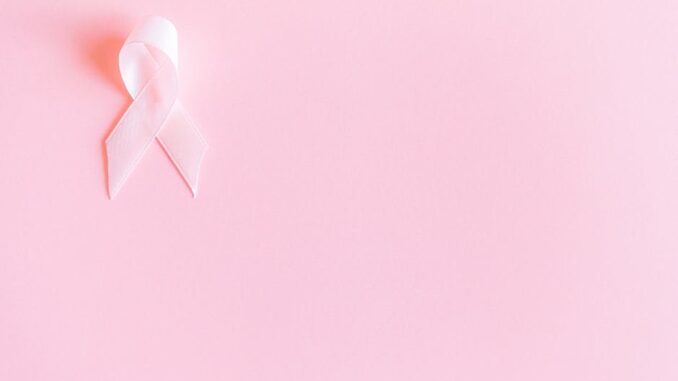
Summary
Stanford Medicine has developed a groundbreaking AI model called MUSK that accurately predicts cancer prognoses and treatment responses by combining medical image data with textual information. This innovative approach surpasses traditional methods, paving the way for personalized cancer care. MUSK’s ability to integrate diverse data types and its high accuracy signify a substantial advancement in precision medicine for oncology.
Healthcare data growth can be overwhelming scale effortlessly with TrueNAS by Esdebe.
Main Story
Okay, so, get this. Researchers at Stanford Medicine have developed this amazing AI model, they’re calling it MUSK. And honestly, it could be a total game-changer for cancer treatment. Think about it, a tool that can actually predict how a patient’s cancer will progress and how they’ll respond to different treatments? That’s pretty huge.
Now, what makes MUSK different? Well, most traditional methods rely on either image data (like scans) or text data (like patient notes). MUSK, on the other hand, is multimodal. It combines both!
MUSK: A Whole New Approach
This means it looks at X-rays, CT scans, MRIs and all the written info like pathology reports and physician notes. It’s like giving the AI a full picture, not just a piece of the puzzle. I mean, how often do we look at things in isolation? Usually you need multiple sources to really understand something right? This allows the AI to pick up on subtle connections and patterns that might otherwise be missed. It was trained on a massive amount of data too, like 50 million images and over a billion pieces of text. That’s a lot to process.
And get this, it can even learn from what they call ‘unpaired’ data! Most AI tools need specifically linked image/report pairs, but MUSK? It can learn from just about everything. So, it can process a much wider variety of data, and learn at a faster rate. Which is really important. I mean, think about the limitations of labeled data versus a sea of available information.
The Impact on Cancer Treatment
What’s the real-world impact, though? That’s the question right? Well, in studies, MUSK beat out the standard models, showing better accuracy in predicting outcomes, and also which patients would respond best to things like immunotherapy. For example, they found a 77% accuracy rate for lung cancer patients, compared to 61% with older methods. That’s a huge jump. This could mean fewer ineffective treatments, less wasted time and resources and ultimately, better patient outcomes.
Also, its ability to predict survival rates? That’s invaluable. Clinicians can use that to tailor treatment to each individual’s needs, and give much better informed prognoses. This is such an important part of care, and often it’s not precise enough. That’s why I’m so impressed. I think we all would be. This level of precision can make such a big difference in patient’s lives, and let’s be honest, give them a bit of hope.
More Than Just Prognosis
While it’s focused on cancer right now, the technology itself has really broad potential. The same way we use MUSK to analyze the data and make predictions about a patient’s treatment path, the underlying framework for analysis of data could be used in a variety of fields. This could open the door to AI tools for things like diagnosing other diseases, personalizing drug development and all kinds of cool stuff.
I mean, think about how this technology can revolutionize care, its not just about the accuracy, it’s about how we could use AI to reshape everything.
A Glimpse of the Future
It’s clear that we’re really moving into a new era of AI in medicine. Tools like MUSK are becoming increasingly sophisticated, and I genuinely believe this shift will mean a more personalized and effective approach to healthcare. The ultimate goal is to make healthcare better for everyone, right? And technology like this could help move us towards that, even making universal coverage more attainable, which I think is something everyone can get behind. It’s a pretty exciting time for medicine, and honestly, kind of a relief to see advancements that offer so much promise.
The ability to learn from ‘unpaired’ data is intriguing. Could this approach be extended to other diagnostic areas where data sets are often incomplete or inconsistently linked?
That’s a great point! The ability to leverage ‘unpaired’ data is a key strength of MUSK. Extending this to other diagnostic areas with incomplete datasets is definitely something that could have massive implications, potentially transforming how we approach diagnosis beyond just cancer.
Editor: MedTechNews.Uk
Thank you to our Sponsor Esdebe – https://esdebe.com
So, 50 million images *and* a billion text bits? Seems like even AI has to deal with messy data, not just the rest of us. Wonder what kind of algorithm they use to sift through that much digital clutter.
That’s a great observation! The sheer volume of data is certainly a challenge, and it highlights the sophistication of the algorithms involved. It’s fascinating how AI can manage these massive datasets to identify meaningful patterns that we might miss. The article mentioned that MUSK could leverage ‘unpaired’ data to learn faster. I wonder how it would cope with data that was not only ‘unpaired’ but also corrupted.
Editor: MedTechNews.Uk
Thank you to our Sponsor Esdebe – https://esdebe.com
So, MUSK eats up 50 million images AND a billion text bits, eh? I’m just picturing it now, a digital data monster on an all-you-can-eat buffet. Does it ever get indigestion?
That’s a fun analogy! It’s fascinating how AI can process such a vast amount of information. I also wonder about the challenges of managing this data; the sheer scale must present a number of interesting logistical problems. What tools would they use to organize all of that data?
Editor: MedTechNews.Uk
Thank you to our Sponsor Esdebe – https://esdebe.com
The multimodal approach is interesting, combining both image and text data. How does the system weigh the relative importance of these different data types when making predictions?