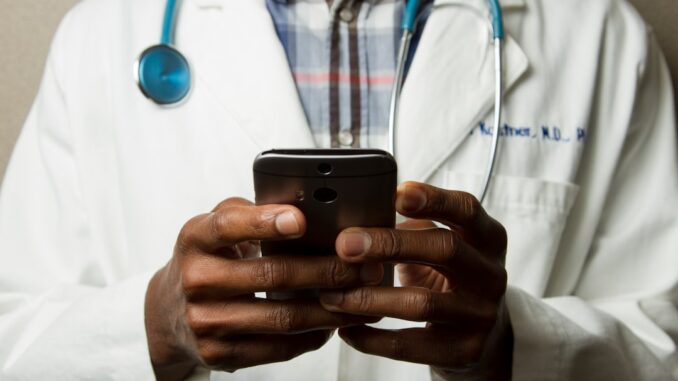
Summary
This article explores two groundbreaking AI advancements poised to reshape healthcare and scientific research. The first involves generative AI models accelerating the discovery of novel materials, while the second focuses on enhancing medical imaging analysis for faster diagnoses and improved patient care. These breakthroughs highlight the transformative potential of AI in diverse fields.
Healthcare data growth can be overwhelming scale effortlessly with TrueNAS by Esdebe.
** Main Story**
AI is really shaking things up these days, isn’t it? And when you look at what’s happening in healthcare and science, it’s just…mind-blowing. We’re not just talking about incremental improvements, we’re talking about potential paradigm shifts. Two recent advancements, in particular, are worth diving into.
Speeding Up Materials Discovery with Generative AI
So, first up is generative AI. And I know, ‘generative AI’ sounds like something straight out of a sci-fi film. However, it is changing the way researchers are discovering new materials. Think about it: traditionally, finding the right material for a specific job – medicine, energy, electronics – was a slow, painstaking process of trial and error. But with these large-scale AI models, which are popping up everywhere, we can basically ‘design’ materials with specific properties on a computer. This is thanks to collaborations between tech companies like Microsoft, different Universities and the private sector.
For instance, imagine needing a new type of battery that’s more efficient and lighter. Instead of spending years experimenting in the lab, you could use generative AI to simulate thousands of different material combinations and predict which ones are most likely to work. It’s like having a super-powered crystal ball, but for materials science. This approach helps in exploring a vast design space, something which was simply not possible earlier.
Revolutionizing Medical Imaging Analysis
Now, let’s switch gears to medical imaging. Medical Imaging is a field that has been slow to innovate in recent years, but the advent of AI is changing that. I remember, talking to my friend who’s a radiologist, about how much time they spend poring over images, looking for subtle anomalies that could indicate disease. And it’s a lot.
That’s where the second breakthrough comes in: a collaboration between Mayo Clinic and Microsoft Research, which produced something called RAD-DINO. Essentially, RAD-DINO is this multimodal foundation model that combines text and images to analyze radiology results faster and more accurately. It’s a bit like giving radiologists a second pair of eyes. Not only can it identify anatomical matches between chest X-rays of different patients, but it can also detect changes from previous images. This allows for earlier diagnoses and improved patient care. It might even generate reports automatically.
The initial focus is on automatic report generation, evaluating tube and line placements, and identifying changes from previous images. Early results show promise for better patient outcomes and streamlined healthcare workflows, I feel like, in the long run, its really going to change the way hospitals operate.
The Bigger Picture: What Does it All Mean?
These two AI breakthroughs really underscore the incredible potential of AI to transform various fields. While I think that the potential is there to improve human health and our knowledge of Science, we should be cautious about deploying these tools. The ability to accelerate materials discovery and enhance medical imaging analysis…it’s a game-changer.
And as AI technology continues to evolve, well, who knows what’s next? We can probably expect even more groundbreaking applications that will reshape healthcare and scientific research in the years ahead. I think that ultimately we must approach the development and deployment of these tools responsibly. It’s up to us to make sure that these advances are used to benefit everyone, and not just a select few.
The RAD-DINO project’s multimodal approach to radiology is fascinating. How might combining other data types, like genomic information or patient history, further enhance diagnostic accuracy and personalize treatment plans in the future?
That’s a great question! Integrating genomic data with RAD-DINO could provide a deeper understanding of disease predispositions, leading to more precise diagnoses. Patient history could then contextualize these findings, creating truly personalized treatment strategies. Exciting possibilities!
Editor: MedTechNews.Uk
Thank you to our Sponsor Esdebe
Regarding generative AI in materials discovery, what validation methods are being employed to ensure the predicted material properties translate effectively from simulation to real-world applications, especially considering the complexities of manufacturing and scaling?
That’s a really important question! Validation is critical. Currently, many researchers are using a combination of experimental validation and advanced simulation techniques to bridge the gap between predicted and real-world properties. Further research into uncertainty quantification will improve the simulations. These validation strategies are vital for ensuring the successful application of AI-designed materials.
Editor: MedTechNews.Uk
Thank you to our Sponsor Esdebe
The RAD-DINO project’s ability to detect changes from previous images is particularly exciting. Could this approach be expanded to other medical imaging modalities beyond radiology, such as MRI or CT scans, to monitor disease progression more comprehensively?