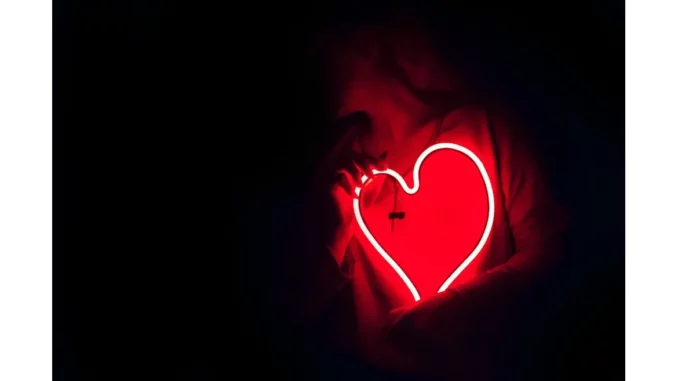
The convergence of artificial intelligence (AI) and preventive cardiology is heralding a transformative era, particularly in the field of coronary artery calcium scoring (CACS). Cardiovascular disease (CVD) remains the predominant cause of mortality in the United States, necessitating the development of precise and efficient risk assessment tools. Coronary artery calcium (CAC) serves as a crucial indicator for coronary heart disease (CHD) and atherosclerotic cardiovascular disease (ASCVD), offering vital insights into an individual’s risk profile. However, the traditional methods employed for CAC scoring, which predominantly rely on ECG-gated non-contrast computed tomography (NCCT), present certain limitations, including significant costs, radiation exposure, and the requirement for specialised imaging protocols.
AI, with its capacity to execute tasks on par with human ability, stands ready to revolutionise the efficiency and accessibility of coronary artery calcium scoring. By harnessing machine learning and deep learning algorithms, AI can automate the complex tasks of calcium segmentation and quantification. This reduces the dependence on dedicated CT protocols, allowing for the utilisation of non-dedicated CT scans for calcium scoring. Such advancements are particularly advantageous in mitigating radiation exposure and reducing overall costs, thereby making preventive cardiology more widely accessible to a diverse patient populace.
The promise of AI in CACS is highlighted by several recent investigations that have demonstrated its effectiveness across a variety of CT protocols. Notably, AI models have been devised to extract quantitative CACS from non-dedicated routine chest CT protocols, such as low-dose CT (LDCT), cardiac CT angiography (CCTA), and positron emission tomography (PET)/CT attenuation scans. These models have yielded promising results, particularly in the realms of sensitivity, specificity, and processing time, frequently surpassing the capabilities of traditional manual methods.
Nonetheless, despite these technological advancements, the widespread integration of AI-driven CACS encounters several significant obstacles. The absence of insurance coverage for CACS, coupled with the necessity for specialised imaging personnel and the lack of standardised protocols for non-dedicated CT use, constitutes substantial barriers. Additionally, the generalisation of AI models across different populations poses a challenge, as many models tend to overfit, performing well with training data but faltering with external datasets.
To surmount these challenges, developing diverse multi-detector and multi-institutional data banks is essential. Such repositories will facilitate the training and validation of AI models, ensuring their applicability across various patient demographics and clinical environments. Furthermore, enhancing data diversity, external validation, and metric standardisation will augment the reliability of AI-driven CACS. These steps are vital to ensuring that AI models can be effectively implemented in real-world scenarios, ultimately enhancing patient care.
In drawing these threads together, AI-driven advancements in coronary calcium scoring offer remarkable potential for reshaping preventive cardiology. By optimising workflow, reducing costs, and facilitating the use of non-dedicated CT scans, AI can significantly improve the early detection and management of cardiovascular disease. As these technologies continue to evolve, the integration of AI into routine clinical practice is set to play a pivotal role in alleviating the global burden of cardiovascular disease. The ongoing development and refinement of AI methodologies promise not only to enhance clinical outcomes but also to expand the accessibility of preventive cardiological care, heralding a new chapter in the fight against heart disease.
Be the first to comment