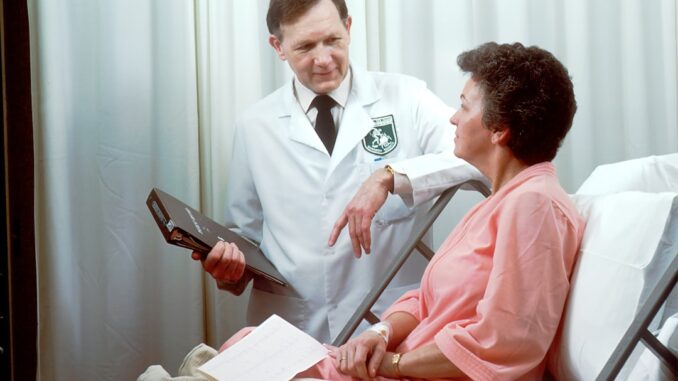
Summary
OpenAI introduces HealthBench, a benchmark designed to evaluate the safety and performance of Large Language Models (LLMs) in healthcare. Developed with input from physicians globally, HealthBench utilizes realistic conversations and detailed rubrics to measure LLMs against real-world medical scenarios. This comprehensive evaluation aims to ensure AI advancements truly benefit human health.
Healthcare data growth can be overwhelming scale effortlessly with TrueNAS by Esdebe.
** Main Story**
OpenAI Unveils HealthBench: A New Era for Healthcare AI
OpenAI recently launched HealthBench, a groundbreaking benchmark designed to rigorously evaluate the performance and safety of LLMs in healthcare. This innovative tool signifies a pivotal step towards responsible AI integration in medicine, addressing crucial safety concerns and paving the way for transformative advancements. HealthBench moves beyond traditional exam-style tests and delves into realistic health scenarios, prioritizing real-world applicability and physician judgment.
Evaluating LLMs in Realistic Medical Scenarios
HealthBench comprises 5,000 multi-turn conversations, simulating real-world interactions between AI models and patients or clinicians. These conversations, crafted through synthetic generation and adversarial testing, cover diverse medical specialties, languages, and contexts. The model’s task is to provide the optimal response to each user message. A team of 262 physicians from 60 countries, representing 26 medical specialties and 49 languages, collaborated to build this benchmark.
Physician-Driven Rubrics for Comprehensive Assessment
For each conversation, a physician-created rubric guides the evaluation of model responses. These rubrics incorporate 48,562 unique criteria, outlining ideal response elements and potential pitfalls, such as the inclusion of specific facts or avoidance of overly technical jargon. Each criterion carries a weighted point value, reflecting its clinical significance as judged by physicians. The model’s responses undergo evaluation using GPT-4.1, which determines if each rubric criterion is met. This generates an overall score, compared against the maximum achievable score, providing a clear measure of the model’s performance.
HealthBench’s Seven Key Themes
HealthBench is structured around seven key themes:
- Expertise-tailored communication: Assesses the model’s ability to adapt communication style to the expertise level of the user.
- Response depth: Evaluates the comprehensiveness and informativeness of responses.
- Emergency referrals: Tests appropriate identification and referral for emergency situations.
- Health data tasks: Measures ability to analyze and process health data effectively.
- Global health: Evaluates model performance across various cultural and geographical health contexts.
- Responding under uncertainty: Assesses appropriate handling of uncertain or ambiguous medical information.
- Context seeking: Evaluates ability to gather necessary contextual information for effective responses.
The Promise of HealthBench for Advancing Healthcare AI
HealthBench offers a significant advancement in evaluating AI models for healthcare applications, placing a strong emphasis on safety and real-world performance. This detailed assessment tool not only measures the current capabilities of LLMs but also guides future development, ensuring progress toward AI systems that genuinely benefit human health. As AI continues to evolve, HealthBench will serve as a vital tool for navigating the complex landscape of healthcare, ultimately transforming the practice of medicine and improving patient care. OpenAI’s commitment to this rigorous evaluation process underscores the importance of responsible AI development and deployment, ensuring that AI advancements translate into tangible improvements in human health. While OpenAI’s model-based grading raises concerns about objectivity, HealthBench’s focus on real-world clinical scenarios marks a significant step toward more relevant AI evaluation in healthcare. This initiative addresses the critical need for rigorous testing of AI’s abilities to reason, explain, and communicate effectively within the complex context of medical practice. AI holds enormous potential for improving diagnostics, accelerating drug discovery, and personalizing treatments. However, challenges remain, such as data privacy, algorithmic bias, and the need for robust clinical validation. Continued research and development are essential to harnessing AI’s transformative power while mitigating potential risks. As of May 18, 2025, HealthBench represents a critical step toward realizing the promise of AI in medicine, offering a robust framework for evaluating and improving these powerful tools.
Given HealthBench’s focus on expertise-tailored communication, how effectively does it assess an LLM’s ability to navigate the emotional and psychological nuances inherent in patient interactions, beyond just factual accuracy?