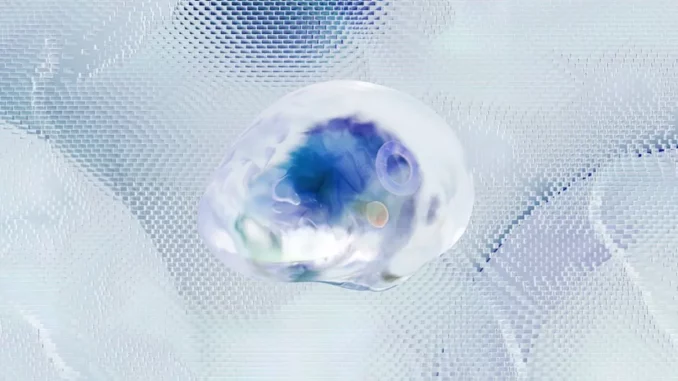
During my recent discussion with Dr. Emily Zhang, a research scientist collaborating with Yaping Qi on an innovative project, her palpable excitement was infectious. Her enthusiasm illuminated the complex domain of two-dimensional (2D) materials and the transformative role that deep learning innovations are playing in their analysis. Our conversation ventured into both the scientific intricacies and practical ramifications of this research, offering a glimpse into the promising synergy between deep learning and materials science.
Dr. Zhang candidly shared her relief at the advancements their research has brought. “Traditional methods for analysing Raman spectra are often laborious and susceptible to human error,” she confessed. The conventional approach necessitates extensive manual interpretation, which can be both time-consuming and prone to inaccuracies. This sentiment is widely shared within the scientific community, which has longed for a more efficient methodology. The research team at Tohoku University, under Yaping Qi’s leadership, has pioneered a new deep learning-based method that significantly advances the identification and classification of 2D materials. Central to this approach is the Denoising Diffusion Probabilistic Model (DDPM), a type of generative model adept at completing spectral data, much like filling in the missing pieces of a jigsaw puzzle.
A major hurdle in the field has always been dealing with limited samples and unevenly distributed data. As Dr. Zhang explained, “Relying on traditional methods can skew analysis when only a handful of samples are available.” The DDPM framework addresses this issue by generating synthetic data, which is subsequently enhanced by a four-layer convolutional neural network (CNN). This innovative combination has yielded remarkable results, with classification accuracy soaring to 98.8% using the original dataset and achieving a flawless 100% with the augmented data. Dr. Zhang emphasised how this automated approach not only enhances classification performance but also minimises the need for manual intervention, making the process more efficient and scalable.
The broader implications of this research are profound. Dr. Zhang highlighted the vast potential applications across various fields, from electronics to medical technologies, where 2D materials play a pivotal role. By accelerating the development and analysis of these materials, the research not only advances materials science but also potentially catalyses innovations in diverse industries. Moreover, the environmental benefits of this approach are noteworthy. Faster and more precise identification of materials leads to less waste and more efficient resource utilisation, presenting a more sustainable approach to materials research.
This study is pioneering in its application of DDPM to Raman spectral data generation, producing promising results. The method allows for precise material characterisation even when experimental data is scarce or challenging to obtain. Such breakthroughs could significantly streamline the transition from laboratory research to real-world applications, facilitating the quicker development of consumer products.
As our conversation concluded, Dr. Zhang expressed optimism about the future of this research. “We’re merely scratching the surface,” she remarked with a smile, alluding to the limitless possibilities for refining and expanding this model. The integration of deep learning techniques into materials science is still nascent, yet the potential for future developments is vast.
Reflecting on my dialogue with Dr. Zhang, it is apparent that this deep learning-based approach signifies more than a technical progression; it is a transformative tool poised to shape the future of materials science. As researchers continue to explore the capabilities of AI and machine learning, the horizon for new discoveries and innovations appears ever brighter. In the realm of 2D materials, this advancement is not merely a step forward but a leap into a new era of rapid, precise, and automated material identification that could redefine the landscape of scientific research and industrial applications alike.
Be the first to comment