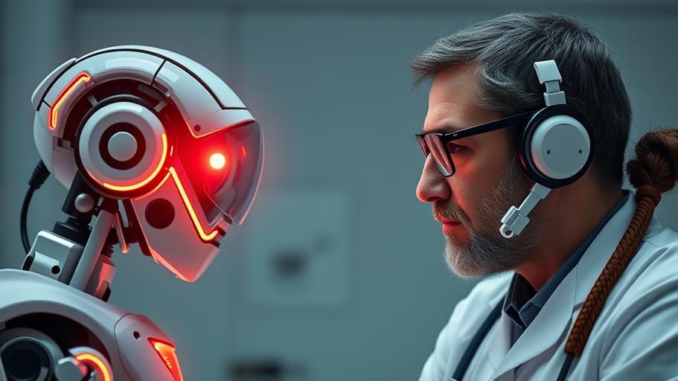
Summary
UCHealth is tackling the pervasive issue of patient falls, a leading cause of injury and reduced independence, especially among older adults. Using AI-powered risk modeling integrated with their EHR system, UCHealth provides care teams with patient-specific fall prevention strategies. This proactive approach improves patient safety and reduces alarm fatigue among clinicians.
** Main Story**
UCHealth Combats Inpatient Falls with AI-Enhanced Risk Modeling
Falls are a significant concern for hospitalized patients, often leading to injuries, prolonged stays, and decreased independence. UCHealth, a leading healthcare system, is tackling this challenge head-on with an innovative approach: AI-enhanced risk modeling. This technology empowers care teams to proactively prevent falls by providing patient-specific interventions.
AI-Powered Predictions and Personalized Interventions
UCHealth’s system analyzes various data points, including patient mobility data, behavioral health indicators, and other risk factors, to predict the likelihood of an inpatient fall resulting in injury. This information integrates directly into their Epic electronic health record system through cloud-based tools. Rather than relying on generic fall precautions, which can disrupt workflows and lead to alarm fatigue among clinicians, the AI model generates a personalized risk profile for each patient. This profile then informs a set of tailored interventions, ensuring that high-risk individuals receive the appropriate level of attention and resources.
Three-Tiered Risk Classification for Actionable Insights
The AI model employs a three-tiered risk classification system: Highest Risk, Elevated Risk, and Universal Risk. This nuanced approach provides clinicians with more actionable insights than a simple high/low risk indicator. For each risk level, the system recommends specific precautions, such as bed alarms, gait belts, and closer nursing station placement. This ensures that staff knows precisely what actions to take for each patient, promoting consistency and efficiency in fall prevention efforts.
Implementation Challenges and Solutions
Integrating the AI model into existing clinical workflows presented several challenges. Initially, simply displaying a numeric risk score proved insufficient. Clinicians needed to understand the reasoning behind the risk assessment and the recommended precautions. To address this, UCHealth redesigned the user interface to provide clear explanations and actionable guidance. The system now displays not only the risk level but also the factors contributing to that assessment and the specific interventions recommended. This transparency ensures that clinicians can effectively utilize the AI-generated insights to improve patient care. Furthermore, standardizing the precautions for each risk level was crucial. By providing a clear protocol for each category, UCHealth ensured consistency and clarity for all staff members.
Conclusion: A Proactive Approach to Patient Safety
UCHealth’s AI-enhanced risk modeling represents a significant advancement in fall prevention. By leveraging data and advanced analytics, the system provides personalized interventions that improve patient safety and reduce alarm fatigue among clinicians. As healthcare systems continue to seek innovative solutions to enhance patient care, UCHealth’s approach serves as a valuable example of how AI can transform risk management and create a safer environment for all. Falls are a major concern, but through the innovative use of technology, UCHealth is taking significant steps to mitigate their impact and improve patient outcomes.
AI predicting falls? Does this mean we’ll soon have robots preemptively yelling, “Timber!” before Grandma even thinks about standing? In all seriousness, how’s the AI handling those patients who *insist* they’re fine right before they aren’t?
That’s a great question! The challenge with patients who are in denial is definitely one we’re working on. The AI flags the risk, but the care team’s judgment is crucial to building trust and encouraging compliance with preventative measures. It’s a human-AI partnership!
Editor: MedTechNews.Uk
Thank you to our Sponsor Esdebe
The three-tiered risk classification seems particularly effective. Do you have any data on how the “Elevated Risk” category impacts resource allocation compared to a binary risk assessment model?
That’s a great point! We found the three-tiered system allows for more targeted resource allocation. The “Elevated Risk” group receives interventions that wouldn’t be feasible for everyone but are crucial in preventing falls. We’re still gathering data, but initial results show a significant improvement in fall rates within this specific cohort compared to previous binary approaches.
Editor: MedTechNews.Uk
Thank you to our Sponsor Esdebe
AI improving patient safety and reducing alarm fatigue? Finally, machines doing something useful besides suggesting what I should buy next. Any thoughts on expanding this tech to predict and prevent *other* hospital mishaps, like rogue bedpan incidents? Just curious!
That’s a fun thought! Exploring applications beyond falls is definitely on our radar. We’re looking into how predictive models could improve resource allocation and prevent other common hospital incidents. Your comment highlights the broader potential of AI in patient safety, and we appreciate the innovative idea!
Editor: MedTechNews.Uk
Thank you to our Sponsor Esdebe
So, we’re standardizing fall precautions now? I assume this means interpretive dance classes for patients flagged as “Highest Risk” are off the table? Seriously though, standardization plus AI – interesting combo. Is there any consideration for regional differences in hospital layouts and resources when applying this model across the UCHealth system?
That’s a really interesting point about regional differences! Absolutely, the model needs to be adaptable. We’re collecting data across all UCHealth facilities to refine the AI and account for variations in layouts, staffing, and available resources. Local adaptation is key for success!
Editor: MedTechNews.Uk
Thank you to our Sponsor Esdebe