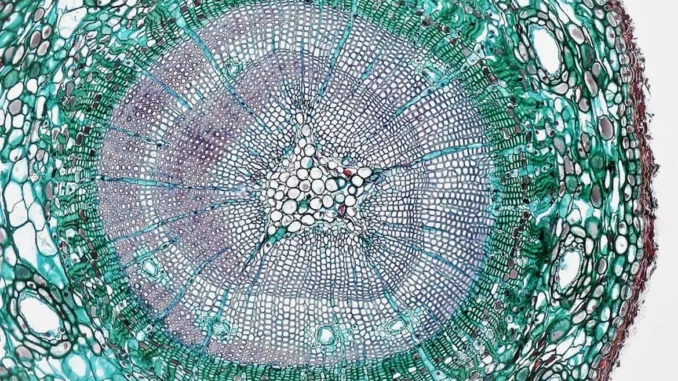
In the ever-evolving landscape of medical diagnostics, the integration of artificial intelligence (AI) has begun to significantly alter the way diseases are detected and analysed. The recent developments at Washington State University (WSU) introduce a cutting-edge deep learning AI model that not only matches but often surpasses human experts in identifying pathologies from tissue images. Published in the esteemed journal Scientific Reports, this breakthrough is set to transform both clinical diagnostics and research related to diseases, offering unparalleled speed and precision.
The foundation of this advancement rests on deep learning, an advanced AI technique that mimics the neural networks of the human brain. Unlike conventional machine learning, which relies on human input to identify features, deep learning independently discerns intricate patterns within data. This capability makes it especially effective for complex image analysis. Developed by computer scientists Colin Greeley and Lawrence Holder, the WSU model was trained on images from previous epigenetic studies led by biologist Michael Skinner. These studies concentrated on detecting molecular disease indicators in various tissues, such as kidneys and reproductive organs, at a molecular level.
One of the most remarkable features of the WSU deep learning model is its ability to process gigapixel images, which are extremely high-resolution images encompassing billions of pixels. Traditional methods of analysing such images are not only laborious but also prone to human error. The AI model circumvents these challenges by examining smaller sections of the image while maintaining the overall context, akin to finely tuning a microscope’s focus. This innovative approach not only streamlines the analytical process but also enhances accuracy, enabling the AI to detect pathologies that might elude human experts.
The implications of this technological advancement are extensive. In the realm of Skinner’s epigenetic research, where molecular changes can influence gene behaviour without altering DNA sequences, analyses that previously spanned a year can now be completed within weeks. This acceleration is critical for large-scale studies, potentially expediting the understanding of diseases and fostering significant breakthroughs. Moreover, the AI model’s applications extend beyond research laboratories into practical domains. Holder’s team is actively working with WSU veterinary researchers to diagnose diseases in deer and elk tissue samples, showcasing the model’s versatility and its potential benefits for both human and veterinary medicine.
In human healthcare, the AI model’s proficiency in accurately identifying cancerous tissues and other gene-related diseases heralds a new era in diagnostics. By drastically reducing the time required for pathologists to analyse biopsy images from hours to mere minutes, the AI model not only enhances efficiency but also heightens the chances of early disease detection. Early diagnosis is crucial for successful treatment outcomes, making this AI model a potential game-changer in medical practice.
The creation of this deep learning AI model marks a significant milestone in the integration of AI into medical diagnostics. By outperforming human capabilities in pathology identification, the model illustrates AI’s potential to complement and elevate human expertise. As AI technology continues to evolve, it is anticipated to play an ever-more vital role in personalised medicine, facilitating treatment plans tailored to precise diagnostic data.
This technological triumph also highlights the importance of interdisciplinary collaboration in advancing medical research. WSU’s achievement, through the convergence of computer science, biology, and veterinary medicine experts, demonstrates the power of combining diverse fields to achieve groundbreaking results. The deep learning AI model developed at Washington State University stands as a transformative innovation in pathology identification. Its speed, precision, and adaptability promise to accelerate disease research and improve diagnostic practices across both animal and human medicine. As this technology advances, it is poised to become an indispensable asset in the pursuit of improved healthcare outcomes.
Be the first to comment