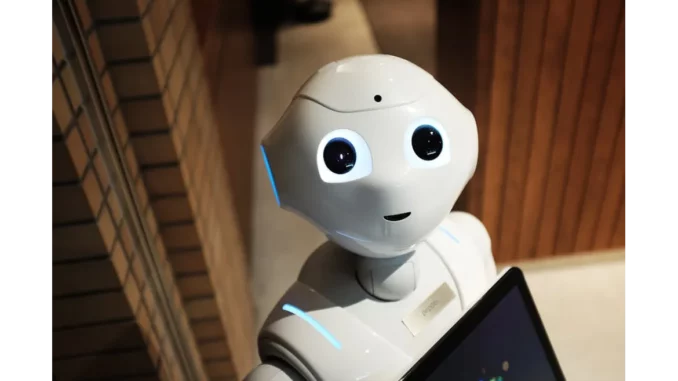
Reflecting on a century of advancements in artificial intelligence (AI), the evolution of explainable AI (XAI) stands out as one of the most compelling developments. With AI systems increasingly embedded in critical sectors such as healthcare, automotive, and finance, the importance of understanding how these systems make decisions is paramount. The journey of explainable AI is not merely a story of technological progress, but it also involves grappling with ethical and practical challenges posed by machines that impact human lives.
Safeguard patient information with TrueNASs self-healing data technology.
The origins of explainability in AI can be traced back to the early 20th century, with the introduction of the “black box” metaphor. This concept described systems whose internal mechanisms were obscured, visible only through their inputs and outputs. The need for transparency became evident with the development of neural networks in the 1940s and 50s by pioneers like Warren McCulloch and Walter Pitts. Their work laid the foundation for neural networks, including the perceptron developed by Frank Rosenblatt, which aimed to replicate human learning and decision-making processes. However, as models grew in complexity, they also became more opaque, posing significant interpretability challenges.
During the 1980s and 90s, deep learning experienced considerable advancements, notably through the introduction of backpropagation and convolutional neural networks (CNNs) by researchers such as Yann LeCun. These innovations enabled AI systems to process and analyse vast datasets, achieving remarkable accuracy in tasks like image recognition and natural language processing. Yet, the complexity of these models made them less interpretable, underscoring the persistent “black box” problem. As AI systems became more prevalent, the demand for transparency and accountability surged, giving rise to XAI as a distinct field of research. In the early 2000s, techniques such as saliency maps and LIME emerged, aiming to offer insights into AI decision-making processes. These methods signalled a shift towards a more human-centred approach to AI, where the goal was not only to create accurate models but also to ensure their decisions could be comprehended and trusted by humans.
In recent years, the importance of explainable AI has been further magnified by the rise of large language models (LLMs) and the increasing integration of AI into high-stakes applications. The European Union’s AI Act, which came into effect in 2024, mandates transparency and accountability in AI systems, underscoring the necessity for explainability. This regulatory framework reflects a broader trend towards responsible AI, focusing on creating systems that are not only effective but also ethical and trustworthy. However, the field of explainable AI continues to face several challenges. A primary issue is the trade-off between model complexity and interpretability. As AI models become more sophisticated, deciphering their decision-making processes becomes increasingly difficult. Researchers are actively working on developing new methods that can provide meaningful explanations without compromising model performance.
Moreover, ensuring that explanations are accessible and meaningful to diverse stakeholders is another significant challenge. This requires a nuanced understanding of the needs and expectations of various user groups, from AI developers to end-users and regulators. The aim is to create explanations that are not only technically sound but also actionable and relevant to the intended audience. This involves balancing the technical intricacies of AI models with the practical requirements of users, ensuring that the information provided is both insightful and comprehensible.
Looking to the future, the potential of explainable AI is immense. As research continues to advance, we anticipate the development of more sophisticated methods for interpreting AI models and the establishment of robust frameworks for integrating explainability into the AI development lifecycle. By addressing the challenges and seizing the opportunities, explainable AI can play a pivotal role in fostering trust and ensuring the responsible use of AI technologies in the coming years. This evolution promises to bridge the gap between complex AI systems and human understanding, creating a more transparent and accountable technological landscape.
Be the first to comment