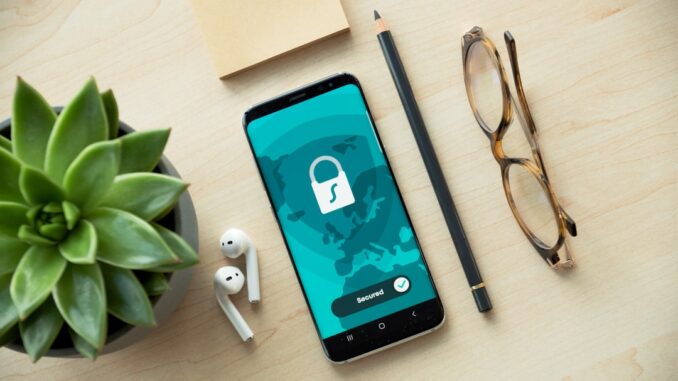
Summary
AI’s potential in medicine is immense, but flawed data can lead to harmful outcomes. Ensuring data quality, mitigating bias, and prioritizing patient privacy are crucial for responsible AI development. By addressing these challenges, we can harness AI’s power while safeguarding patient well-being.
Safeguard patient information with TrueNASs self-healing data technology.
** Main Story**
AI is changing medical technology fast, offering some amazing possibilities in diagnostics, personalized treatments, and just making things more efficient overall. But here’s the thing: if the data it uses isn’t up to par, well, that’s where things can get a little dicey.
Think about it, the effectiveness and safety of AI in healthcare? It really all boils down to the data. This article? We’re diving into the potential problems when AI in medicine gets trained on data that’s either not good enough or, even worse, biased. So, let’s get into why keeping data honest and ethical is so important.
The Perils of Poor Data
AI algorithms, they’re like sponges, right? They learn from whatever you feed them. And if that “food” is incomplete, inaccurate, or has a slant to it, the AI is going to soak up all those flaws and make them even bigger. In healthcare, that can cause some real problems.
- Misdiagnosis and Mistreatment: Imagine an AI trained on incomplete data. It might misdiagnose someone, leading to the wrong treatment, maybe even something harmful. For example, if an AI is mainly trained on data from one group of people, it might not work well when it looks at data from people of different backgrounds. I remember one time I was working on an AI project, and we quickly realized that the training data was overwhelmingly from one geographic location. We had to spend a lot of time and resources to find data from other areas to make sure it would work more effectively.
- Perpetuation of Bias: Here’s another scary one. Biased data can lead to AI systems that discriminate against certain people. If an AI is trained on data that shows differences in healthcare access from the past, it might keep those unfair patterns going. You really don’t want an AI to keep existing prejudices going; that defeats the point!
- Erosion of Trust: If AI systems keep giving unreliable or biased results because of bad data, people, both patients and doctors, might lose faith in it. And if that happens, it will be harder to use AI and get all the good things it can offer.
The Importance of Data Integrity in Healthcare AI
So, how do we avoid these problems? Well, we need to take some key steps.
- Data Diversity and Representativeness: First off, AI systems need to be trained on datasets that are diverse and accurately show the patient populations they’ll be working with. That means data from different groups of people, ages, and health conditions.
- Data Quality Control: We also need really strict quality control to make sure the data is accurate, complete, and consistent. That means regular data checks, validation steps, and ways to catch errors. And honestly, there’s no cutting corners, if the data doesn’t have integrity, there’s no way the system can function properly.
- Bias Detection and Mitigation: Researchers and developers need to actively look for and fix biases in AI algorithms and datasets. One way to do this is by using fairness-aware machine learning and including diverse viewpoints in the development process. It’s also helpful to test the algorithm on many diverse datasets to see where it fails.
Ethical Considerations
But data integrity isn’t the only thing. Ethics are huge when it comes to AI in healthcare.
- Transparency and Explainability: AI systems should be open about how they make decisions. Doctors should be able to understand how the AI arrived at a diagnosis or treatment suggestion. This builds trust and helps doctors catch and fix any errors.
- Patient Privacy and Data Security: When using patient data for AI development, we have to follow strict privacy rules and security measures. Patients should have control over their data and know exactly how it’s being used. It goes without saying that you can’t put profit before people’s private information, that’s a recipe for disaster.
- Human Oversight and Collaboration: Remember, AI should help doctors, not replace them. The final call should always be with a qualified healthcare professional who can use the AI’s recommendations along with their own knowledge and the patient’s input. I think most people in the industry agree on that fact, but, it bears repeating.
Conclusion
AI has huge potential to change healthcare for the better. But to make that happen, we need to develop and use it responsibly. By making data integrity a priority, fixing biases, and sticking to ethical principles, we can use AI to improve patient outcomes while protecting their well-being and trust. As AI keeps developing, we need researchers, developers, doctors, and policymakers to keep working together to make sure this powerful technology is used safely and fairly. Isn’t that the least we can do?
Be the first to comment