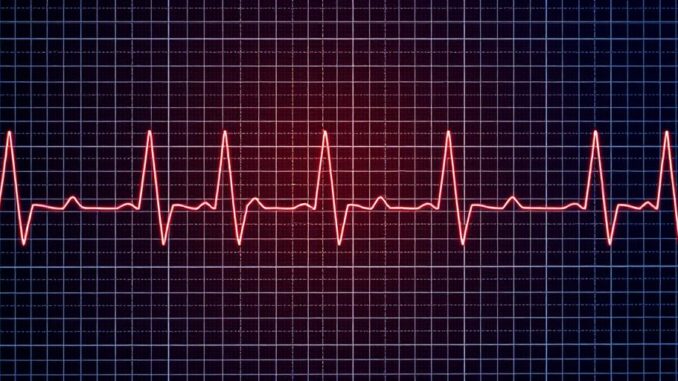
Abstract
Electrocardiography (ECG) stands as a cornerstone in cardiovascular diagnostics, providing a non-invasive window into the heart’s electrical activity. This research report delves into the multifaceted aspects of ECG, ranging from its fundamental principles and waveform interpretation to the limitations inherent in its application and the transformative advancements reshaping the field. We explore the electrophysiological basis of ECG signals, dissecting the components of a standard ECG complex and their correlation to specific cardiac events. Furthermore, we critically examine the challenges associated with ECG interpretation, including artifacts and patient-specific variations. The latter portion of this report is dedicated to exploring the current state-of-the-art advancements in ECG technology, notably artificial intelligence (AI)-driven analysis, remote monitoring capabilities, and high-resolution ECG techniques. Finally, we discuss the implications of these advancements for improved diagnostic accuracy, personalized medicine, and proactive cardiovascular health management, while also considering the ethical and regulatory landscapes surrounding these emerging technologies. This review aims to provide a comprehensive overview, useful for both established experts and those seeking a deeper understanding of the dynamic landscape of electrocardiography.
Many thanks to our sponsor Esdebe who helped us prepare this research report.
1. Introduction
The electrocardiogram (ECG or EKG) has revolutionized the diagnosis and management of cardiac disease since its inception by Willem Einthoven in the early 20th century [1]. By measuring the electrical activity of the heart over time, the ECG provides invaluable insights into various cardiac conditions, ranging from arrhythmias and ischemia to structural abnormalities and electrolyte imbalances. The ECG’s non-invasive nature, relative affordability, and widespread availability have cemented its position as an indispensable diagnostic tool in both acute and chronic care settings. However, despite its clinical utility, ECG interpretation can be complex and is subject to inter-observer variability. Furthermore, the conventional 12-lead ECG provides only a snapshot of cardiac electrical activity, often missing transient or intermittent events. This limitation has fueled the development of advanced ECG technologies aimed at improving diagnostic accuracy, extending monitoring capabilities, and enhancing the accessibility of ECG-based cardiac assessments. This report will critically analyze the fundamental principles of ECG, explore its limitations, and examine the most promising advancements in the field, including AI-driven interpretation and remote monitoring solutions.
Many thanks to our sponsor Esdebe who helped us prepare this research report.
2. Electrophysiological Basis of the ECG
The ECG is a recording of the electrical potentials generated by the heart during depolarization and repolarization of the atrial and ventricular myocardium. These electrical potentials are conducted through the body and can be detected by electrodes placed on the skin. The resulting waveforms reflect the sequential activation and recovery of different regions of the heart. Understanding the electrophysiological basis of ECG signals is crucial for accurate interpretation and diagnosis.
2.1 Cellular Electrophysiology
The resting membrane potential of cardiac myocytes is typically around -90 mV, maintained by the selective permeability of the cell membrane to ions, primarily potassium (K+), sodium (Na+), and calcium (Ca2+). Depolarization occurs when the cell membrane becomes more permeable to Na+ and Ca2+, leading to an influx of positively charged ions and a shift in the membrane potential towards a more positive value. Repolarization is the process by which the cell membrane potential returns to its resting state, primarily through the efflux of K+ ions. These ionic currents are responsible for the action potentials that propagate throughout the heart, triggering coordinated contraction of the cardiac muscle. Different cardiac cells (e.g., sinoatrial (SA) node cells, atrial myocytes, ventricular myocytes, Purkinje fibers) exhibit distinct action potential morphologies due to variations in the expression and function of ion channels.
2.2 Conduction System of the Heart
The heart’s conduction system consists of specialized cells that rapidly transmit electrical impulses throughout the myocardium, ensuring coordinated atrial and ventricular contraction. The sinoatrial (SA) node, located in the right atrium, is the heart’s primary pacemaker, initiating electrical impulses that spread through the atria, causing atrial depolarization (represented by the P wave on the ECG). The impulse then reaches the atrioventricular (AV) node, which delays the signal to allow for complete atrial contraction before ventricular activation. The impulse then travels through the Bundle of His, which divides into the left and right bundle branches, distributing the signal to the left and right ventricles, respectively. Ventricular depolarization is represented by the QRS complex on the ECG. Finally, ventricular repolarization is reflected by the T wave.
2.3 ECG Waveforms and Intervals
A standard ECG complex consists of several distinct waveforms and intervals, each reflecting a specific phase of the cardiac cycle:
- P Wave: Represents atrial depolarization. Its morphology and duration can provide insights into atrial abnormalities, such as atrial enlargement or atrial fibrillation.
- PR Interval: Represents the time interval between the onset of atrial depolarization (P wave) and the onset of ventricular depolarization (QRS complex). Prolongation of the PR interval can indicate AV block, while shortening can be seen in conditions like Wolff-Parkinson-White syndrome.
- QRS Complex: Represents ventricular depolarization. Its morphology, amplitude, and duration are crucial for identifying ventricular abnormalities, such as ventricular hypertrophy, bundle branch blocks, and ventricular arrhythmias.
- ST Segment: Represents the period between ventricular depolarization and repolarization. ST segment elevation or depression is a hallmark of myocardial ischemia or infarction.
- T Wave: Represents ventricular repolarization. T wave abnormalities can be indicative of ischemia, electrolyte imbalances, or other cardiac conditions.
- QT Interval: Represents the total duration of ventricular depolarization and repolarization. Prolongation of the QT interval can increase the risk of ventricular arrhythmias, such as torsades de pointes.
Many thanks to our sponsor Esdebe who helped us prepare this research report.
3. ECG Interpretation: Challenges and Pitfalls
While ECG is a powerful diagnostic tool, its interpretation is not without challenges. Accurate interpretation requires a thorough understanding of normal ECG variations, as well as the ability to recognize and differentiate pathological patterns. Several factors can complicate ECG interpretation, including artifacts, patient-specific variations, and inter-observer variability.
3.1 Artifacts
Artifacts are unwanted signals that can distort the ECG tracing and mimic pathological conditions. Common sources of artifacts include:
- Muscle Tremor: Can cause irregular, high-frequency signals that obscure the underlying ECG waveform.
- Movement Artifact: Caused by patient movement or loose electrodes, resulting in baseline wander or abrupt changes in the ECG signal.
- Electrical Interference: Can introduce 60 Hz (or 50 Hz in some countries) interference, creating a fuzzy or noisy ECG tracing.
Careful attention to electrode placement, patient positioning, and environmental factors can help minimize artifacts and improve ECG quality.
3.2 Patient-Specific Variations
Normal ECG variations can occur based on age, sex, body habitus, and underlying medical conditions. For example:
- Age: Children typically have faster heart rates and shorter PR and QT intervals compared to adults.
- Sex: Women may have slightly shorter QRS durations and smaller T wave amplitudes than men.
- Body Habitus: Obese individuals may have lower voltage ECG complexes due to increased tissue impedance.
- Underlying Medical Conditions: Conditions such as hypertension, heart failure, and electrolyte imbalances can affect ECG morphology.
Clinicians must be aware of these variations when interpreting ECGs to avoid misdiagnosis.
3.3 Inter-Observer Variability
ECG interpretation is subjective, and studies have shown significant inter-observer variability, particularly in the interpretation of subtle ECG abnormalities. This variability can lead to inconsistent diagnoses and treatment decisions. Efforts to improve inter-observer agreement include standardized training programs, the use of computer-aided interpretation tools, and the development of consensus guidelines. However, even with these measures, inter-observer variability remains a significant challenge in ECG interpretation. I believe that the integration of AI-powered interpretation tools can play a significant role in mitigating the subjective element and improving consistency across different observers, though careful validation and regulatory oversight are essential.
Many thanks to our sponsor Esdebe who helped us prepare this research report.
4. Advancements in ECG Technology
The limitations of conventional ECG technology have spurred the development of several advancements aimed at improving diagnostic accuracy, extending monitoring capabilities, and enhancing accessibility.
4.1 AI-Powered ECG Analysis
Artificial intelligence (AI), particularly deep learning algorithms, has shown tremendous promise in ECG analysis. AI algorithms can be trained to automatically detect and classify various cardiac arrhythmias, identify subtle ECG abnormalities that may be missed by human interpreters, and predict the risk of future cardiac events. Several AI-powered ECG interpretation tools have been developed and are being used in clinical practice. While these tools show great promise, it is crucial to validate their performance rigorously and address concerns about bias and interpretability. The ‘black box’ nature of some deep learning models raises concerns about trust and accountability. Future research should focus on developing more transparent and explainable AI models that can provide clinicians with insights into the reasoning behind their diagnoses.
4.2 Remote and Wearable ECG Monitoring
Remote ECG monitoring technologies, such as Holter monitors, event recorders, and wearable ECG devices, allow for continuous or intermittent monitoring of cardiac electrical activity over extended periods. These technologies are particularly useful for detecting transient or infrequent arrhythmias that may not be captured by a standard 12-lead ECG. Wearable ECG devices, such as smartwatches and chest-worn sensors, are becoming increasingly popular for personal cardiac monitoring. These devices can detect arrhythmias, such as atrial fibrillation, and alert users to seek medical attention. While remote ECG monitoring has the potential to improve early detection and management of cardiac arrhythmias, it also raises concerns about data privacy, security, and the potential for over-diagnosis and unnecessary interventions. A critical evaluation of the clinical utility and cost-effectiveness of these technologies is essential before widespread adoption. I predict that as these technologies mature, they will become integrated into more comprehensive telehealth platforms, facilitating remote consultations and personalized cardiac care.
4.3 High-Resolution ECG (HRECG)
High-resolution ECG (HRECG) is a technique that uses advanced signal processing methods to enhance the resolution of ECG signals, allowing for the detection of subtle abnormalities that may be missed by conventional ECG. HRECG has been used to identify patients at risk for ventricular arrhythmias and sudden cardiac death, particularly in patients with structural heart disease. While HRECG has shown promise in risk stratification, its clinical utility remains debated, and its use is not yet widespread.
4.4 Vectorcardiography (VCG)
Vectorcardiography (VCG) represents the heart’s electrical activity as a series of vectors in three dimensions, providing a more comprehensive view of cardiac depolarization and repolarization compared to the standard 12-lead ECG. VCG can be particularly useful for diagnosing subtle conduction abnormalities and detecting myocardial ischemia. While VCG offers certain advantages over standard ECG, it requires specialized equipment and expertise, limiting its widespread use. However, with advancements in computational power and signal processing techniques, VCG may see a resurgence in clinical practice. Modern VCG systems can be integrated with standard ECG equipment, making it easier to acquire and interpret VCG data. I believe that the combination of VCG with AI-powered analysis could unlock new insights into cardiac electrophysiology and improve diagnostic accuracy.
Many thanks to our sponsor Esdebe who helped us prepare this research report.
5. Role of ECG in Diagnosing Heart Conditions
The ECG plays a crucial role in the diagnosis and management of a wide range of heart conditions.
5.1 Arrhythmias
The ECG is the primary tool for diagnosing cardiac arrhythmias, including atrial fibrillation, atrial flutter, supraventricular tachycardia, ventricular tachycardia, and ventricular fibrillation. The ECG can identify the type of arrhythmia, determine its severity, and guide treatment decisions. Remote ECG monitoring technologies are particularly valuable for detecting paroxysmal or intermittent arrhythmias. AI-powered ECG analysis can help to automate the detection of arrhythmias and reduce the workload on clinicians.
5.2 Myocardial Ischemia and Infarction
ST segment elevation or depression on the ECG is a hallmark of myocardial ischemia or infarction. The ECG can help to differentiate between ST-segment elevation myocardial infarction (STEMI) and non-ST-segment elevation myocardial infarction (NSTEMI), which require different treatment strategies. Early diagnosis and treatment of myocardial infarction are crucial for minimizing myocardial damage and improving patient outcomes. AI-powered ECG analysis can help to expedite the diagnosis of myocardial infarction and improve triage decisions.
5.3 Hypertrophy and Cardiomyopathy
The ECG can provide clues about the presence of left ventricular hypertrophy (LVH), right ventricular hypertrophy (RVH), and various cardiomyopathies. Specific ECG criteria, such as the Sokolow-Lyon index, can be used to diagnose LVH. However, the sensitivity and specificity of ECG for diagnosing hypertrophy and cardiomyopathy are limited, and additional diagnostic tests, such as echocardiography, are often required.
5.4 Electrolyte Imbalances
Electrolyte imbalances, such as hyperkalemia and hypokalemia, can affect the ECG waveform. Hyperkalemia can cause peaked T waves, prolonged PR interval, and QRS widening, while hypokalemia can cause flattened T waves, prominent U waves, and ST segment depression. The ECG can help to identify electrolyte imbalances and guide appropriate treatment.
5.5 Other Cardiac Conditions
The ECG can also be useful in diagnosing other cardiac conditions, such as pericarditis, pulmonary embolism, and drug-induced cardiotoxicity. Specific ECG patterns, such as diffuse ST segment elevation in pericarditis and S1Q3T3 pattern in pulmonary embolism, can provide valuable diagnostic information.
Many thanks to our sponsor Esdebe who helped us prepare this research report.
6. Future Directions and Challenges
Electrocardiography continues to evolve at a rapid pace, driven by advancements in technology and a growing understanding of cardiac electrophysiology. Several promising areas of research and development are poised to shape the future of ECG.
6.1 Personalized ECG Interpretation
Future ECG interpretation algorithms will likely incorporate patient-specific data, such as age, sex, ethnicity, medical history, and genetic information, to provide more personalized and accurate diagnoses. This approach will require large datasets and sophisticated machine learning techniques to identify subtle patterns and individual variations. I anticipate a shift towards integrating ECG data with other clinical data, such as imaging results, laboratory values, and genomic information, to create a more holistic and individualized assessment of cardiac risk.
6.2 Integration with Telehealth and Remote Patient Monitoring
The integration of ECG with telehealth platforms and remote patient monitoring systems will enable more proactive and personalized cardiac care. Remote ECG monitoring can be used to detect early signs of cardiac deterioration and facilitate timely interventions. Telehealth consultations can provide patients with convenient access to expert cardiac care, regardless of their location. This integration will require seamless data sharing, secure communication protocols, and robust data analytics capabilities.
6.3 Addressing Ethical and Regulatory Challenges
The increasing use of AI-powered ECG analysis and remote ECG monitoring raises several ethical and regulatory challenges. Concerns about data privacy, security, and bias must be addressed to ensure responsible and equitable use of these technologies. Regulatory frameworks must be developed to ensure the safety and efficacy of AI-powered ECG devices and to protect patient rights. I believe that open-source datasets and transparent AI algorithms can promote trust and accountability in this rapidly evolving field.
6.4 Development of Novel ECG Biomarkers
Future research may lead to the discovery of novel ECG biomarkers that can improve the diagnosis and risk stratification of cardiac disease. These biomarkers could include subtle changes in ECG morphology, timing, or variability that are not readily apparent to the human eye. Advanced signal processing techniques and machine learning algorithms will be essential for identifying and validating these novel biomarkers.
Many thanks to our sponsor Esdebe who helped us prepare this research report.
7. Conclusion
Electrocardiography remains a vital tool in the diagnosis and management of cardiac disease. While the fundamental principles of ECG have been well-established for over a century, ongoing advancements in technology are transforming the field. AI-powered ECG analysis, remote monitoring capabilities, and high-resolution ECG techniques hold the potential to improve diagnostic accuracy, extend monitoring capabilities, and enhance accessibility. However, these advancements also raise ethical and regulatory challenges that must be addressed to ensure responsible and equitable use. By embracing innovation and addressing these challenges, we can unlock the full potential of electrocardiography to improve cardiovascular health outcomes. Continued research into novel ECG biomarkers and personalized ECG interpretation algorithms will further refine the diagnostic and prognostic capabilities of this invaluable tool. The future of electrocardiography is bright, promising a new era of proactive and personalized cardiac care.
Many thanks to our sponsor Esdebe who helped us prepare this research report.
References
[1] Einthoven, W. (1912). The Mechanism of the Electrocardiogram. The Lancet, 180(4634), 853-861.
[2] Goldberger, A. L. (2006). Clinical Electrocardiography: A Simplified Approach. Mosby.
[3] Macfarlane, P. W., & Lawrie, T. D. V. (Eds.). (2011). Comprehensive Electrocardiology. Springer Science & Business Media.
[4] Rautaharju, P. M., Surawicz, B., Gettes, L. S., Bailey, J. J., & Childers, R. (2009). AHA/ACCF/HRS recommendations for the standardization and interpretation of the electrocardiogram: part IV: current electrocardiography reporting standards. Circulation, 119(10), e235-e240.
[5] Acharya, U. R., Fujita, H., Lih, O. S., Adam, M., Tan, J. H., & Koh, J. E. W. (2017). Automated diagnosis of arrhythmias using different classifiers with features extracted from normal and ectopic ECG beats. Information Sciences, 393, 1-14.
[6] Perez, M. V., Mahaffey, K. W., Quinton, A. R., et al. (2019). Large-Scale Assessment of a Smartwatch to Identify Atrial Fibrillation. New England Journal of Medicine, 381(20), 1909-1917.
[7] Attia, Z. I., Noseworthy, P. A., Lopez-Jimenez, F., et al. (2019). An artificial intelligence-enabled ECG algorithm for the identification of patients with atrial fibrillation during normal sinus rhythm: a prospective observational study. The Lancet, 394(10204), 861-867.
[8] Mason, J. W., Ramseth, D. J., Chan, B. S., & Thames, M. D. (1987). Electrocardiographic T-wave abnormalities that identify patients with idiopathic ventricular fibrillation. Journal of the American College of Cardiology, 10(6), 1221-1226.
[9] Flowers, N. C., Horan, L. G., Thomas, J. R., & Tolleson, W. J. (1969). The anatomic basis for high-frequency components in the electrocardiogram. Circulation, 39(4), 531-538.
[10] Kors, J. A., van Herpen, G., Sitzia, J., Quinn, D., & Macfarlane, P. W. (2003). The diagnostic accuracy of computer interpretation of electrocardiograms. Heart, 89(5), 503-508.
The discussion of AI-powered ECG analysis is fascinating. Exploring the potential of explainable AI in this context could significantly increase clinician trust and adoption by providing insights into the model’s decision-making process.