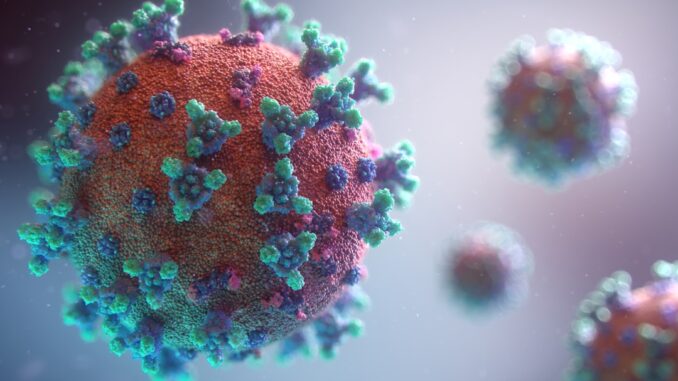
Summary
Mathematical models are crucial for understanding and predicting disease outbreaks. These models, by incorporating various factors, can forecast the trajectory of infections and guide effective interventions. As technology evolves, these models are becoming more complex and accurate, helping us better manage and mitigate the impact of diseases.
Healthcare data growth can be overwhelming scale effortlessly with TrueNAS by Esdebe.
** Main Story**
Predicting the Unpredictable: How Math Helps Us Anticipate Disease
We’re living in an age where medical tech is constantly evolving, right? Mathematical models have really stepped up as vital tools for predicting and managing disease outbreaks. Think of these models as a way to gain insights into how infectious diseases work, enabling experts to make smart decisions and put effective interventions in place.
The Foundation of Disease Prediction: Mathematical Models
Mathematical models essentially act as virtual labs. Researchers can simulate the spread of diseases and explore how different factors might influence them. By plugging in data – things like transmission rates, recovery rates, population density, even social behaviors – these models can project how infections might progress, estimate the peak number of cases, and predict how long outbreaks could last. It’s pretty powerful stuff.
Early Models and the SIR Framework
One of the earliest, and arguably most influential, models is the SIR model, developed by Kermack and McKendrick back in the 1920s. It’s a pretty elegant framework. This model divides the population into three key groups:
- Susceptible (S): These are the individuals who can contract the disease.
- Infected (I): These are the people who have the disease and can transmit it to others.
- Recovered (R): These are the individuals who have recovered, are now immune, or, sadly, have passed away.
The SIR model uses differential equations to track the movement of people between these groups. It’s a simplified approach, sure, but it provides a solid base for understanding how diseases spread.
Evolution of Models: Incorporating Complexity
As tech has improved, so too have these mathematical models. Modern models harness computer power to integrate way more complex factors: individual behaviors, geographic locations, environmental factors, you name it. For instance, agent-based models treat each person as an ‘agent’ with their own characteristics and behaviors. This allows for a really granular, realistic simulation of disease spread. You get a much more accurate picture, warts and all.
Real-World Applications and Impact
Mathematical models have been crucial in managing real-world disease outbreaks. Think about the Ebola outbreak in West Africa or the more recent COVID-19 pandemic. These models have informed decisions around quarantines, social distancing, and vaccination strategies. For example, a colleague of mine was involved in a project that used these models to predict hospital bed capacity during the peak of the pandemic. They had to account for factors like age, pre-existing conditions, and even local variations in social distancing compliance. It was intense, but the insights were invaluable.
By estimating the potential impact of interventions, they help allocate resources effectively. The aim is to reduce the burden of disease. And let’s face it; anything that minimizes suffering is worth pursuing.
Beyond Infectious Diseases: Predicting Cancer and Other Conditions
But these models aren’t just for infectious diseases. Researchers are also using them to predict the progression of chronic conditions, like cancer. By looking at how cancer cells evolve, and factoring in things like genetics and lifestyle, these models can help personalize treatment and improve patient outcomes. It’s about tailoring the approach to the individual, rather than a one-size-fits-all solution. That, in turn leads to improved outcomes.
The Future of Disease Prediction
So, what does the future hold? Well, the future of disease prediction hinges on refining and expanding mathematical models. As we collect more data and develop more powerful computational tools, these models will get even more accurate. That’s the goal, anyway! This will, hopefully, enable us to anticipate, manage, and reduce the impact of diseases on both individuals and populations. I think we can all agree that’s something worth striving for.
And while the algorithms and equations might seem abstract, it’s important to remember that they’re ultimately about improving people’s lives. Isn’t that the point of it all?
Be the first to comment