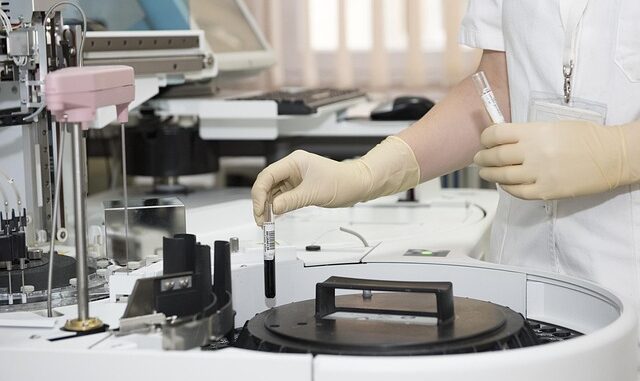
Abstract
Automation, encompassing a spectrum of technologies from Robotic Process Automation (RPA) to Artificial Intelligence (AI) and Machine Learning (ML), is rapidly reshaping industries worldwide. Healthcare, a sector traditionally reliant on manual processes and human expertise, stands to benefit significantly from the judicious application of automation. This report provides a comprehensive review of the diverse applications of automation in healthcare, spanning clinical, administrative, and research domains. We explore specific use cases, including diagnosis and treatment planning enhanced by AI-driven image analysis, drug discovery accelerated through computational modeling, and administrative tasks streamlined via RPA. Furthermore, the report delves into the potential challenges and ethical considerations associated with widespread automation in healthcare, such as algorithmic bias, data privacy concerns, and the impact on the healthcare workforce. By critically examining both the opportunities and risks, this review aims to provide a nuanced understanding of the transformative potential of automation in healthcare and inform future research and implementation strategies.
Many thanks to our sponsor Esdebe who helped us prepare this research report.
1. Introduction
Healthcare systems globally are facing increasing pressure from aging populations, rising costs, and workforce shortages. These challenges necessitate innovative solutions to improve efficiency, enhance patient outcomes, and reduce the burden on healthcare professionals. Automation, defined broadly as the use of technology to perform tasks with minimal human intervention, presents a promising avenue for addressing these challenges. While the term “automation” often conjures images of physical robots, its application in healthcare extends far beyond robotics to encompass software-based tools and algorithms that automate cognitive and administrative processes. From RPA automating medical record issuance (as highlighted in the prompt’s context) to AI-powered diagnostic systems, automation’s potential impact on healthcare is profound.
This report aims to provide a comprehensive overview of the current landscape of automation in healthcare, focusing on its diverse applications, potential benefits, and associated challenges. Unlike studies focusing solely on specific automation technologies or clinical areas, this review adopts a holistic perspective, examining the integration of various automation modalities across the entire healthcare ecosystem. We delve into the theoretical underpinnings of different automation techniques, their practical implementation in real-world healthcare settings, and the ethical considerations that must be addressed to ensure responsible and equitable deployment. The report is structured to first outline the key automation technologies relevant to healthcare, then explore their applications in various domains, and finally address the challenges and ethical considerations surrounding their adoption.
Many thanks to our sponsor Esdebe who helped us prepare this research report.
2. Key Automation Technologies in Healthcare
Several automation technologies are playing an increasingly important role in transforming healthcare. These technologies often work synergistically to provide comprehensive solutions.
2.1 Robotic Process Automation (RPA)
RPA involves the use of software “robots” to automate repetitive, rule-based tasks traditionally performed by humans. These robots can interact with existing software applications and systems, such as Electronic Health Records (EHRs) and billing platforms, without requiring significant changes to the underlying infrastructure. In healthcare, RPA is particularly well-suited for automating administrative tasks, such as:
- Claims Processing: Automating the submission, validation, and processing of insurance claims, reducing errors and accelerating payment cycles.
- Medical Record Management: Automating the retrieval, indexing, and archiving of medical records, improving accessibility and reducing administrative burden.
- Appointment Scheduling: Automating appointment scheduling and reminders, optimizing resource utilization and reducing no-show rates.
- Supply Chain Management: Automating the ordering and tracking of medical supplies, ensuring adequate stock levels and minimizing waste.
2.2 Artificial Intelligence (AI) and Machine Learning (ML)
AI and ML are transforming healthcare by enabling computers to learn from data, identify patterns, and make predictions. These technologies are used in a wide range of applications, including:
- Diagnosis: AI-powered image analysis tools can assist radiologists in detecting diseases like cancer from medical images with improved accuracy and speed. ML algorithms can analyze patient data to predict the likelihood of specific conditions, enabling earlier intervention.
- Treatment Planning: AI can personalize treatment plans by analyzing patient characteristics, medical history, and genetic information to identify the most effective therapies.
- Drug Discovery: ML algorithms can analyze vast datasets of chemical compounds and biological targets to identify promising drug candidates, accelerating the drug discovery process.
- Predictive Analytics: ML models can predict patient readmission rates, identify patients at risk of developing chronic diseases, and forecast demand for healthcare services, enabling proactive interventions and resource allocation.
2.3 Natural Language Processing (NLP)
NLP focuses on enabling computers to understand and process human language. In healthcare, NLP is used for:
- Clinical Documentation Analysis: NLP can extract key information from clinical notes, such as diagnoses, medications, and allergies, to improve data quality and support clinical decision-making.
- Sentiment Analysis: NLP can analyze patient feedback and social media data to gauge patient satisfaction and identify areas for improvement.
- Chatbots and Virtual Assistants: NLP-powered chatbots can provide patients with information, schedule appointments, and answer frequently asked questions, improving access to care and reducing the workload on healthcare staff.
2.4 Robotics
Robotics in healthcare encompasses a range of applications, from surgical robots to automated dispensing systems:
- Surgical Robotics: Surgical robots enable surgeons to perform complex procedures with greater precision, dexterity, and control, resulting in smaller incisions, reduced blood loss, and faster recovery times.
- Rehabilitation Robotics: Robotic devices can assist patients with rehabilitation exercises, improving strength, mobility, and coordination.
- Pharmacy Automation: Automated dispensing systems can accurately and efficiently dispense medications, reducing medication errors and improving pharmacy workflow.
- Cleaning and Disinfection Robots: Autonomous robots equipped with UV light or chemical disinfectants can sanitize hospital rooms and other healthcare facilities, reducing the spread of infections.
Many thanks to our sponsor Esdebe who helped us prepare this research report.
3. Applications of Automation in Healthcare
Automation is transforming various aspects of healthcare delivery, from clinical practice to administrative operations.
3.1 Clinical Applications
Automation is revolutionizing clinical practice by enabling more accurate diagnoses, personalized treatment plans, and improved patient outcomes.
- Diagnostic Imaging: AI-powered image analysis tools can detect subtle anomalies in medical images that might be missed by human radiologists, leading to earlier and more accurate diagnoses of conditions like cancer, stroke, and heart disease. For example, deep learning algorithms have demonstrated superhuman performance in detecting diabetic retinopathy from retinal images and identifying lung nodules from CT scans [1].
- Precision Medicine: AI and ML algorithms can analyze patient data, including genetic information, to identify biomarkers that predict treatment response and personalize treatment plans accordingly. This approach is particularly promising in oncology, where AI can help select the most effective therapies based on a patient’s individual tumor profile [2].
- Remote Patient Monitoring: Wearable sensors and remote monitoring devices can collect physiological data, such as heart rate, blood pressure, and glucose levels, which can be analyzed by AI algorithms to detect early signs of deterioration and trigger alerts to healthcare providers. This enables proactive interventions and reduces the need for hospital readmissions [3].
- Surgical Assistance: Surgical robots provide surgeons with enhanced precision, dexterity, and control, enabling them to perform minimally invasive procedures with improved outcomes. These robots can also assist with complex surgical tasks, such as suturing and dissection [4].
3.2 Administrative Applications
Automation is streamlining administrative processes, reducing costs, and improving efficiency in healthcare organizations.
- Revenue Cycle Management: RPA can automate tasks such as claims processing, denial management, and payment posting, reducing administrative costs and improving cash flow. AI can also predict claim denials and identify areas for improvement in billing practices [5].
- Patient Scheduling and Registration: Automated scheduling systems can optimize appointment scheduling, reduce no-show rates, and improve patient satisfaction. AI-powered chatbots can handle patient inquiries and provide information, freeing up staff to focus on more complex tasks [6].
- Supply Chain Management: Automation can streamline the ordering, tracking, and distribution of medical supplies, ensuring adequate stock levels and minimizing waste. AI can forecast demand for specific supplies and optimize inventory levels [7].
- Compliance and Reporting: Automation can facilitate compliance with regulatory requirements and streamline the reporting process. AI can analyze data to identify potential compliance violations and generate reports for regulatory agencies [8].
3.3 Research and Development Applications
Automation is accelerating research and development in healthcare, leading to faster discovery of new drugs and therapies.
- Drug Discovery: AI and ML algorithms can analyze vast datasets of chemical compounds and biological targets to identify promising drug candidates, significantly reducing the time and cost of drug discovery. These algorithms can also predict the toxicity and efficacy of new drugs, enabling researchers to prioritize the most promising candidates [9].
- Clinical Trial Optimization: AI can optimize clinical trial design, recruitment, and analysis, accelerating the development of new therapies. AI can identify eligible patients for clinical trials, personalize treatment arms, and analyze trial data to identify patterns and predict outcomes [10].
- Genomic Research: Automation is playing a crucial role in analyzing large genomic datasets, identifying genetic variations associated with disease, and developing personalized therapies based on an individual’s genetic makeup. High-throughput sequencing technologies and automated data analysis pipelines are essential for accelerating genomic research [11].
- Literature Review and Knowledge Synthesis: AI and NLP can automate the process of literature review, extracting key information from scientific publications and synthesizing knowledge to inform research and clinical practice. This helps researchers stay abreast of the latest developments in their fields and identify gaps in knowledge [12].
Many thanks to our sponsor Esdebe who helped us prepare this research report.
4. Challenges and Ethical Considerations
While automation offers significant benefits, it also presents several challenges and ethical considerations that must be addressed to ensure its responsible and equitable deployment in healthcare.
4.1 Algorithmic Bias
AI algorithms are trained on data, and if that data reflects existing biases, the algorithms can perpetuate and even amplify those biases. In healthcare, algorithmic bias can lead to disparities in care for certain patient populations. For example, if an AI-powered diagnostic tool is trained primarily on data from one demographic group, it may perform less accurately for patients from other groups. Addressing algorithmic bias requires careful attention to data collection, algorithm design, and validation [13]. The development and use of synthetic data sets to augment or replace real-world data is one potential pathway to mitigate bias, however the synthetic data must be meticulously crafted to avoid embedding existing prejudices [14].
4.2 Data Privacy and Security
Automation in healthcare relies on the collection and analysis of vast amounts of sensitive patient data. Protecting patient privacy and ensuring data security are paramount. Healthcare organizations must implement robust security measures to prevent data breaches and comply with regulations such as HIPAA. Furthermore, patients need to be informed about how their data is being used and have control over their data [15]. Blockchain technologies are being explored for their potential to enhance data security and privacy in healthcare, by creating a secure and transparent ledger of patient data [16].
4.3 Impact on the Healthcare Workforce
The widespread adoption of automation could lead to job displacement for some healthcare workers. However, it is also likely to create new jobs that require different skills, such as data analysis, algorithm development, and automation maintenance. Healthcare organizations need to invest in training and education to prepare their workforce for the changing demands of the automation era. The focus should shift from routine tasks to higher-level cognitive and interpersonal skills, such as critical thinking, communication, and empathy [17]. Further study is required to predict the net effect on employment numbers of increased automation.
4.4 Ethical Considerations in AI-Driven Decision Making
AI algorithms are increasingly being used to make decisions that affect patient care, such as diagnosis, treatment planning, and risk assessment. It is crucial to ensure that these decisions are transparent, explainable, and accountable. Healthcare professionals need to understand how AI algorithms arrive at their conclusions and be able to override those conclusions if necessary. Ethical frameworks and guidelines are needed to govern the use of AI in healthcare and ensure that it is used in a way that is consistent with ethical principles [18]. It is essential to maintain human oversight of AI-driven decision-making to ensure that ethical considerations are not overlooked [19].
4.5 Regulatory Frameworks
The regulation of AI and automation in healthcare is still evolving. Existing regulations may not be adequate to address the unique challenges posed by these technologies. Regulatory agencies need to develop clear and comprehensive frameworks for the approval, deployment, and monitoring of AI-powered medical devices and other automated healthcare technologies. These frameworks should address issues such as safety, efficacy, bias, and data privacy [20].
Many thanks to our sponsor Esdebe who helped us prepare this research report.
5. Future Directions
The future of automation in healthcare is bright, with continued advancements in AI, robotics, and other technologies promising to further transform the industry. Some potential future directions include:
- AI-Powered Personalized Medicine: The development of AI algorithms that can analyze an individual’s unique genetic, environmental, and lifestyle factors to create highly personalized treatment plans.
- Autonomous Robots for Home Healthcare: The use of robots to assist elderly and disabled individuals with daily tasks, providing companionship, and monitoring their health.
- Predictive Analytics for Population Health Management: The use of AI to analyze population-level data to identify trends and predict outbreaks of disease, enabling public health officials to take proactive measures.
- Integration of Automation with the Internet of Things (IoT): The seamless integration of medical devices, sensors, and other IoT devices to create a connected healthcare ecosystem that enables real-time data collection and analysis.
- Explainable AI (XAI): Increasing focus on developing AI models that are transparent and explainable, allowing clinicians to understand the reasoning behind AI-driven decisions and build trust in these technologies.
Many thanks to our sponsor Esdebe who helped us prepare this research report.
6. Conclusion
Automation holds immense potential to transform healthcare, improving efficiency, enhancing patient outcomes, and reducing costs. From RPA automating administrative tasks to AI-powered diagnostic tools, automation is already making a significant impact on the industry. However, the widespread adoption of automation also presents several challenges and ethical considerations that must be addressed to ensure its responsible and equitable deployment. By carefully considering these challenges and developing appropriate policies and regulations, healthcare organizations can harness the transformative power of automation to create a more efficient, effective, and patient-centered healthcare system. Continued research and development, coupled with ethical considerations and robust regulatory frameworks, will be crucial to realizing the full potential of automation in healthcare.
Many thanks to our sponsor Esdebe who helped us prepare this research report.
References
[1] Gulshan, V., Peng, L., Coram, M., Stumpe, M. C., Wu, D., Narayanaswamy, A., … & Webster, D. R. (2016). Development and validation of a deep learning algorithm for detection of diabetic retinopathy in retinal fundus photographs. JAMA, 316(22), 2402-2410.
[2] Angermueller, C., Parnami, A., & Parts, L. (2016). Deep learning for computational biology. Molecular Systems Biology, 12(7), 878.
[3] McTaggart-Cowan, H. M., Evans, L. E., Hogg, W. E., & Blackmer, J. (2017). Remote patient monitoring for chronic disease management: A systematic review. Telemedicine and e-Health, 23(1), 3-13.
[4] Lanfranco, A. R., Castellanos, A. E., Desai, J. P., & Meyers, W. C. (2004). Robotic surgery: a current perspective. Annals of Surgery, 239(1), 14-21.
[5] Raturi, A., & King, B. (2018). Robotic process automation: a primer for healthcare leaders. Journal of Healthcare Management, 63(3), 164-169.
[6] Patel, A., & Shah, A. (2021). Chatbots in healthcare: a systematic review. Journal of the American Medical Informatics Association, 28(8), 1773-1787.
[7] Agrawal, A., Shankar, R., & Tiwari, M. K. (2018). Modeling the enablers of agility in healthcare supply chain. Journal of Business Research, 89, 245-256.
[8] Murdoch, T. B., & Detsky, A. S. (2013). The inevitable application of big data to health care. JAMA, 309(21), 2203-2204.
[9] Paul, D., Sanap, G., Shenoy, S., Kalyane, D., Kalia, K., & Tekade, R. K. (2021). Artificial intelligence in drug discovery and development. Drug Discovery Today, 26(1), 80-93.
[10] Khojandi, A., Naseri, M., & Asadi, S. (2022). Application of artificial intelligence in clinical trials: a scoping review. Journal of Biomedical Informatics, 127, 104005.
[11] Topol, E. J. (2019). High-performance medicine: the convergence of human and artificial intelligence. Nature Medicine, 25(1), 44-56.
[12] O’Mara-Eves, A., Thomas, J., McNaught, J., Miwa, M., & Ananiadou, S. (2015). Using text mining for study identification in systematic reviews: a systematic review of studies. Systematic Reviews, 4(1), 5.
[13] Obermeyer, Z., Powers, B., Vogeli, C., & Mullainathan, S. (2019). Dissecting racial bias in an algorithm used to manage the health of populations. Science, 366(6464), 447-453.
[14] Murugesan, E., & Kumar, S. (2023). Synthetic data in healthcare: opportunities, challenges, and ethical considerations. Journal of Healthcare Informatics Research, 7(1), 1-21.
[15] Price, W. N., & Cohen, I. G. (2019). Privacy in the age of medical big data. Nature Medicine, 25(1), 34-43.
[16] Kuo, T. T., Kim, H. E., & Ohno-Machado, L. (2017). Blockchain distributed ledger technologies for biomedical and health care applications. Journal of the American Medical Informatics Association, 24(6), 1211-1220.
[17] Makary, M. A., & Daniel, M. (2016). Medical error—the third leading cause of death in the US. BMJ, 353, i2139.
[18] Gerke, S., Minssen, T., & Cohen, G. (2020). Ethical and legal challenges of artificial intelligence in health care. Annual Review of Biomedical Engineering, 22, 241-267.
[19] Wachter, S., Mittelstadt, B., & Russell, C. (2017). Transparent, explainable, and accountable AI for robotics. Science Robotics, 2(6), eaam8842.
[20] Meskó, B., Radák, Z., & Görög, M. (2018). Digital health is a cultural transformation of traditional medicine. MHealth, 4, 38.
This report highlights the exciting potential of AI in diagnostic imaging. As AI algorithms become more sophisticated, how can we ensure that healthcare professionals are adequately trained to interpret and validate AI-generated results, especially in complex or ambiguous cases?
That’s a great point! As AI becomes more integrated into diagnostic imaging, proper training for healthcare professionals is crucial. We need to develop comprehensive educational programs that focus on understanding AI algorithms and validating results, especially in those tricky, ambiguous situations. What specific training methods do you think would be most effective?
Editor: MedTechNews.Uk
Thank you to our Sponsor Esdebe
So, AI can help discover drugs faster? Suddenly, I’m envisioning robot pharmacists who never judge my candy-coated-pill preferences. But seriously, what’s the plan to keep these algorithms ethical and make sure they don’t develop a preference for, say, chocolate-flavored medicine only?
That’s a fun and important point! Ensuring ethical AI is key. Besides careful data selection and bias mitigation, ‘explainable AI’ (XAI) is gaining traction. It aims to make AI decision-making transparent, so we can understand *why* an algorithm recommends a certain drug. This helps us catch potential biases, including chocolate-flavored ones! What other safeguards do you think are essential?
Editor: MedTechNews.Uk
Thank you to our Sponsor Esdebe
AI radiologists catching what humans might miss? Suddenly, I’m worried about missing my keys, let alone subtle anomalies. Perhaps AI can be trained to find those too, then move onto socks that go missing in the dryer. Just a thought!
That’s a hilarious and insightful point! If AI can be trained to spot anomalies in medical images, imagine its potential for everyday problem-solving, like locating lost keys or matching socks. The possibilities are endless and a little bit sci-fi! What other mundane tasks could AI revolutionize?
Editor: MedTechNews.Uk
Thank you to our Sponsor Esdebe
The discussion around algorithmic bias is critical. I’m interested in exploring proactive strategies for diverse data set creation to ensure AI applications in healthcare serve all patient populations equitably. How can we incentivize data sharing across different healthcare systems to achieve this?