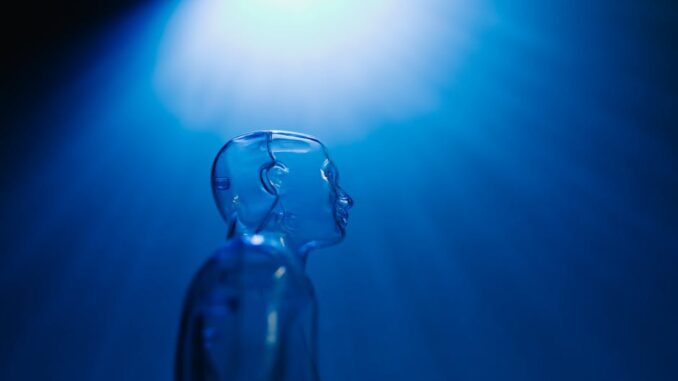
Summary
This article explores the groundbreaking use of AI in detecting schizophrenia, focusing on machine learning’s ability to analyze various data sources for early diagnosis and personalized treatment. It also discusses the potential of AI to revolutionize mental healthcare, improving patient outcomes and reducing the global burden of this debilitating disease. This technology offers hope for earlier intervention and more effective treatment strategies for schizophrenia.
** Main Story**
Schizophrenia is a tough nut to crack. This severe mental illness affects millions globally, presenting real challenges in both diagnosis and treatment. Traditionally, we’ve relied on subjective symptom assessment, which, let’s be honest, can lead to delays in getting people the help they need. But things are changing, and for the better. Artificial intelligence, specifically machine learning, is stepping up to the plate, potentially revolutionizing how we detect this complex condition.
Machine Learning: Decoding Schizophrenia
So, how is AI making a difference? Well, machine learning algorithms are fantastic at spotting patterns in massive datasets. That makes them perfectly suited for untangling the complexities of schizophrenia. Researchers are tapping into ML to sift through all sorts of data:
-
Brain Scans: Think fMRI and EEG data. Studies have shown that ML can predict early schizophrenia symptoms in high-risk individuals with impressive accuracy. It can even pinpoint those with strong schizotypal personality traits. This is a big deal because early intervention can seriously lessen the long-term impact of the illness. It’s about getting ahead of the curve. I remember reading a study a while back where they managed to predict, with surprising accuracy, which teenagers were likely to develop symptoms later in life. Blew my mind.
-
Social Media Content: I know, it sounds a bit ‘Big Brother,’ but hear me out. ML algorithms can now detect linguistic markers of schizophrenia in social media posts. By analyzing language patterns and the topics people are discussing, researchers can potentially identify individuals who might be showing signs of the disorder. This could open up new avenues for outreach and support. Obviously there are privacy concerns here but the technology is progressing.
-
Speech Patterns: Ever notice how someone’s speech changes when they’re stressed or unwell? AI does, too. Analyzing speech patterns via ML is another promising approach. AI models can distinguish between the speech of individuals with schizophrenia and healthy controls with high accuracy. They can pick up on subtle changes in speech that a human clinician might miss. It’s all about those nuanced details.
-
Genetic and Epigenetic Data: This is where it gets really interesting. ML models can analyze genetic and epigenetic information to predict someone’s likelihood of developing schizophrenia. This offers valuable insights into the underlying biological mechanisms of the disease and could pave the way for more personalized medicine. Imagine being able to tailor treatment based on someone’s genetic makeup. That said, it’s still early days, but the potential is there.
Explainable AI: Bringing Clarity to the Black Box
One of the biggest concerns with AI, especially in healthcare, is the ‘black box’ problem. How do we know why an AI made a certain decision? That’s where explainable AI (XAI) comes in. XAI methods, such as LIME and SHAP, provide insights into the decision-making processes of ML models. They help clinicians understand why a particular diagnosis was made. This transparency is essential for building trust in AI-driven tools and ensuring responsible implementation in clinical settings. If you can’t explain it, how can you trust it, right?
The Future is Now…Almost
The future looks bright for AI in schizophrenia care. The potential impact is significant, not only for diagnosis but also for treatment and ongoing management:
-
Early Diagnosis and Intervention: Like I said, AI-powered tools could identify at-risk individuals before symptoms become debilitating, allowing for timely intervention and potentially changing the course of the disease. Prevention is better than cure, always.
-
Personalized Treatment: By analyzing individual patient data, AI can assist clinicians in tailoring treatment plans to specific needs and optimizing treatment outcomes. Think about it: no more one-size-fits-all approaches.
-
Improved Prognosis: AI can predict the trajectory of the illness, helping clinicians and patients make informed decisions about long-term care. It’s about empowering people with information.
-
Reduced Healthcare Burden: Automating administrative tasks and streamlining diagnostic processes can free up clinicians’ time, allowing them to focus on patient-centered care. And let’s face it, the healthcare system could use all the help it can get.
A Word of Caution
Now, it’s not all sunshine and roses. There are challenges we need to address:
-
Data Bias: ML models are only as good as the data they learn from. If the data is biased, the results will be, too. Ensuring diverse and representative datasets is critical. We don’t want to perpetuate existing inequalities.
-
Ethical Implications: The use of AI in mental healthcare raises ethical questions about privacy, autonomy, and the potential for stigmatization. Strict guidelines and regulations are essential to protect individuals’ rights and dignity. It can become a very slippery slope.
-
Clinical Integration: Successfully integrating AI tools into clinical workflows requires addressing practical considerations like data interoperability, user training, and regulatory approval. You can’t just drop an AI tool into a hospital and expect everyone to know how to use it. It needs to be seamless, intuitive, and compliant.
Ultimately, as AI technology continues to evolve, its role in schizophrenia detection and treatment will undoubtedly expand. By embracing the power of machine learning while addressing the inherent challenges, we have a real opportunity to revolutionize mental healthcare and improve the lives of millions affected by this condition. It’s not a magic bullet, but it’s a powerful tool that, if used responsibly, could make a world of difference. And isn’t that what we’re all striving for?
The use of social media content is intriguing, but how can we ensure the algorithms accurately differentiate between genuine indicators of schizophrenia and the typical expressions of online discourse or diverse cultural communication styles?
That’s a great point! Ensuring accuracy in differentiating indicators versus online discourse is crucial. Algorithms need to be trained on diverse datasets, accounting for cultural nuances and communication styles. Ongoing evaluation and refinement are key to mitigating false positives. This also highlights the importance of ethical considerations and transparency in AI development. Thanks for raising this important question!
Editor: MedTechNews.Uk
Thank you to our Sponsor Esdebe