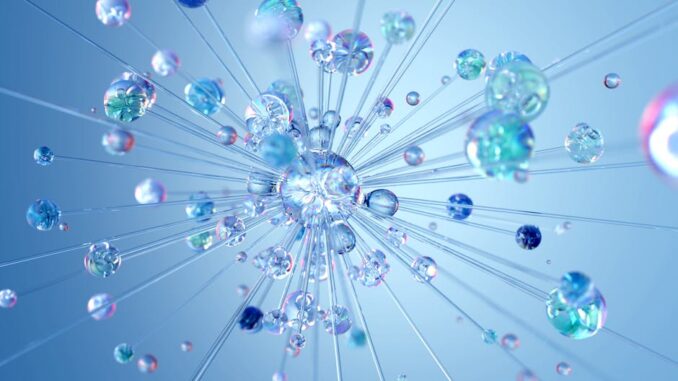
Abstract
Motor skill development is a complex and multifaceted process that underpins a vast array of human capabilities. This research report provides an in-depth exploration of motor skill acquisition, encompassing neurobiological mechanisms, computational modeling, and technological interventions. We delve into the hierarchical organization of motor control, from spinal reflexes to cortical planning, highlighting the contributions of specific brain regions and neural circuits. Furthermore, we examine the role of sensory feedback, error correction, and reinforcement learning in shaping motor performance. We critically assess current computational models of motor learning, including those based on optimal control, Bayesian inference, and neural networks, and discuss their limitations and potential for future development. Finally, we explore the emerging landscape of assistive technologies, including wearable sensors, brain-computer interfaces, and robotic exoskeletons, and their impact on rehabilitation and motor augmentation. Throughout, we emphasize the importance of a multidisciplinary approach that integrates neuroscience, engineering, and clinical practice to advance our understanding of motor skills and improve the lives of individuals with motor impairments. This report also offers perspectives on the ethical implications of increasingly sophisticated assistive technologies and the importance of equitable access to these advancements.
Many thanks to our sponsor Esdebe who helped us prepare this research report.
1. Introduction
Motor skills are fundamental to human behavior, enabling us to interact effectively with our environment, communicate, and express ourselves. From the simple act of reaching for an object to the complex coordination required for playing a musical instrument or performing athletic feats, motor skills are essential for daily life. The acquisition and refinement of these skills is a continuous process, beginning in infancy and continuing throughout adulthood. Understanding the underlying mechanisms of motor skill development is therefore of paramount importance, not only for advancing our basic knowledge of human behavior, but also for developing effective interventions for individuals with motor impairments, such as those caused by stroke, cerebral palsy, or neurodegenerative diseases.
This research report aims to provide a comprehensive overview of motor skill development, integrating perspectives from neuroscience, engineering, and clinical practice. We will begin by examining the neurobiological foundations of motor control, including the roles of the motor cortex, cerebellum, basal ganglia, and spinal cord. We will then delve into the computational principles that govern motor learning, exploring how the brain optimizes motor performance through processes such as error correction, reinforcement learning, and predictive modeling. Furthermore, we will discuss the impact of sensory feedback on motor control, including the contributions of visual, proprioceptive, and vestibular information. We will then move on to examine the emerging landscape of assistive technologies, including wearable sensors, brain-computer interfaces, and robotic exoskeletons, and their potential for rehabilitation and motor augmentation. Finally, we will consider the ethical implications of these technologies and the importance of equitable access to motor skill enhancement.
Many thanks to our sponsor Esdebe who helped us prepare this research report.
2. Neurobiological Foundations of Motor Control
The neural control of movement is a complex and hierarchical process, involving multiple brain regions and neural circuits. At the highest level, the motor cortex is responsible for planning and initiating voluntary movements. The primary motor cortex (M1) contains a somatotopic map of the body, with different regions controlling different muscle groups. However, motor control is not simply a matter of activating specific muscles in isolation. Instead, movements are coordinated patterns of muscle activity, and the motor cortex works in concert with other brain regions to produce smooth and accurate movements.
The premotor cortex (PMC) and supplementary motor area (SMA) are involved in planning and sequencing movements. The PMC is particularly important for visually guided movements, while the SMA is involved in internally generated movements. These areas receive input from the parietal cortex, which provides information about the body’s position in space. The cerebellum plays a crucial role in motor coordination and error correction. It receives input from the motor cortex, spinal cord, and vestibular system, and uses this information to fine-tune movements and ensure that they are accurate and coordinated. The cerebellum is also involved in motor learning, particularly the acquisition of procedural skills.
The basal ganglia are a group of subcortical nuclei that play a role in selecting and initiating movements. They receive input from the motor cortex and other brain regions, and use this information to decide which movements to execute and which to suppress. The basal ganglia are also involved in reward-based learning, helping us to learn which movements are most likely to lead to positive outcomes. The spinal cord is the final pathway for motor commands. It receives input from the motor cortex and brainstem, and transmits this information to the muscles. The spinal cord also contains local circuits that are responsible for reflexes and other basic motor functions. Descending pathways from the brain, such as the corticospinal tract, modulate the activity of these circuits to produce voluntary movements.
2.1 The Role of Sensory Feedback:
Sensory feedback plays a crucial role in motor control, allowing us to monitor our movements and make adjustments as needed. Visual feedback is particularly important for visually guided movements, allowing us to track the position of our limbs and adjust our movements to reach our target. Proprioceptive feedback, from muscles and joints, provides information about the position and movement of our body in space. Vestibular feedback, from the inner ear, provides information about our head position and movement. These sensory inputs are integrated in the brain to create a coherent representation of our body and its environment. Models of motor control increasingly emphasize the predictive capabilities of the brain, where internal models predict the sensory consequences of actions. Discrepancies between predicted and actual sensory feedback are then used to update the internal model and improve future movements. This concept, known as sensorimotor adaptation, highlights the brain’s ability to learn and adapt to changing environmental conditions.
Many thanks to our sponsor Esdebe who helped us prepare this research report.
3. Computational Principles of Motor Learning
Motor learning is the process by which we acquire and refine motor skills. This process involves a variety of computational mechanisms, including error correction, reinforcement learning, and predictive modeling. Error correction is a fundamental mechanism of motor learning. When we make an error, our brain detects the discrepancy between our intended movement and our actual movement, and uses this information to adjust our future movements. This process is thought to be mediated by the cerebellum, which compares the actual sensory feedback from a movement to the predicted sensory feedback, and generates an error signal that is used to update the motor plan. Reinforcement learning is another important mechanism of motor learning. When we perform a movement that leads to a positive outcome, our brain releases dopamine, a neurotransmitter that strengthens the neural connections associated with that movement. This process helps us to learn which movements are most likely to lead to positive outcomes. Predictive modeling is a more recent approach to understanding motor learning. This approach suggests that the brain builds internal models of the body and its environment, and uses these models to predict the consequences of our actions. These models are constantly updated based on sensory feedback, allowing us to make accurate predictions and perform movements with greater precision.
3.2 Computational Models:
Computational models provide a powerful tool for understanding the underlying mechanisms of motor learning. Several different types of computational models have been developed, including optimal control models, Bayesian inference models, and neural network models. Optimal control models assume that the brain chooses motor commands to minimize a cost function, such as energy expenditure or movement error. These models can be used to predict how people will move in different situations, and how they will adapt to changes in their environment. Bayesian inference models assume that the brain combines prior knowledge with sensory evidence to make inferences about the state of the world. These models can be used to explain how people integrate different sources of information to make decisions about movement. Neural network models simulate the activity of neurons and neural circuits. These models can be used to explore how different brain regions interact to produce motor behavior, and how these interactions change during learning. While each of these approaches offers valuable insights, none is without limitations. Optimal control models often rely on simplified representations of the body and environment, and may not capture the full complexity of motor behavior. Bayesian inference models can be computationally expensive, and may not be suitable for real-time motor control. Neural network models can be difficult to interpret, and may not provide clear insights into the underlying computational principles.
Future research will likely focus on developing more sophisticated computational models that integrate different approaches. For example, hybrid models that combine optimal control with Bayesian inference could provide a more complete understanding of motor learning. Similarly, neural network models that are trained on realistic data could provide more accurate predictions of motor behavior.
Many thanks to our sponsor Esdebe who helped us prepare this research report.
4. Assistive Technologies for Motor Impairments
Assistive technologies are devices and systems that are designed to help individuals with disabilities to perform tasks that they would otherwise be unable to do. In the context of motor impairments, assistive technologies can be used to improve mobility, communication, and daily living activities. Wearable sensors, such as accelerometers and gyroscopes, can be used to track movements and provide feedback to individuals with motor impairments. These sensors can be integrated into clothing or attached to the body, and can be used to monitor gait, posture, and other aspects of movement. This data can be used to provide real-time feedback to help individuals improve their motor control, or to track progress over time. Brain-computer interfaces (BCIs) are devices that allow individuals to control external devices using their brain activity. BCIs can be used to control computers, wheelchairs, and other assistive devices. These interfaces typically involve measuring electrical activity in the brain using electrodes placed on the scalp (electroencephalography, EEG) or implanted in the brain (electrocorticography, ECoG). The brain activity is then decoded to determine the user’s intent, and used to control the external device. Robotic exoskeletons are wearable robots that provide assistance to individuals with motor impairments. These devices can be used to support weakened limbs, provide assistance with walking, or enable individuals to perform tasks that would otherwise be impossible. Robotic exoskeletons are typically powered by batteries or motors, and can be controlled using a variety of methods, including buttons, joysticks, or BCIs.
4.1 The Impact of Technology on Rehabilitation
Assistive technologies have the potential to revolutionize rehabilitation for individuals with motor impairments. By providing support, feedback, and control, these technologies can help individuals regain motor function, improve their quality of life, and participate more fully in society. However, there are also challenges associated with the use of assistive technologies. These technologies can be expensive, require extensive training, and may not be suitable for all individuals. Furthermore, there are ethical considerations associated with the use of these technologies, such as issues related to privacy, autonomy, and equity.
4.2 Future Directions
Future research in assistive technologies will likely focus on developing more sophisticated and user-friendly devices. This will involve improving the accuracy and reliability of sensors and decoding algorithms, developing more intuitive control interfaces, and reducing the cost and complexity of these technologies. Furthermore, future research will need to address the ethical and social implications of assistive technologies, to ensure that these technologies are used in a way that is beneficial to all individuals.
Many thanks to our sponsor Esdebe who helped us prepare this research report.
5. Ethical Considerations and Equitable Access
The rapid advancement of technologies aimed at enhancing motor skills and restoring function raises several critical ethical considerations. As assistive technologies become more sophisticated and integrated into daily life, questions regarding autonomy, privacy, and equitable access become increasingly important.
5.1 Autonomy and Agency:
Assistive technologies are designed to empower individuals with motor impairments, yet they can also raise concerns about autonomy and agency. For example, BCIs that directly control external devices raise questions about who is in control: the user or the technology? It is crucial to ensure that individuals using assistive technologies retain a sense of agency and control over their own actions. This requires careful design of the technology to ensure that it responds accurately and reliably to the user’s intent, and that users have the ability to override or modify the technology’s actions.
5.2 Privacy and Data Security:
Assistive technologies often collect and store sensitive data about the user’s movements, brain activity, and other personal information. This data could be vulnerable to unauthorized access or misuse. It is essential to implement robust security measures to protect the privacy of users and ensure that their data is used responsibly. This includes obtaining informed consent from users before collecting their data, implementing strong encryption and access controls, and establishing clear policies regarding data sharing and storage.
5.3 Equitable Access:
Assistive technologies can be expensive and may not be accessible to all individuals who could benefit from them. This raises concerns about equity and social justice. It is crucial to ensure that assistive technologies are available to all individuals, regardless of their socioeconomic status, race, ethnicity, or geographic location. This requires addressing the cost of these technologies through government subsidies, insurance coverage, or charitable donations. It also requires developing culturally appropriate and accessible technologies that meet the needs of diverse populations. Addressing the social stigma associated with using these devices is also important to enhance user acceptance. Finally, ongoing innovation should focus on creating open-source solutions and platforms, fostering collaboration and driving down costs, thus making cutting-edge motor assistance accessible to a wider audience.
Many thanks to our sponsor Esdebe who helped us prepare this research report.
6. Conclusion
Motor skill development is a complex and fascinating process that is essential for human behavior. Understanding the neurobiological foundations of motor control, the computational principles of motor learning, and the potential of assistive technologies is crucial for improving the lives of individuals with motor impairments. This research report has provided a comprehensive overview of these topics, highlighting the importance of a multidisciplinary approach that integrates neuroscience, engineering, and clinical practice. As technology continues to advance, it is essential to address the ethical considerations and ensure equitable access to assistive technologies, so that all individuals can benefit from these advancements. Future research should focus on developing more sophisticated computational models of motor learning, improving the accuracy and reliability of assistive technologies, and addressing the ethical and social implications of these technologies. By continuing to advance our understanding of motor skills and developing innovative technologies, we can improve the lives of individuals with motor impairments and unlock the full potential of human movement.
Many thanks to our sponsor Esdebe who helped us prepare this research report.
References
- Wolpert, D. M., & Ghahramani, Z. (2000). Computational principles of movement neuroscience. Nature Neuroscience, 3(11), 1087-1095.
- Shadmehr, R., & Mussa-Ivaldi, F. A. (2012). Biological learning and control. Current Opinion in Neurobiology, 22(6), 1131-1139.
- Doya, K. (2000). Complementary roles of basal ganglia and cerebellum in learning and motor control. Current Opinion in Neurobiology, 10(6), 732-739.
- Scott, S. H. (2004). Optimal feedback control and the neural basis of volitional motor control. Nature Reviews Neuroscience, 5(7), 532-546.
- Riener, R., Lünenburger, L., Colombo, G. (2006). Human-centered robotics applied to gait training and rehabilitation. Journal of Physical and Rehabilitation Medicine, 86, 669-679.
- Hochberg, L. R., Serruya, M. D., Friehs, G. M., Mukand, J. A., Saleh, M., Caplan, A. H., … & Donoghue, J. P. (2006). Neuronal ensemble control of prosthetic devices by a human with tetraplegia. Nature, 442(7099), 164-171.
- Lebedev, M. A., & Nicolelis, M. A. L. (2006). Brain-machine interfaces: past, present and future. Trends in Neurosciences, 29(9), 536-546.
- Velliste, M., Perge, J. A., Mantoan, L. J., Whitlock, J. R., Thakor, N. V., & Schwartz, A. B. (2008). Cortical control of a prosthetic arm for self-feeding. Nature, 453(7198), 1098-1101.
- Iriki, A., & Sakura, O. (2008). The neural basis of tool use by primates: towards an understanding of the body schema and its plasticity. Cerebral Cortex, 8(1), 63-69.
- Rizzo, J. R., Kapur, N., Robinson, D. T., Coggeshall, S., Rizzo III, J. F., & Friedland, D. (2005). Vision rehabilitation: a survey of visual dysfunctions, rehabilitation techniques, and technologies. Journal of Neuro-Ophthalmology, 25(3), 214-226.
- Bostan, A. C., Dum, R. P., & Strick, P. L. (2013). Cerebellar networks with the cerebral cortex and basal ganglia. Trends in Cognitive Sciences, 17(5), 241-254.
- Wolpert, D.M., Diedrichsen, J., Flanagan, J.R. (2011). Principles of sensorimotor learning. Nature Reviews Neuroscience, 12, 739-751.
- Herzfeld, D.J., Vaswani, P.A., Marko, M.K., Ayaz, A., Shadmehr, R. (2014). Encoding of action by populations of neurons in primate primary motor cortex. Journal of Neuroscience, 34, 923-934.
Be the first to comment