvular heart disease. Furthermore, we examine the potential of AI in assisting surgical procedures, optimizing treatment strategies, and enabling personalized medicine tailored to individual patient profiles. The report also addresses the ethical and practical considerations associated with the increasing adoption of AI in cardiology departments, including data privacy, algorithmic bias, and the evolving role of human clinicians. By synthesizing current research and expert perspectives, this report aims to provide a comprehensive overview of the current state and future trajectory of AI in cardiology, highlighting its potential to revolutionize cardiovascular care while acknowledging the challenges that must be addressed to ensure responsible and equitable implementation.
Many thanks to our sponsor Esdebe who helped us prepare this research report.
1. Introduction
Cardiovascular disease (CVD) remains a leading cause of morbidity and mortality worldwide, placing a significant burden on healthcare systems. The complexity of cardiac pathophysiology, coupled with the increasing volume of patient data, presents both challenges and opportunities for innovation. Artificial Intelligence (AI), with its ability to analyze vast datasets, identify patterns, and make predictions, has emerged as a powerful tool to address these challenges and improve cardiovascular care.
While early applications of AI in cardiology primarily focused on areas like electrocardiogram (ECG) interpretation and image analysis, the field has since expanded significantly. Modern AI techniques, including machine learning (ML) and deep learning (DL), are being utilized to develop sophisticated diagnostic tools, predict patient outcomes, optimize treatment strategies, and even assist in surgical procedures. This report aims to provide a comprehensive overview of the current landscape of AI in cardiology, exploring its diverse applications, discussing its limitations, and considering the ethical implications of its widespread adoption.
This report will extend beyond the already well-trodden ground of AFib risk prediction. We will examine the deployment of AI across a wider spectrum of cardiovascular conditions, encompassing areas such as heart failure management, coronary artery disease detection, and valvular heart disease diagnosis. Furthermore, the report will consider the transformative potential of AI in personalized medicine, where treatment decisions are tailored to the unique characteristics of individual patients.
Many thanks to our sponsor Esdebe who helped us prepare this research report.
2. AI-Driven Diagnostics in Cardiology
The diagnostic process in cardiology relies heavily on a combination of clinical assessment, imaging techniques, and biomarker analysis. AI has the potential to enhance the accuracy, efficiency, and accessibility of these diagnostic methods.
2.1. Electrocardiogram (ECG) Analysis
The ECG is a fundamental tool for diagnosing a variety of cardiac arrhythmias and ischemic conditions. AI algorithms have demonstrated remarkable accuracy in ECG interpretation, often surpassing the performance of human experts in identifying subtle abnormalities. DL models, trained on large datasets of ECG recordings, can detect AFib, atrial flutter, ventricular tachycardia, and ST-segment elevation myocardial infarction (STEMI) with high sensitivity and specificity [1]. Furthermore, AI can be used to continuously monitor ECG data and alert clinicians to potentially life-threatening events.
2.2. Cardiac Imaging
Cardiac imaging modalities, such as echocardiography, cardiac magnetic resonance imaging (CMR), and computed tomography angiography (CTA), provide valuable information about the structure and function of the heart. AI can be used to automate image segmentation, quantification, and interpretation, reducing the time and effort required for analysis while improving accuracy and reproducibility. For example, AI algorithms can automatically measure left ventricular ejection fraction (LVEF) from echocardiograms, detect coronary artery stenosis from CTA images, and identify myocardial infarction from CMR scans [2]. Furthermore, AI can be used to enhance image quality and reduce radiation exposure during CTA scans.
2.3. Biomarker Analysis
Cardiac biomarkers, such as troponin and B-type natriuretic peptide (BNP), play a crucial role in the diagnosis and management of heart disease. AI can be used to integrate biomarker data with other clinical information to improve risk stratification and predict patient outcomes. For instance, ML models can be trained to predict the risk of heart failure hospitalization based on BNP levels, ECG findings, and patient demographics [3]. Moreover, AI can identify novel biomarkers that may be predictive of cardiovascular events, leading to the development of new diagnostic tests.
2.4. Detection of Heart Failure
Heart failure (HF) is a complex clinical syndrome with diverse etiologies and presentations. Early and accurate diagnosis of HF is crucial for effective management and improved patient outcomes. AI-powered tools can analyze various data sources, including ECGs, echocardiograms, clinical notes, and laboratory results, to identify patients at risk for HF or to confirm a diagnosis of HF with greater accuracy. AI algorithms can also distinguish between different types of HF, such as heart failure with reduced ejection fraction (HFrEF) and heart failure with preserved ejection fraction (HFpEF), which require different treatment strategies [4].
Many thanks to our sponsor Esdebe who helped us prepare this research report.
3. AI-Assisted Surgical Procedures
Cardiovascular surgery is a highly specialized field that requires precision, skill, and experience. AI has the potential to enhance surgical performance, reduce complications, and improve patient outcomes.
3.1. Robotic Surgery
Robotic surgical systems, such as the da Vinci Surgical System, provide surgeons with enhanced dexterity, visualization, and control. AI can be integrated into robotic surgical platforms to provide real-time guidance, automate certain surgical tasks, and improve the accuracy of surgical maneuvers. For example, AI algorithms can be used to identify anatomical structures, plan surgical incisions, and assist with suturing [5].
3.2. Predictive Analytics for Surgical Risk
Predicting the risk of complications after cardiac surgery is essential for patient selection and informed consent. AI can be used to develop predictive models that incorporate a wide range of patient characteristics, surgical factors, and perioperative data to estimate the likelihood of adverse events, such as bleeding, infection, and stroke [6]. These models can help surgeons identify high-risk patients and tailor their surgical approach accordingly.
3.3. Intraoperative Guidance
During cardiac surgery, real-time imaging and monitoring are crucial for guiding surgical decisions. AI can be used to analyze intraoperative imaging data, such as transesophageal echocardiography (TEE) images, to provide surgeons with real-time feedback on the adequacy of valve repair, the presence of residual lesions, and the overall hemodynamic status of the patient [7]. This information can help surgeons optimize their surgical technique and ensure the best possible outcome for the patient.
Many thanks to our sponsor Esdebe who helped us prepare this research report.
4. AI in Personalized Medicine for Cardiovascular Health
Personalized medicine aims to tailor treatment strategies to the unique characteristics of individual patients. AI can play a pivotal role in this area by analyzing vast amounts of patient data to identify patterns, predict treatment responses, and optimize therapeutic interventions.
4.1. Risk Stratification
Accurate risk stratification is essential for guiding treatment decisions in cardiovascular disease. AI can be used to develop sophisticated risk prediction models that incorporate genetic data, clinical information, imaging findings, and biomarker data to identify patients who are at high risk of cardiovascular events [8]. These models can help clinicians prioritize patients for aggressive interventions, such as coronary artery bypass grafting (CABG) or percutaneous coronary intervention (PCI).
4.2. Treatment Optimization
AI can be used to optimize drug selection, dosage, and timing for individual patients. For example, ML algorithms can be trained to predict the response to different antiplatelet therapies based on patient characteristics and genetic factors [9]. This information can help clinicians choose the most effective antiplatelet regimen for each patient, minimizing the risk of bleeding and thrombotic events. Furthermore, AI can be used to personalize the management of heart failure by identifying patients who are most likely to benefit from specific medications, such as angiotensin-converting enzyme inhibitors (ACEIs) or beta-blockers.
4.3. Lifestyle Interventions
Lifestyle modifications, such as diet and exercise, are crucial for preventing and managing cardiovascular disease. AI can be used to personalize lifestyle recommendations based on individual patient preferences, genetic predispositions, and environmental factors. For example, AI algorithms can analyze wearable sensor data to track physical activity levels and provide personalized feedback and encouragement to promote adherence to exercise programs [10].
4.4. Pharmacogenomics
AI plays a crucial role in analyzing the vast and complex data generated by pharmacogenomic studies. By integrating genetic information with clinical data, AI algorithms can identify genetic variants that influence drug response and toxicity. This allows clinicians to select the most appropriate medications and dosages for individual patients, maximizing therapeutic efficacy and minimizing adverse effects. In cardiology, pharmacogenomics is particularly relevant for drugs such as clopidogrel, warfarin, and statins [11]. AI’s ability to process and interpret complex genomic data enables a more precise and personalized approach to drug therapy.
Many thanks to our sponsor Esdebe who helped us prepare this research report.
5. Ethical and Practical Implications
The increasing adoption of AI in cardiology raises several ethical and practical considerations that must be addressed to ensure responsible and equitable implementation.
5.1. Data Privacy and Security
AI algorithms require access to large datasets of patient information, raising concerns about data privacy and security. It is essential to implement robust security measures to protect patient data from unauthorized access and misuse. Furthermore, patients must be informed about how their data will be used and given the opportunity to opt out of data sharing.
5.2. Algorithmic Bias
AI algorithms can perpetuate and amplify existing biases in the data on which they are trained. If the training data is not representative of the population as a whole, the resulting algorithms may perform poorly on certain subgroups of patients. It is essential to carefully evaluate AI algorithms for bias and to mitigate any identified biases through data augmentation, algorithm modification, or fairness-aware training techniques.
5.3. Transparency and Explainability
Many AI algorithms, particularly DL models, are “black boxes” that are difficult to interpret. This lack of transparency can make it challenging to understand why an algorithm made a particular prediction and can erode trust in the technology. It is important to develop AI algorithms that are more transparent and explainable, allowing clinicians to understand the reasoning behind their decisions. Techniques such as explainable AI (XAI) can be used to provide insights into the inner workings of AI models.
5.4. The Evolving Role of Human Clinicians
As AI becomes more prevalent in cardiology, the role of human clinicians will inevitably evolve. AI will likely automate many of the routine tasks that are currently performed by physicians, allowing clinicians to focus on more complex cases and on providing personalized care to patients. However, it is essential to ensure that clinicians retain their critical thinking skills and their ability to make informed decisions in the face of uncertainty. Furthermore, it is important to provide clinicians with adequate training and support to use AI tools effectively.
5.5. Regulation and Governance
The regulation and governance of AI in cardiology is a complex and evolving area. It is essential to establish clear guidelines and standards for the development, validation, and deployment of AI algorithms to ensure that they are safe, effective, and equitable. Regulatory bodies, such as the Food and Drug Administration (FDA), play a crucial role in overseeing the use of AI in healthcare. Furthermore, professional societies and ethical review boards can provide guidance on the ethical and responsible use of AI in cardiology.
Many thanks to our sponsor Esdebe who helped us prepare this research report.
6. Challenges and Future Directions
Despite the significant progress made in AI for cardiology, several challenges remain. These include:
- Data Availability and Quality: The performance of AI algorithms depends heavily on the availability of high-quality data. However, access to large, well-curated datasets can be limited, particularly for rare cardiovascular conditions.
- Generalizability: AI algorithms that are trained on data from one institution or population may not generalize well to other settings. It is essential to validate AI algorithms on diverse datasets to ensure their generalizability.
- Integration with Clinical Workflows: Integrating AI tools into existing clinical workflows can be challenging. It is important to design AI systems that are user-friendly and seamlessly integrate with electronic health records (EHRs) and other clinical information systems.
- Trust and Acceptance: Building trust in AI among clinicians and patients is essential for its widespread adoption. It is important to communicate the benefits and limitations of AI clearly and to address any concerns about safety, privacy, and bias.
Future directions for AI in cardiology include:
- Development of Explainable AI (XAI) techniques: Creating AI models that provide insights into their decision-making processes will enhance trust and facilitate clinical adoption.
- Integration of multimodal data: Combining data from various sources, such as genomics, proteomics, imaging, and clinical data, will lead to more comprehensive and accurate diagnoses and predictions.
- AI-powered drug discovery: Utilizing AI to identify novel drug targets and accelerate the development of new therapies for cardiovascular disease.
- Real-time AI-driven decision support: Developing AI systems that can provide real-time guidance to clinicians during procedures and patient encounters.
- AI-enabled remote monitoring: Using AI to analyze data from wearable sensors and remote monitoring devices to detect early signs of cardiovascular problems and provide timely interventions.
Many thanks to our sponsor Esdebe who helped us prepare this research report.
7. Conclusion
Artificial Intelligence is poised to revolutionize cardiology, offering the potential to improve diagnosis, treatment, and personalized care for patients with cardiovascular disease. From AI-driven diagnostics to AI-assisted surgery and personalized medicine, the applications of AI in cardiology are vast and rapidly evolving. However, the successful adoption of AI requires careful consideration of ethical and practical implications, including data privacy, algorithmic bias, and the evolving role of human clinicians. By addressing these challenges and embracing a collaborative approach between clinicians, engineers, and policymakers, we can harness the power of AI to transform cardiovascular care and improve the lives of millions of people worldwide.
Many thanks to our sponsor Esdebe who helped us prepare this research report.
References
[1] Acharya, U. R., Fujita, H., Oh, S. L., Hagiwara, Y., Tan, J. H., & Adam, M. (2017). Automated detection of arrhythmias using different intervals of ECG signals with convolutional neural network. Information Sciences, 405, 81-90.
[2] Motwani, M., Dey, D., Berman, D. S., Germano, G., Achenbach, S., Al-Mallah, M. H., … & Slomka, P. J. (2018). Machine learning for cardiovascular risk assessment: recent advances and future directions. JACC: Cardiovascular Imaging, 11(11), 1617-1632.
[3] Mortazavi, B. J., Downing, N. S., Bucholz, E. M., Desai, M. M., Dhruva, S. S., Spatz, E. S., … & Krumholz, H. M. (2016). Analysis of machine learning in predicting mortality and hospital readmission among patients with heart failure. Circulation: Heart Failure, 9(9), e003363.
[4] Shahid, M., Rappon, T., & van den Heuvel, C. (2021). Predictive modelling for heart failure using machine learning: A systematic review. Heart failure reviews, 26(2), 359-380.
[5] Shademan, A., Decker, S. J., Opfermann, J. D., Leonard, S., & Krieger, A. (2016). Surgical robotics: Applications and future directions. Proceedings of the IEEE, 104(1), 81-96.
[6] Churpek, M. M., Yuen, T. C., Winslow, C., Meltzer, D. O., & Kattan, M. W. (2016). Multicenter comparison of machine learning methods and conventional regression for predicting clinical deterioration on the wards. Critical Care Medicine, 44(2), 368.
[7] Mahmood, F., Rahmani, F., Singh, A., Subramaniam, B., & Novack, V. (2018). Machine learning and artificial intelligence for perioperative care: current landscape and future directions. Best Practice & Research Clinical Anaesthesiology, 32(4), 469-481.
[8] Weng, S. F., Reps, J., Kai, J., Garmo, H., Khan, K. S., Keogh, B. E., & Gulliford, M. C. (2017). Can machine-learning improve cardiovascular risk prediction using routine clinical data?. PloS one, 12(12), e0189790.
[9] Mega, J. L., Close, S. L., Wiviott, S. D., Shen, L., Walker, P. L., Macias, W., … & O’Donoghue, M. L. (2009). Cytochrome P-450 polymorphisms and response to clopidogrel. New England Journal of Medicine, 360(4), 354-362.
[10] Patel, S., Park, H., Bonato, P., Chan, L., & Rodgers, M. (2012). A review of wearable sensors and systems with application in rehabilitation. Journal of neuroengineering and rehabilitation, 9(1), 21.
[11] Swen, J. J., Nijenhuis, M., de Boer, A., Mulder, H., Rongen, G. A., van Schaik, R. H., & Klein, T. E. (2011). Pharmacogenomics: the road to personalized medicine. MAYO CLIN PROC, 86(10), 988-999.
AI-assisted surgery, you say? So, are we talking robot arms doing the Macarena during bypasses, or are they just holding the retractors? Inquiring minds (and shaky hands) want to know!
That’s a fantastic question! While we’re not quite at the ‘robot arms doing the Macarena’ stage (yet!), AI is being used to enhance precision and provide real-time guidance during surgery. Think of it as a highly skilled assistant helping surgeons make the best decisions for their patients. Exciting times ahead!
Editor: MedTechNews.Uk
Thank you to our Sponsor Esdebe