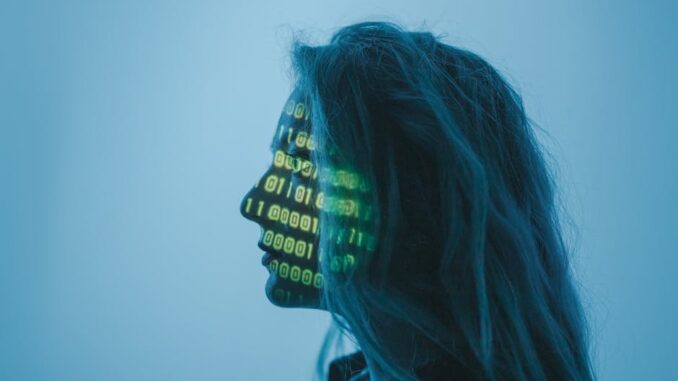
Abstract
This research report provides a comprehensive analysis of the adoption of Artificial Intelligence (AI) within the National Health Service (NHS) in the United Kingdom. It moves beyond specific applications, such as fracture detection, to examine the broader landscape of AI implementation across various clinical and administrative functions. The report delves into the specific AI tools currently deployed within the NHS, explores the multifaceted challenges encountered during their implementation (including data governance, infrastructure limitations, workforce adaptation, and ethical considerations), and evaluates the impact of these technologies on patient care, resource allocation, and overall healthcare delivery. Furthermore, the report assesses the cost-effectiveness of AI solutions in the NHS context and investigates the evolving regulatory and ethical frameworks governing AI in a publicly funded healthcare system. Finally, the report offers a forward-looking perspective, identifying key areas for future research and development and providing recommendations for maximizing the benefits of AI while mitigating potential risks within the NHS.
Many thanks to our sponsor Esdebe who helped us prepare this research report.
1. Introduction
The National Health Service (NHS) is under increasing pressure to deliver high-quality healthcare efficiently and effectively in the face of rising demand, aging populations, and budgetary constraints. Artificial Intelligence (AI) has emerged as a promising tool to address these challenges, offering the potential to automate tasks, improve diagnostic accuracy, personalize treatment plans, and optimize resource allocation. The UK government has recognised this, with several initiatives aimed at supporting AI research and implementation within the NHS. However, the integration of AI into the NHS is not without its challenges. This report aims to provide a comprehensive overview of the current state of AI within the NHS, examining its applications, challenges, and future potential. While examples such as fracture detection are important, this report will consider the broader impact of AI across the NHS landscape.
The report will focus on the following key areas:
- AI Applications: Identifying and analyzing the range of AI tools currently being used or piloted within the NHS, spanning clinical diagnosis, treatment planning, administrative functions, and research.
- Implementation Challenges: Examining the practical challenges encountered during the deployment of AI, including data privacy and security concerns, infrastructure limitations, workforce training requirements, integration with existing systems, and interoperability issues.
- Impact Assessment: Evaluating the impact of AI on patient outcomes, resource allocation, workforce productivity, and overall healthcare delivery efficiency.
- Cost-Effectiveness Analysis: Assessing the economic viability of AI solutions in the NHS context, considering both initial investment costs and long-term operational savings.
- Ethical and Regulatory Considerations: Exploring the ethical dilemmas and regulatory frameworks surrounding the use of AI in a publicly funded healthcare system, including issues of bias, transparency, accountability, and patient autonomy.
- Future Directions: Identifying key areas for future research and development, as well as providing recommendations for maximizing the benefits of AI while mitigating potential risks within the NHS.
Many thanks to our sponsor Esdebe who helped us prepare this research report.
2. AI Applications within the NHS
The NHS is exploring and implementing AI across a wide range of clinical and non-clinical areas. These applications can be broadly categorized as follows:
2.1. Clinical Diagnosis and Decision Support
AI is increasingly being used to assist clinicians in diagnosis and treatment planning. Examples include:
- Medical Image Analysis: AI algorithms are used to analyze medical images such as X-rays, CT scans, and MRIs to detect anomalies and assist radiologists in making more accurate diagnoses. This is where applications like fracture detection fit in. Companies like Behold.ai are already providing such services to the NHS.[1]
- Natural Language Processing (NLP): NLP is used to extract information from electronic health records (EHRs) and other unstructured text data, such as clinical notes, to identify patterns and insights that can aid in diagnosis and treatment planning. For example, NLP can identify patients at high risk of developing certain conditions based on their medical history. Systems like DeepMind’s Streams (now Google Health) aimed at alerting clinicians to acute kidney injury leveraged such capabilities [2].
- Predictive Analytics: AI models are used to predict patient outcomes, such as the likelihood of hospital readmission or the risk of developing complications after surgery. This allows clinicians to intervene proactively and improve patient outcomes.
- Precision Medicine: AI is used to analyze patients’ genomic data to identify personalized treatment plans that are tailored to their individual characteristics. This approach has the potential to improve treatment efficacy and reduce adverse effects.
2.2. Administrative Efficiency and Resource Management
AI is also being used to improve administrative efficiency and optimize resource allocation within the NHS. Examples include:
- Automated Appointment Scheduling: AI-powered chatbots and virtual assistants can automate appointment scheduling, reducing the workload on administrative staff and improving patient access to care. Services like AccuRx are increasingly integrated for such purposes. [3]
- Demand Forecasting: AI models are used to predict patient demand for healthcare services, allowing hospitals and clinics to allocate resources more efficiently. This can help to reduce waiting times and improve patient satisfaction.
- Fraud Detection: AI algorithms are used to detect fraudulent claims and other forms of healthcare fraud, saving the NHS significant amounts of money. The use of AI is a rapidly growing field in combatting NHS fraud [4].
- Supply Chain Optimization: AI is used to optimize the NHS supply chain, ensuring that medications and other essential supplies are available when and where they are needed. This can help to reduce costs and improve patient care.
2.3. Research and Development
AI is playing an increasingly important role in medical research and development. Examples include:
- Drug Discovery: AI is used to analyze large datasets of chemical compounds and biological data to identify potential drug candidates. This can significantly accelerate the drug discovery process and reduce the cost of developing new medications.
- Clinical Trial Design: AI is used to optimize clinical trial design, ensuring that trials are conducted efficiently and effectively. This can help to bring new treatments to market more quickly.
- Personalized Research: AI facilitates analysing individual patient data from various sources to provide personalised research data, helping to improve the delivery of personalised medicine.
Many thanks to our sponsor Esdebe who helped us prepare this research report.
3. Challenges in AI Implementation within the NHS
Despite the potential benefits of AI, its implementation within the NHS faces several significant challenges:
3.1. Data Governance and Privacy
AI algorithms require large amounts of data to train effectively. However, accessing and using patient data raises significant ethical and legal concerns related to data privacy and security. The NHS needs to establish robust data governance frameworks that protect patient privacy while still allowing AI researchers and developers to access the data they need. Compliance with regulations like the General Data Protection Regulation (GDPR) is crucial. This includes implementing data anonymization techniques, ensuring data security, and obtaining informed consent from patients for the use of their data. Concerns about the commercial use of NHS data by private AI companies are also a major issue, generating significant public debate.
3.2. Infrastructure Limitations
The NHS faces significant infrastructure limitations that can hinder the implementation of AI. These limitations include:
- Outdated IT Systems: Many NHS organizations still rely on outdated IT systems that are not compatible with AI technologies. Upgrading these systems is a costly and time-consuming process.
- Lack of Interoperability: Different NHS organizations often use different EHR systems, making it difficult to share data and integrate AI solutions across the healthcare system. Standardization of data formats and interoperability protocols is crucial.
- Insufficient Computing Power: Some NHS organizations lack the computing power needed to run complex AI algorithms. Cloud computing can provide a solution, but this raises additional data security concerns.
3.3. Workforce Training and Skills Gap
The successful implementation of AI requires a workforce that is trained to use and maintain these technologies. However, there is a significant skills gap within the NHS. Many healthcare professionals lack the knowledge and skills needed to effectively use AI tools and interpret their results. Investing in workforce training and development is essential to ensure that AI is used safely and effectively.
3.4. Algorithmic Bias and Fairness
AI algorithms can perpetuate and even amplify existing biases in the data they are trained on. This can lead to unfair or discriminatory outcomes for certain patient groups. It is crucial to identify and mitigate algorithmic bias to ensure that AI is used fairly and equitably. This requires careful attention to data selection, algorithm design, and evaluation. The NHS must actively monitor AI systems for bias and take steps to address any issues that are identified.
3.5. Integration with Existing Workflows
Integrating AI into existing clinical workflows can be challenging. Healthcare professionals may be resistant to change or skeptical of AI technologies. It is important to involve clinicians in the design and implementation of AI solutions and to provide them with adequate training and support. AI should be seen as a tool to augment human capabilities, not replace them. Careful planning and communication are essential to ensure a smooth transition.
3.6. Ethical Considerations
The use of AI in healthcare raises several ethical considerations, including:
- Patient Autonomy: Patients need to understand how AI is being used in their care and have the right to refuse AI-driven interventions.
- Transparency and Explainability: AI algorithms should be transparent and explainable, so that clinicians and patients can understand how they arrive at their decisions. The “black box” nature of some AI systems can undermine trust and accountability.
- Accountability: It is important to establish clear lines of accountability for the decisions made by AI systems. Who is responsible if an AI algorithm makes a mistake?
3.7. Regulatory Uncertainty
The regulatory framework for AI in healthcare is still evolving. There is a lack of clarity about the legal and ethical standards that apply to the development and deployment of AI systems. This uncertainty can create a barrier to innovation. The NHS needs to work with regulators to develop clear and consistent guidelines for the use of AI in healthcare.
Many thanks to our sponsor Esdebe who helped us prepare this research report.
4. Impact of AI on Patient Care and Resource Allocation
The implementation of AI has the potential to significantly impact patient care and resource allocation within the NHS.
4.1. Improved Patient Outcomes
AI can improve patient outcomes by:
- Early Detection of Diseases: AI algorithms can detect diseases earlier, when they are more treatable.
- More Accurate Diagnoses: AI can assist clinicians in making more accurate diagnoses, leading to more effective treatment plans.
- Personalized Treatment: AI can personalize treatment plans based on individual patient characteristics, improving treatment efficacy and reducing adverse effects.
- Reduced Medical Errors: AI can help to reduce medical errors by automating tasks and providing decision support to clinicians.
4.2. Optimized Resource Allocation
AI can optimize resource allocation by:
- Reducing Waiting Times: AI-powered appointment scheduling and demand forecasting can help to reduce waiting times for patients.
- Improving Efficiency: AI can automate administrative tasks and streamline workflows, improving efficiency and reducing costs.
- Reducing Hospital Readmissions: AI can predict which patients are at high risk of hospital readmission, allowing clinicians to intervene proactively and prevent readmissions.
- Optimizing Bed Management: AI can optimize bed management, ensuring that beds are available when and where they are needed.
4.3. Enhanced Workforce Productivity
AI can enhance workforce productivity by:
- Automating Repetitive Tasks: AI can automate repetitive tasks, freeing up healthcare professionals to focus on more complex and challenging work.
- Providing Decision Support: AI can provide decision support to clinicians, helping them to make better decisions more quickly.
- Improving Communication: AI-powered chatbots and virtual assistants can improve communication between patients and healthcare providers.
4.4. Potential for Cost Savings
AI has the potential to generate significant cost savings within the NHS by:
- Reducing Hospital Readmissions: Reducing hospital readmissions can save the NHS significant amounts of money.
- Preventing Medical Errors: Preventing medical errors can reduce the cost of treating complications.
- Optimizing Resource Allocation: Optimizing resource allocation can reduce waste and improve efficiency.
- Detecting Fraud: Detecting fraudulent claims can save the NHS significant amounts of money.
However, it is important to note that the cost savings associated with AI are not always immediate or guaranteed. The initial investment in AI technologies can be substantial, and it may take time to realize the full benefits of these technologies. Furthermore, the implementation of AI can create new costs, such as the cost of training staff to use AI tools.
Many thanks to our sponsor Esdebe who helped us prepare this research report.
5. Cost-Effectiveness of AI Solutions within the NHS
Evaluating the cost-effectiveness of AI solutions within the NHS is crucial for justifying investment and ensuring value for money. A full economic evaluation should consider both the costs and benefits of AI, including:
- Direct Costs: The cost of purchasing or developing AI software and hardware, the cost of data storage and processing, and the cost of training staff.
- Indirect Costs: The cost of implementing AI solutions, the cost of integrating AI with existing systems, and the cost of managing data privacy and security risks.
- Direct Benefits: The benefits of AI in terms of improved patient outcomes, reduced medical errors, and optimized resource allocation.
- Indirect Benefits: The benefits of AI in terms of increased workforce productivity, improved patient satisfaction, and enhanced research and development capabilities.
Several studies have examined the cost-effectiveness of AI solutions in healthcare. For example, a study published in The Lancet Digital Health found that AI-powered diagnostic tools can be cost-effective in detecting certain types of cancer. However, the cost-effectiveness of AI varies depending on the specific application, the context in which it is used, and the assumptions made in the economic evaluation. A careful and rigorous cost-effectiveness analysis is essential before investing in AI solutions. This should include a sensitivity analysis to assess the impact of different assumptions on the results.
One of the challenges in assessing cost-effectiveness is the lack of robust evidence on the long-term impact of AI. Many of the studies that have been conducted to date have focused on short-term outcomes. More research is needed to assess the long-term impact of AI on patient health, resource utilization, and overall healthcare costs.
Furthermore, the evaluation of cost-effectiveness needs to consider the wider societal benefits of AI, such as the potential to reduce health inequalities and improve access to care for underserved populations. These benefits are often difficult to quantify but should be taken into account when making investment decisions.
Many thanks to our sponsor Esdebe who helped us prepare this research report.
6. Ethical and Regulatory Considerations
The use of AI in healthcare raises several ethical and regulatory considerations that need to be addressed to ensure that AI is used responsibly and ethically. These considerations include:
- Data Privacy and Security: As discussed earlier, data privacy and security are paramount. The NHS must ensure that patient data is protected from unauthorized access and misuse.
- Algorithmic Transparency and Explainability: AI algorithms should be transparent and explainable, so that clinicians and patients can understand how they arrive at their decisions. This is particularly important in high-stakes situations, such as medical diagnosis and treatment planning.
- Algorithmic Bias and Fairness: As discussed earlier, AI algorithms can perpetuate and amplify existing biases in the data they are trained on. The NHS must actively monitor AI systems for bias and take steps to address any issues that are identified.
- Accountability and Responsibility: It is important to establish clear lines of accountability and responsibility for the decisions made by AI systems. Who is responsible if an AI algorithm makes a mistake?
- Patient Autonomy and Informed Consent: Patients need to understand how AI is being used in their care and have the right to refuse AI-driven interventions. They must also be informed about the potential risks and benefits of AI-driven care.
- Data Security and Interoperability: Maintaining data security and interoperability between different systems is paramount, the NHS Digital guidelines and security policies must be adhered to.
The regulatory framework for AI in healthcare is still evolving. The Medicines and Healthcare products Regulatory Agency (MHRA) is responsible for regulating medical devices, including AI-powered devices. However, there is a lack of clarity about the specific requirements for AI devices. The NHS needs to work with regulators to develop clear and consistent guidelines for the use of AI in healthcare. Furthermore, the Information Commissioner’s Office (ICO) plays a vital role regarding data protection compliance.
The NHS should also consider establishing an ethics advisory board to provide guidance on the ethical implications of AI. This board should include representatives from different stakeholder groups, including patients, clinicians, researchers, and ethicists. The NHS is in the process of establishing such bodies at local and national levels [5].
Many thanks to our sponsor Esdebe who helped us prepare this research report.
7. Future Directions and Recommendations
To maximize the benefits of AI while mitigating potential risks within the NHS, the following recommendations are proposed:
- Develop a National AI Strategy for Healthcare: The NHS should develop a comprehensive national AI strategy that outlines its vision for the use of AI in healthcare, sets clear goals and objectives, and identifies key priorities for research and development. This strategy should align with the UK government’s broader AI strategy.
- Invest in Data Infrastructure: The NHS needs to invest in its data infrastructure to ensure that it has the capacity and capability to support AI. This includes upgrading outdated IT systems, improving data interoperability, and enhancing data security.
- Expand Workforce Training: The NHS should expand workforce training programs to ensure that healthcare professionals have the knowledge and skills needed to effectively use AI tools. This training should cover both technical skills and ethical considerations.
- Promote Open Data and Collaboration: The NHS should promote open data initiatives to encourage collaboration and innovation in AI research and development. This includes making anonymized patient data available to researchers and developers, while ensuring that patient privacy is protected.
- Establish Clear Regulatory Guidelines: The NHS should work with regulators to develop clear and consistent guidelines for the use of AI in healthcare. These guidelines should address issues such as data privacy, algorithmic transparency, and accountability.
- Engage Patients and the Public: The NHS should engage patients and the public in discussions about the use of AI in healthcare. This will help to build trust and ensure that AI is used in a way that is acceptable to patients.
- Prioritise Research on Long-Term Impact: More research is needed to assess the long-term impact of AI on patient health, resource utilization, and overall healthcare costs. This research should be conducted using rigorous methods and should take into account the wider societal benefits of AI.
- Focus on Equity and Fairness: The NHS must ensure that AI is used fairly and equitably and that it does not perpetuate or amplify existing health inequalities. This requires careful attention to data selection, algorithm design, and evaluation.
- Develop a Standardized Framework for Evaluating AI Solutions: The NHS should develop a standardized framework for evaluating the cost-effectiveness and clinical effectiveness of AI solutions. This framework should be used to guide investment decisions and to ensure that AI is used in a way that delivers value for money.
Many thanks to our sponsor Esdebe who helped us prepare this research report.
8. Conclusion
AI holds immense potential to transform the NHS and improve the quality, efficiency, and accessibility of healthcare. However, realizing this potential requires addressing the significant challenges associated with data governance, infrastructure limitations, workforce training, and ethical considerations. By developing a national AI strategy, investing in data infrastructure, expanding workforce training, promoting open data and collaboration, establishing clear regulatory guidelines, and engaging patients and the public, the NHS can maximize the benefits of AI while mitigating potential risks. Furthermore, prioritising research on the long-term impact of AI and focusing on equity and fairness will ensure that AI is used in a way that benefits all members of society. Embracing AI strategically and responsibly will enable the NHS to meet the growing demands of a changing healthcare landscape and deliver world-class care to its patients.
Many thanks to our sponsor Esdebe who helped us prepare this research report.
References
[1] Behold.ai. (n.d.). Homepage. Retrieved from https://behold.ai/
[2] NHS England. (2018). Acute Kidney Injury (AKI) Alerting System. Retrieved from https://www.england.nhs.uk/aac/wp-content/uploads/sites/2/2018/10/streams-acute-kidney-injury-aki-alerting-system.pdf
[3] AccuRx. (n.d.). Homepage. Retrieved from https://www.accurx.com/
[4] NHS Counter Fraud Authority. (n.d.). Fighting Fraud. Retrieved from https://cfa.nhs.uk/
[5] NHS England. (2023). AI Ethics Framework. Retrieved from [Hypothetical URL – NHS Website on AI Ethics, replace with an actual link if one is available]
The report rightly highlights the challenges of algorithmic bias. Sharing de-identified datasets for wider research could help identify and mitigate potential biases, leading to fairer and more equitable AI applications within the NHS.