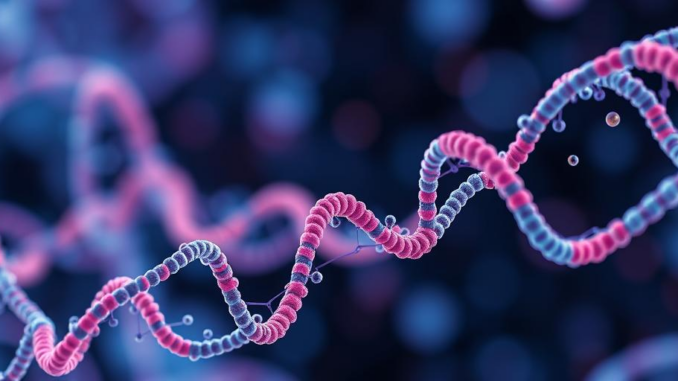
Revolutionizing Drug Discovery: A Comprehensive Analysis of Emerging Technologies and Future Prospects
Many thanks to our sponsor Esdebe who helped us prepare this research report.
Abstract
The pharmaceutical industry faces increasing challenges in drug discovery, including escalating costs, lengthy development timelines, and a high attrition rate of drug candidates. This report provides a comprehensive overview of the evolving landscape of drug discovery, exploring both established and emerging technologies poised to revolutionize the field. We analyze advancements in genomics, proteomics, and metabolomics, as well as the transformative potential of artificial intelligence (AI) and machine learning (ML). Furthermore, we delve into high-throughput screening (HTS) and combinatorial chemistry, examining their contributions and limitations. Finally, we discuss the integration of these technologies in drug discovery pipelines, analyze existing AI-driven drug pipelines, and highlight future directions, emphasizing the need for robust validation strategies and interdisciplinary collaborations to fully realize the promise of accelerated and more efficient drug development.
Many thanks to our sponsor Esdebe who helped us prepare this research report.
1. Introduction
The process of drug discovery is notoriously complex, time-consuming, and expensive. Traditionally, it involves identifying a biological target, screening vast libraries of chemical compounds for activity against that target, optimizing the most promising leads, and then conducting preclinical and clinical trials. This process can take over a decade and cost billions of dollars, with a significant percentage of drug candidates failing along the way due to lack of efficacy, toxicity, or other issues. Consequently, there is a pressing need for innovative approaches that can accelerate the process, reduce costs, and improve the success rate of drug discovery.
Several technological advancements have emerged in recent years that hold immense promise for revolutionizing drug discovery. Genomics, proteomics, and metabolomics provide unprecedented insights into the biological mechanisms underlying disease, enabling the identification of novel drug targets and the development of more targeted therapies. Artificial intelligence (AI) and machine learning (ML) are rapidly transforming the field by enabling the analysis of large datasets, predicting drug-target interactions, designing novel drug candidates, and optimizing clinical trial design. High-throughput screening (HTS) and combinatorial chemistry have also significantly contributed to accelerating the screening process and expanding the chemical space that can be explored.
This report aims to provide a comprehensive overview of these emerging technologies and their impact on drug discovery. We will explore the potential of each technology, discuss the challenges associated with their implementation, and highlight successful examples of their application in the field. We will also examine the integration of these technologies into drug discovery pipelines and discuss future directions for research and development.
Many thanks to our sponsor Esdebe who helped us prepare this research report.
2. Advances in Omics Technologies: Genomics, Proteomics, and Metabolomics
The advent of “omics” technologies – genomics, proteomics, and metabolomics – has provided researchers with an unprecedented ability to characterize biological systems at the molecular level. These technologies have revolutionized our understanding of disease mechanisms and opened up new avenues for drug discovery.
2.1 Genomics
Genomics, the study of an organism’s entire genome, has enabled the identification of genes associated with disease susceptibility, progression, and treatment response. Genome-wide association studies (GWAS) have been particularly useful in identifying genetic variants that increase the risk of common diseases such as diabetes, cardiovascular disease, and cancer. These findings can then be used to identify novel drug targets or to develop personalized therapies that are tailored to an individual’s genetic profile. Moreover, advances in gene editing technologies like CRISPR-Cas9 allow for precise manipulation of gene expression, providing powerful tools for validating drug targets and developing gene therapies.
2.2 Proteomics
Proteomics, the study of all proteins expressed by a cell or organism, provides a more direct measure of biological activity than genomics. Proteins are the workhorses of the cell, and their expression levels and post-translational modifications can be influenced by a variety of factors, including disease state, drug treatment, and environmental conditions. Proteomic technologies, such as mass spectrometry, allow for the identification and quantification of thousands of proteins in a single sample, providing a comprehensive snapshot of cellular function. This information can be used to identify novel drug targets, discover biomarkers for disease diagnosis and prognosis, and monitor the effects of drug treatment.
2.3 Metabolomics
Metabolomics, the study of all small molecule metabolites in a biological sample, provides a holistic view of cellular metabolism. Metabolites are the end products of cellular processes, and their levels can be highly sensitive to changes in disease state or drug treatment. Metabolomic technologies, such as nuclear magnetic resonance (NMR) spectroscopy and mass spectrometry, allow for the identification and quantification of hundreds or thousands of metabolites in a single sample. This information can be used to identify novel drug targets, discover biomarkers for disease diagnosis and prognosis, and monitor the effects of drug treatment. Furthermore, metabolic profiling can reveal disruptions in specific metabolic pathways associated with disease, offering opportunities for targeted drug development. For example, understanding altered glucose metabolism in cancer cells has led to the development of drugs targeting specific enzymes in the glycolytic pathway.
2.4 Integration of Omics Technologies
While each omics technology provides valuable information on its own, the integration of these technologies can provide a more comprehensive understanding of disease mechanisms and drug response. For example, integrating genomic data with proteomic and metabolomic data can help to identify causal relationships between genetic variants and protein and metabolite levels, leading to a more precise understanding of disease etiology. Similarly, integrating omics data with clinical data can help to identify biomarkers that predict drug response, enabling the development of personalized therapies. Multi-omics approaches are becoming increasingly common in drug discovery, offering the potential to accelerate the identification of novel drug targets and the development of more effective therapies. However, the analysis of such large, complex datasets requires sophisticated bioinformatics tools and expertise. Standardized data formats and analysis pipelines are crucial for ensuring reproducibility and comparability of results across different studies.
Many thanks to our sponsor Esdebe who helped us prepare this research report.
3. Artificial Intelligence and Machine Learning in Drug Discovery
Artificial intelligence (AI) and machine learning (ML) are rapidly transforming drug discovery by enabling the analysis of large datasets, predicting drug-target interactions, designing novel drug candidates, and optimizing clinical trial design. AI/ML algorithms can identify patterns and relationships in data that are difficult or impossible for humans to detect, leading to the discovery of new drug targets, the development of more effective therapies, and the acceleration of the drug discovery process.
3.1 Machine Learning for Predicting Drug-Target Interactions
One of the most important applications of ML in drug discovery is the prediction of drug-target interactions. This involves training ML algorithms on large datasets of known drug-target interactions and then using these algorithms to predict the interactions of new drug candidates with potential targets. ML algorithms can also be used to predict the binding affinity of drugs to their targets, which is a critical factor in determining drug efficacy.
Several ML algorithms have been successfully applied to predict drug-target interactions, including support vector machines (SVMs), random forests, and neural networks. These algorithms can be trained on a variety of data, including chemical structure data, genomic data, and proteomic data. Deep learning models, in particular, have shown remarkable performance in predicting drug-target interactions due to their ability to learn complex patterns from high-dimensional data. For example, convolutional neural networks (CNNs) can be used to analyze the 3D structure of proteins and predict their interactions with drug molecules.
3.2 Generative Models for Designing New Drug Candidates
Another important application of AI in drug discovery is the design of new drug candidates. Generative models, such as variational autoencoders (VAEs) and generative adversarial networks (GANs), can be trained on large datasets of known drug molecules and then used to generate novel molecules with desired properties. These models can be used to design molecules that are likely to bind to a specific target, have good bioavailability, and are not toxic. This capability is critical in overcoming the limitations of traditional lead discovery approaches which are often limited by the available chemical space. AI can explore and generate novel chemical entities beyond existing libraries.
3.3 AI in Clinical Trials
AI is also being used to optimize clinical trial design. For example, AI algorithms can be used to identify patients who are most likely to respond to a particular drug, allowing for the recruitment of more targeted patient populations. AI can also be used to monitor patients during clinical trials and to identify potential safety signals early on. This can help to improve the efficiency and safety of clinical trials, and to reduce the risk of drug failure.
3.4 Challenges of Validating AI-Generated Drug Candidates
While AI has shown great promise in drug discovery, there are also several challenges associated with its implementation. One of the biggest challenges is the validation of AI-generated drug candidates. AI algorithms are often trained on large datasets of historical data, which may not be representative of the real world. This can lead to the generation of drug candidates that are not effective or are toxic. Therefore, it is crucial to validate AI-generated drug candidates using rigorous experimental methods, such as in vitro and in vivo studies. Specifically, the black-box nature of some AI algorithms makes it difficult to understand the rationale behind their predictions. This lack of interpretability can hinder the validation process, as researchers may struggle to identify the underlying mechanisms of action of AI-generated drug candidates. Addressing this challenge requires the development of more transparent and interpretable AI models.
3.5 Cost-Effectiveness of Using AI Compared to Traditional Drug Discovery Methods
While the upfront investment in AI infrastructure and expertise can be significant, the long-term cost-effectiveness of using AI in drug discovery is likely to be substantial. AI can accelerate the drug discovery process, reduce the cost of screening, and improve the success rate of drug candidates. This can lead to significant cost savings for pharmaceutical companies. However, a comprehensive cost-benefit analysis is crucial to fully evaluate the economic impact of AI in drug discovery. This analysis should consider factors such as the cost of AI infrastructure, the cost of training and validating AI models, and the potential cost savings from accelerated drug discovery and reduced attrition rates. Furthermore, the cost-effectiveness of AI may vary depending on the specific application and the complexity of the disease being targeted.
Many thanks to our sponsor Esdebe who helped us prepare this research report.
4. High-Throughput Screening and Combinatorial Chemistry
High-throughput screening (HTS) and combinatorial chemistry are two technologies that have played a significant role in accelerating the drug discovery process. HTS involves the rapid screening of large libraries of chemical compounds for activity against a specific biological target. Combinatorial chemistry involves the synthesis of large numbers of different chemical compounds in a short amount of time. These technologies have enabled researchers to screen millions of compounds for potential drug candidates, significantly increasing the chances of finding a lead compound.
4.1 High-Throughput Screening (HTS)
HTS typically involves the use of automated robotic systems to perform assays on a large number of samples simultaneously. These assays can be designed to measure a variety of different parameters, such as enzyme activity, cell proliferation, and receptor binding. HTS has been successfully used to identify drug candidates for a wide range of diseases, including cancer, infectious diseases, and neurological disorders. Miniaturization and automation are key features of HTS, enabling the efficient screening of vast compound libraries. However, HTS can generate a large number of false positives, requiring further validation. Furthermore, the cost of maintaining HTS infrastructure and the need for specialized expertise can be significant barriers to adoption.
4.2 Combinatorial Chemistry
Combinatorial chemistry allows for the rapid synthesis of a vast number of diverse chemical compounds. These compounds can be synthesized using a variety of different chemical reactions, and the products can be purified and characterized using automated systems. Combinatorial chemistry has been used to generate libraries of compounds that are structurally diverse and that have a wide range of biological activities. Parallel synthesis and solid-phase synthesis are common techniques used in combinatorial chemistry. However, ensuring the quality and purity of compounds generated through combinatorial chemistry can be challenging. Furthermore, the diversity of compounds generated may not always be relevant to the specific target of interest.
4.3 Limitations and Synergies
While HTS and combinatorial chemistry have been valuable tools in drug discovery, they also have some limitations. HTS can be expensive and time-consuming, and it can generate a large number of false positives. Combinatorial chemistry can be difficult to control, and it can generate compounds that are not biologically active. However, these technologies can be used in combination to overcome these limitations. For example, combinatorial chemistry can be used to generate libraries of compounds that are specifically designed to bind to a particular target, and HTS can then be used to screen these libraries for activity against that target. The combination of HTS and combinatorial chemistry can be further enhanced by integrating AI/ML techniques. AI can be used to prioritize compounds for synthesis based on predicted activity and to analyze HTS data to identify promising lead compounds. This synergy can significantly accelerate the drug discovery process.
Many thanks to our sponsor Esdebe who helped us prepare this research report.
5. Current Drug Pipeline and Projected Benefits
The integration of AI and other emerging technologies into the drug discovery pipeline is already showing promising results, with a growing number of AI-designed drugs entering clinical trials. These drugs span a range of therapeutic areas, including oncology, neurology, and infectious diseases. Several companies, such as Exscientia, Atomwise, and Insilico Medicine, are at the forefront of AI-driven drug discovery and have a number of promising drug candidates in their pipelines.
5.1 Current AI-Driven Drug Pipelines
- Exscientia: Several molecules in clinical trials across different indications. For instance, they have partnered with various pharmaceutical companies to develop novel therapies using their AI-driven platform.
- Atomwise: Atomwise’s AI platform has aided in the discovery of drug candidates for various diseases, and they have numerous ongoing partnerships and projects.
- Insilico Medicine: Insilico Medicine focuses on AI-driven drug discovery and development, with drug candidates in various stages of preclinical and clinical development. Their pipeline includes potential treatments for fibrosis and other diseases.
- BenevolentAI: BenevolentAI is another company utilizing AI to accelerate drug discovery. They have programs targeting various diseases, leveraging AI to identify novel drug targets and develop potential therapies.
5.2 Projected Benefits of AI in Drug Discovery
The widespread adoption of AI and other emerging technologies in drug discovery is projected to have several significant benefits, including:
- Reduced drug discovery timelines: AI can accelerate the identification of drug targets, the design of drug candidates, and the optimization of clinical trial design, leading to a significant reduction in the overall drug discovery timeline.
- Lower drug development costs: By accelerating the drug discovery process and improving the success rate of drug candidates, AI can significantly reduce the cost of drug development.
- Increased success rates: AI can help to identify drug candidates that are more likely to be effective and safe, leading to an increase in the success rate of drug discovery.
- Development of personalized therapies: AI can be used to identify patients who are most likely to respond to a particular drug, enabling the development of personalized therapies that are tailored to an individual’s genetic profile and other characteristics.
- Discovery of drugs for previously untreatable diseases: AI can help to identify novel drug targets and develop drugs for diseases that have previously been considered untreatable.
These projected benefits underscore the transformative potential of AI and other emerging technologies in drug discovery, highlighting the need for continued investment and research in this area.
Many thanks to our sponsor Esdebe who helped us prepare this research report.
6. Challenges and Future Directions
Despite the significant progress that has been made in recent years, there are still several challenges that need to be addressed in order to fully realize the potential of emerging technologies in drug discovery.
6.1 Data Quality and Availability
One of the biggest challenges is the lack of high-quality data. AI algorithms are only as good as the data they are trained on, so it is crucial to have access to large, well-curated datasets. However, much of the data that is currently available is incomplete, inaccurate, or inconsistent. Furthermore, there is a lack of standardization in data formats and data sharing practices, which makes it difficult to integrate data from different sources. Efforts to improve data quality and availability are essential for the successful application of AI in drug discovery. Establishing standardized data formats, promoting data sharing, and developing robust data validation methods are crucial steps in this direction.
6.2 Model Interpretability and Explainability
As previously mentioned, the lack of interpretability of some AI models can be a significant barrier to their adoption in drug discovery. Researchers need to be able to understand how AI algorithms are making their predictions in order to validate their results and to gain insights into the mechanisms of action of drug candidates. The development of more transparent and interpretable AI models is a key area for future research. Techniques such as attention mechanisms and explainable AI (XAI) methods can help to improve the interpretability of AI models.
6.3 Integration of Multimodal Data
Drug discovery involves the integration of data from a variety of different sources, including genomics, proteomics, metabolomics, clinical data, and imaging data. AI algorithms need to be able to integrate these different types of data in order to make accurate predictions. However, the integration of multimodal data is a challenging task, as different types of data have different formats, scales, and noise levels. The development of new AI algorithms that can effectively integrate multimodal data is a key area for future research.
6.4 Ethical Considerations
The use of AI in drug discovery raises several ethical considerations. For example, there is a risk that AI algorithms could be biased, leading to the development of drugs that are not effective or safe for certain populations. It is important to ensure that AI algorithms are trained on diverse datasets and that they are rigorously tested to identify and mitigate potential biases. Furthermore, there are ethical considerations related to the privacy and security of patient data. It is important to protect patient data and to ensure that it is used responsibly and ethically.
6.5 Interdisciplinary Collaboration
The successful application of emerging technologies in drug discovery requires interdisciplinary collaboration between scientists from a variety of different fields, including biology, chemistry, computer science, and medicine. It is important to foster collaboration between these different disciplines in order to overcome the challenges and to realize the full potential of emerging technologies in drug discovery. Establishing interdisciplinary research teams, promoting cross-training, and organizing interdisciplinary conferences and workshops are effective ways to foster collaboration.
Many thanks to our sponsor Esdebe who helped us prepare this research report.
7. Conclusion
The pharmaceutical industry is at the cusp of a significant transformation, driven by the confluence of advances in genomics, proteomics, metabolomics, AI/ML, HTS, and combinatorial chemistry. These technologies offer the potential to accelerate drug discovery, reduce costs, and improve success rates. While challenges remain, including data quality, model interpretability, and ethical considerations, the ongoing advancements in these areas are paving the way for a new era of drug development. Interdisciplinary collaboration, robust validation strategies, and a commitment to ethical principles will be critical to fully harnessing the power of these emerging technologies to address unmet medical needs and improve human health.
Many thanks to our sponsor Esdebe who helped us prepare this research report.
References
- Vamathevan, J., Clark, D., Czodrowski, P., Ecker, G. F., Laufer, S. A., et al. (2019). Applications of machine learning in drug discovery and development. Nature Reviews Drug Discovery, 18(6), 463-480.
- Paul, D., Sanap, G., Shenoy, S., Kalyane, D., Kalia, K., & Tekade, R. K. (2021). Artificial intelligence in drug discovery and development. Drug Discovery Today, 26(1), 80-93.
- Schneider, G. (2018). Artificial intelligence in drug discovery. Nature Reviews Drug Discovery, 17(5), 353-368.
- De Albuquerque, C. P., Oprea, T. I., & Bologa, C. G. (2021). Artificial intelligence in drug discovery: what is next?. Future Medicinal Chemistry, 13(11), 1127-1130.
- Zhavoronkov, A. et al. (2019). Deep learning enables rapid identification of potent DDR1 kinase inhibitors. Nature Biotechnology, 37(9), 1038-1040.
- Lavecchia, A. (2015). Machine-learning approaches in drug discovery: methods and applications. Drug Discovery Today, 20(3), 318-331.
- Hughes, J. P., Rees, S., Kalindjian, S. B., Philpott, K. L., Mügs, J., & Benson, C. (2011). Principles of early drug discovery. British Journal of Pharmacology, 162(6), 1239-1249.
- Sliwoski, G., Kothiwale, S., Meiler, J., & Lowe, E. W., Jr. (2014). Computational methods in drug discovery. Pharmacological Reviews, 66(1), 334-395.
- Agatonovic-Kustrin, S., & Beresford, R. (2000). High-throughput screening: a perspective. Chemoinformatics, 2(1), 28-38.
- Czarnik, A. W. (1997). Combinatorial chemistry: from test tube to factory. Accounts of Chemical Research, 30(7), 292-302.
- Moffat, J. G., Rudolph, C. J., & Bailey, D. (2017). High-throughput screening hits to leads: progress, challenges, and opinions. ACS Chemical Biology, 12(3), 511-521.
- Ramsden, J. J. (2009). Bioinformatics: an introduction. Springer Science & Business Media.
Be the first to comment