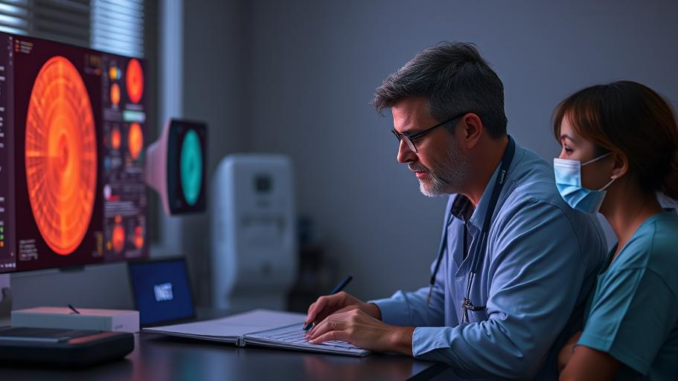
Medical Imaging: A Comprehensive Review of Modalities, Advances, and Translational Challenges
Abstract
Medical imaging has become indispensable in modern healthcare, playing a crucial role in diagnosis, treatment planning, and monitoring of disease progression. This report provides a comprehensive overview of the current state of medical imaging, encompassing various modalities such as X-ray, computed tomography (CT), magnetic resonance imaging (MRI), ultrasound (US), and nuclear medicine techniques (PET/SPECT). We delve into the principles, strengths, and limitations of each modality, highlighting their specific applications in different clinical scenarios. Furthermore, we explore the recent advancements in medical imaging, particularly focusing on the integration of artificial intelligence (AI) and virtual reality (VR). The report also addresses the significant challenges associated with translating novel imaging technologies into routine clinical practice, including regulatory hurdles, cost-effectiveness considerations, and the need for robust clinical validation. Finally, we discuss the ethical implications surrounding the use of AI in medical imaging and the impact of new technologies such as photon counting CT. Our aim is to provide a detailed and nuanced perspective on the current landscape of medical imaging, offering insights for researchers, clinicians, and policymakers alike.
1. Introduction
Medical imaging has revolutionized healthcare, providing non-invasive and minimally invasive methods for visualizing the internal structures and functions of the human body. From the initial discovery of X-rays by Wilhelm Conrad Röntgen in 1895 to the sophisticated imaging modalities available today, the field has experienced exponential growth, transforming the way diseases are diagnosed, treated, and monitored. Medical imaging modalities are now essential tools, with clinical application across almost all areas of medicine.
This report aims to provide a comprehensive review of the current state of medical imaging, covering the fundamental principles of each major modality, their strengths and weaknesses, and recent technological advancements. Furthermore, we will address the challenges associated with the translation of novel imaging techniques from the laboratory to the clinic, a critical step in realizing their full potential for improving patient care. We will focus on the application of AI and VR, and the challenges of introducing new technologies to clinical practice.
2. Current Medical Imaging Modalities
Medical imaging encompasses a diverse range of modalities, each relying on different physical principles to generate images. Understanding the underlying principles and characteristics of each modality is crucial for selecting the appropriate technique for a specific clinical application.
2.1 X-ray Radiography
X-ray radiography, the oldest and most widely used imaging modality, utilizes ionizing radiation to produce images of bones and other dense structures. X-rays are attenuated as they pass through the body, and the degree of attenuation depends on the density and composition of the tissues. The transmitted X-rays are captured by a detector, creating a shadowgraph image that reveals differences in tissue density. While simple and readily available, conventional X-ray radiography provides limited soft tissue contrast. Digital radiography has largely replaced film-based systems, offering improved image quality, reduced radiation dose, and enhanced image processing capabilities. However, despite ongoing advancements in image processing, traditional X-ray radiography still suffers from limitations in sensitivity and specificity for detecting subtle abnormalities.
2.2 Computed Tomography (CT)
Computed tomography (CT) overcomes the limitations of conventional radiography by acquiring multiple X-ray projections from different angles. These projections are then reconstructed using sophisticated algorithms to create cross-sectional images of the body. CT provides excellent spatial resolution and superior soft tissue contrast compared to X-ray radiography. Multidetector CT (MDCT) scanners have further improved imaging speed and resolution, enabling the acquisition of thin-slice images that can be reconstructed in multiple planes. CT angiography (CTA) is a specialized CT technique that utilizes intravenous contrast agents to visualize blood vessels. While CT is a powerful imaging tool, it involves higher radiation doses than X-ray radiography, raising concerns about potential long-term risks, particularly with repeated examinations. Iterative reconstruction techniques have been developed to reduce radiation dose while maintaining image quality. More recently, photon-counting CT, in which individual photons are counted, promise to offer increased contrast-to-noise ratio and reduced radiation dose compared to standard CT. However, these machines are currently expensive and require significant image processing to work optimally.
2.3 Magnetic Resonance Imaging (MRI)
Magnetic resonance imaging (MRI) is a non-ionizing imaging modality that utilizes strong magnetic fields and radiofrequency waves to generate images. MRI exploits the magnetic properties of atomic nuclei, particularly hydrogen protons, to create detailed images of soft tissues. Different tissues exhibit varying magnetic resonance properties, allowing for excellent contrast resolution. MRI is particularly valuable for imaging the brain, spinal cord, musculoskeletal system, and abdominal organs. Various MRI techniques, such as diffusion-weighted imaging (DWI), perfusion imaging, and functional MRI (fMRI), provide additional information about tissue microstructure, blood flow, and brain activity. The major disadvantages of MRI include its relatively long scan times, high cost, and contraindications for patients with certain metallic implants. The presence of a strong magnetic field also necessitates strict safety protocols to prevent accidents.
2.4 Ultrasound (US)
Ultrasound (US) is a real-time imaging modality that utilizes high-frequency sound waves to create images. A transducer emits sound waves that penetrate the body and are reflected back from tissue interfaces. The reflected waves are processed to create an image. Ultrasound is widely used for obstetrical imaging, abdominal imaging, and musculoskeletal imaging. It is a relatively inexpensive and portable imaging technique that does not involve ionizing radiation. Doppler ultrasound is a specialized technique that measures blood flow velocity. The limitations of ultrasound include its dependence on operator skill, limited penetration depth, and poor image quality in patients with obesity or bowel gas. Contrast-enhanced ultrasound (CEUS) utilizes microbubble contrast agents to improve image quality and enhance the visualization of blood vessels.
2.5 Nuclear Medicine (PET/SPECT)
Nuclear medicine techniques, including positron emission tomography (PET) and single-photon emission computed tomography (SPECT), utilize radioactive tracers to visualize physiological processes within the body. These tracers, known as radiopharmaceuticals, are administered intravenously and accumulate in specific tissues or organs. PET detects the annihilation photons produced by positron-emitting isotopes, while SPECT detects the gamma rays emitted by single-photon-emitting isotopes. PET and SPECT are particularly valuable for imaging cancer, cardiac disease, and neurological disorders. PET/CT and SPECT/CT combine the functional information provided by nuclear medicine with the anatomical detail provided by CT. The use of targeted radiopharmaceuticals allows for the detection of specific molecular targets, such as cancer cell receptors. The main limitations of nuclear medicine techniques include the use of ionizing radiation and the limited spatial resolution.
3. Recent Advances in Medical Imaging
The field of medical imaging is constantly evolving, with ongoing advancements in technology, image processing, and data analysis. These advancements are driven by the desire to improve image quality, reduce radiation dose, enhance diagnostic accuracy, and streamline clinical workflows.
3.1 Artificial Intelligence (AI) in Medical Imaging
Artificial intelligence (AI), particularly deep learning, has emerged as a transformative force in medical imaging. AI algorithms can be trained to perform a variety of tasks, including image reconstruction, image segmentation, lesion detection, and disease classification. AI-powered image reconstruction algorithms can reduce noise and artifacts, enabling the acquisition of high-quality images with lower radiation doses. AI-based image segmentation tools can automatically delineate organs and tissues, reducing the time required for manual segmentation. AI algorithms can also be trained to detect subtle abnormalities that may be missed by human observers, improving diagnostic accuracy. For example, AI has been applied to the detection of lung nodules on CT scans, breast cancer on mammograms, and brain tumors on MRI scans. The FDA has approved several AI-based medical imaging applications for clinical use, and the number is expected to grow rapidly. However, ethical concerns exist with the use of AI, around bias, explainability, data privacy and accountability. AI requires large datasets of labelled images, and any bias in these training images can be inherited by the resulting algorithms. Furthermore, the ‘black box’ nature of many deep learning algorithms makes it difficult to understand how they arrive at their decisions, raising concerns about transparency and trust. AI needs to be appropriately implemented and regulated.
3.2 Virtual Reality (VR) in Medical Imaging
Virtual reality (VR) is an emerging technology that has the potential to revolutionize medical imaging. VR allows users to interact with 3D medical images in an immersive and intuitive way. VR can be used for surgical planning, medical education, and patient communication. Surgeons can use VR to practice complex procedures before performing them on patients, improving surgical outcomes. Medical students can use VR to explore anatomical structures and disease processes in a realistic and engaging environment. Patients can use VR to visualize their own medical images and better understand their condition. The cost of VR hardware and software has decreased significantly in recent years, making it more accessible for clinical use. The main challenges associated with VR in medical imaging include the need for high-quality 3D reconstructions, the development of user-friendly interfaces, and the integration of VR into clinical workflows. VR has clear application in training doctors and in visualising radiology for MDTs.
3.3 Advanced Image Reconstruction Techniques
Iterative reconstruction techniques have been developed to reduce radiation dose in CT while maintaining image quality. These techniques use sophisticated algorithms to iteratively refine the image until it matches the acquired data. Compressed sensing is another advanced image reconstruction technique that can reduce scan time and radiation dose by acquiring fewer data points. Deep learning-based image reconstruction algorithms are also being developed, which can further improve image quality and reduce radiation dose. These advanced image reconstruction techniques are becoming increasingly important as efforts are made to minimize radiation exposure to patients.
3.4 Molecular Imaging
Molecular imaging is a rapidly growing field that aims to visualize biological processes at the molecular level. Molecular imaging techniques utilize targeted imaging agents that bind to specific molecular targets, such as cancer cell receptors. These agents can be detected using a variety of imaging modalities, including PET, SPECT, MRI, and optical imaging. Molecular imaging has the potential to improve the diagnosis, treatment, and monitoring of a wide range of diseases, including cancer, cardiovascular disease, and neurological disorders. The development of new targeted imaging agents is a major focus of research in this field.
4. Challenges in Translating New Imaging Technologies into Clinical Practice
While the development of new medical imaging technologies holds great promise, translating these technologies into routine clinical practice is a complex and challenging process. Several barriers can hinder the adoption of new imaging techniques, including regulatory hurdles, cost-effectiveness considerations, and the need for robust clinical validation.
4.1 Regulatory Approval
New medical imaging technologies must undergo rigorous regulatory review before they can be marketed and used in clinical practice. Regulatory agencies, such as the FDA in the United States and the EMA in Europe, require extensive data on the safety and efficacy of new devices. The regulatory approval process can be lengthy and expensive, which can discourage innovation. Furthermore, regulatory requirements may vary across different countries, making it difficult for companies to market their products globally. The regulatory process needs to keep pace with technology development, to ensure that patients can benefit as early as possible.
4.2 Cost-Effectiveness
The cost of new medical imaging technologies is a major barrier to their adoption. Advanced imaging modalities, such as PET/MRI and photon counting CT, are significantly more expensive than conventional imaging techniques. Healthcare providers must carefully consider the cost-effectiveness of new technologies before investing in them. Cost-effectiveness analyses should take into account not only the initial purchase price but also the operating costs, maintenance costs, and the potential benefits to patients. Studies are needed to demonstrate the value of new technologies in terms of improved diagnostic accuracy, reduced treatment costs, and improved patient outcomes. Government support is required to support the roll out of these expensive technologies in poorer regions.
4.3 Clinical Validation
New medical imaging technologies must be rigorously validated in clinical trials before they can be widely adopted. Clinical trials should be designed to assess the diagnostic accuracy, sensitivity, and specificity of new techniques. It is important to compare new techniques to existing standard-of-care imaging modalities to determine their added value. Clinical trials should also assess the impact of new technologies on patient outcomes, such as survival rates and quality of life. The results of clinical trials should be published in peer-reviewed journals to allow for independent evaluation. Clinical validation is essential to ensure that new technologies are safe, effective, and beneficial to patients.
4.4 Training and Expertise
The successful implementation of new medical imaging technologies requires adequate training and expertise. Radiologists, technologists, and other healthcare professionals must be trained on the proper use of new equipment and software. Training should include both theoretical knowledge and hands-on experience. It is also important to establish quality control programs to ensure that imaging procedures are performed consistently and accurately. Continuing education is essential to keep healthcare professionals up-to-date on the latest advancements in medical imaging. New modalities such as photon counting CT have different image characteristics to standard CT, meaning that AI algorithms and reporting techniques need to be adapted to these new modalities.
4.5 Integration into Clinical Workflows
New medical imaging technologies must be seamlessly integrated into existing clinical workflows. This requires careful planning and coordination between different departments and healthcare professionals. Imaging protocols should be standardized to ensure consistency across different sites. IT infrastructure must be upgraded to accommodate the large amounts of data generated by advanced imaging modalities. Electronic health records (EHRs) should be integrated with imaging systems to allow for easy access to patient information. Workflow optimization is essential to ensure that new technologies improve efficiency and do not create unnecessary delays.
5. Ethical Considerations
The increasing use of AI in medical imaging raises important ethical considerations. AI algorithms can be biased if they are trained on biased data, leading to disparities in healthcare. The lack of transparency in AI decision-making can also be problematic, as it can be difficult to understand why an AI algorithm made a particular diagnosis. Data privacy and security are also major concerns, as AI algorithms require access to large amounts of patient data. It is important to develop ethical guidelines and regulations for the use of AI in medical imaging to ensure that these technologies are used responsibly and ethically. These guidelines must strike a balance between promoting innovation and protecting patient rights. Furthermore, the role of radiologists in the era of AI needs to be carefully considered. While AI can assist radiologists in performing certain tasks, it is important to maintain human oversight and ensure that radiologists retain their critical role in interpreting images and making clinical decisions.
6. The Future of Medical Imaging
The future of medical imaging is bright, with ongoing advancements in technology, image processing, and data analysis. We can expect to see further integration of AI and VR into clinical practice, leading to improved diagnostic accuracy, reduced radiation dose, and enhanced patient care. Molecular imaging will continue to play an increasingly important role in the diagnosis and treatment of disease, allowing for the visualization of biological processes at the molecular level. Personalized imaging approaches will be developed, tailoring imaging protocols to individual patient characteristics and needs. The development of new imaging agents and contrast agents will further enhance the capabilities of medical imaging. Ultimately, the goal of medical imaging is to provide clinicians with the tools they need to make accurate diagnoses, plan effective treatments, and monitor patient outcomes, improving the health and well-being of individuals around the world.
7. Conclusion
Medical imaging is a constantly evolving field that has transformed healthcare, providing invaluable tools for diagnosis, treatment planning, and disease monitoring. This report has provided a comprehensive overview of the current state of medical imaging, encompassing various modalities, recent advancements, and translational challenges. The integration of AI and VR, along with the development of advanced image reconstruction techniques and molecular imaging agents, holds great promise for the future of medical imaging. However, it is crucial to address the ethical considerations and challenges associated with the adoption of new technologies to ensure that they are used responsibly and effectively. By overcoming these challenges, we can unlock the full potential of medical imaging to improve patient care and advance medical knowledge.
References
- American College of Radiology. (n.d.). ACR–SPR Practice Parameter for Imaging of the Chest—Suspected Pulmonary Nodules. Retrieved from https://www.acr.org/Clinical-Resources/Practice-Parameters-and-Technical-Standards/Practice-Parameters
- Brady, A. P., Crowe, P. M., & Stack, J. P. (2012). Radiology and the increasing demand for medical imaging: a comparative study of radiology workload in Ireland, 2003-2010. Journal of the Royal Society of Medicine, 105(3), 116-121.
- Chan, H. P., Samala, R. K., Hadjiiski, L. M., Helvie, M. A., & Cha, K. H. (2020). Deep learning in medical image analysis. Annual review of biomedical engineering, 22, 261-286.
- European Medicines Agency. (n.d.). Medical devices. Retrieved from https://www.ema.europa.eu/en/human-regulatory/overview/medical-devices
- Hosny, A., Parmar, C., Quackenbush, J., Schwartz, L. H., & Aerts, H. J. W. L. (2018). Artificial intelligence in radiology. Nature Reviews Cancer, 18(8), 500-510.
- Lazarus, E., Haramati, L. B., & Haramati, N. (2020). Virtual reality for medical education and training. Academic Radiology, 27(10), 1409-1417.
- McCollough, C. H., Leng, S., Yu, L., & Fletcher, J. G. (2015). Dual-energy CT in clinical practice. American Journal of Roentgenology, 204(6), 1162-1170.
- National Cancer Institute. (n.d.). Molecular Imaging. Retrieved from https://www.cancer.gov/about-cancer/diagnosis-staging/diagnosis/molecular-imaging-fact-sheet
- U.S. Food and Drug Administration. (n.d.). Medical Devices. Retrieved from https://www.fda.gov/medical-devices
- D’Orsi CJ, Sickles EA, Mendelson EB, et al. ACR BI-RADS Atlas, Breast Imaging Reporting and Data System. Reston, VA: American College of Radiology; 2013.
- Tang, X., & LeCun, Y. (1993). What can we expect from very large scale neural network when applied to image recognition. In Advances in neural information processing systems (pp. 687-694).
- Rajpurkar P, Irvin J, Ball RL, et al. Deep learning for chest radiograph diagnosis: a retrospective study. PLoS Med. 2017;14(11):e1002426. Published 2017 Nov 7. doi:10.1371/journal.pmed.1002426
- Mannil, M. P., Oddsson, A., & Laukemper, R. (2022). Photon-counting computed tomography. European radiology experimental, 6(1), 67. doi: 10.1186/s41747-022-00318-x
- Fuchs, T. J., & Buhmann, J. M. (2011). Medical image analysis with convolutional neural networks. In Proceedings of the annual symposium on information processing in medical imaging (pp. 488-499). Springer, Berlin, Heidelberg.
- Litjens, G., Kooi, T., Bejnordi, B. E., Setio, A. A. A., Ciompi, F., Ghafoorian, M., … & Sánchez, C. I. (2017). A survey on deep learning in medical image analysis. Medical image analysis, 42, 60-88.
Be the first to comment