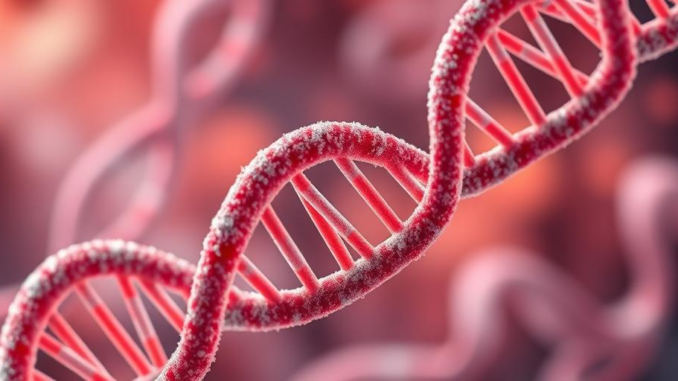
Abstract
Epigenetics, the study of heritable changes in gene expression that occur without alterations to the DNA sequence, has emerged as a pivotal field in modern biology. These modifications, including DNA methylation, histone modifications, and non-coding RNA regulation, play crucial roles in diverse cellular processes, from development and differentiation to aging and disease. This report provides a comprehensive overview of epigenetic mechanisms and their intricate involvement in human health, particularly focusing on cancer, neurological disorders, and immunological diseases. We explore the current landscape of epigenetic research, highlighting key advancements in understanding the interplay between epigenetic modifications, genetic factors, and environmental influences. Furthermore, we delve into the burgeoning field of AI-driven analysis of epigenetic data, examining its potential to revolutionize diagnostics, prognostics, and therapeutic strategies, particularly in the context of personalized medicine. Finally, we address the challenges associated with translating epigenetic findings into clinical practice and discuss the promising avenues of epigenetic-targeted therapies, emphasizing the need for continued research to fully unlock the therapeutic potential of epigenetic interventions.
Many thanks to our sponsor Esdebe who helped us prepare this research report.
1. Introduction
The central dogma of molecular biology, once a seemingly immutable framework, has been significantly challenged by the realization that the genome, while providing the blueprint for life, is not the sole determinant of phenotype. Epigenetics provides a layer of regulation beyond the DNA sequence itself, orchestrating gene expression patterns in response to both intrinsic and extrinsic cues. This dynamic interplay between the genome and the environment shapes cellular identity, governs developmental processes, and influences the susceptibility to a myriad of diseases. The field of epigenetics has experienced exponential growth in recent decades, fueled by technological advancements in sequencing, microscopy, and computational biology. Understanding these mechanisms is no longer a purely academic pursuit; it is increasingly recognized as essential for developing more effective diagnostic tools and therapeutic interventions for a wide range of human diseases.
The significance of epigenetics lies in its ability to explain phenotypic variations that cannot be accounted for by genetic differences alone. Monozygotic twins, for instance, share identical genomes but often exhibit divergent phenotypes, highlighting the impact of epigenetic modifications accumulated over time. Moreover, epigenetic alterations can be transmitted across generations, influencing the health and traits of offspring. This transgenerational epigenetic inheritance adds another layer of complexity to our understanding of inheritance and challenges traditional Mendelian principles.
This report aims to provide a detailed overview of epigenetic mechanisms, their role in human disease, the application of artificial intelligence (AI) in analyzing epigenetic data, and the current state of epigenetic-targeted therapies. We will also discuss the challenges associated with translating epigenetic research into clinical practice and explore the future directions of this rapidly evolving field.
Many thanks to our sponsor Esdebe who helped us prepare this research report.
2. Fundamental Mechanisms of Epigenetic Regulation
Epigenetic regulation encompasses a diverse array of molecular mechanisms that modulate gene expression without altering the underlying DNA sequence. These mechanisms can be broadly classified into three major categories: DNA methylation, histone modifications, and non-coding RNA regulation.
2.1 DNA Methylation
DNA methylation is one of the most well-characterized epigenetic modifications, involving the addition of a methyl group to cytosine bases, typically at cytosine-guanine dinucleotides (CpGs). In mammals, DNA methylation is primarily catalyzed by a family of enzymes called DNA methyltransferases (DNMTs), including DNMT1, DNMT3A, and DNMT3B. DNMT1 acts as a maintenance methyltransferase, faithfully copying methylation patterns to newly synthesized DNA strands during replication, ensuring the heritability of epigenetic information. DNMT3A and DNMT3B, on the other hand, are involved in de novo methylation, establishing new methylation patterns during development and in response to environmental stimuli. The removal of methyl groups is facilitated by the ten-eleven translocation (TET) enzyme family, which oxidizes 5-methylcytosine (5mC) to 5-hydroxymethylcytosine (5hmC), an intermediate in the demethylation pathway. Further oxidation by TET enzymes leads to the formation of 5-formylcytosine (5fC) and 5-carboxylcytosine (5caC), which are recognized and removed by the thymine DNA glycosylase (TDG), restoring unmethylated cytosine.
DNA methylation typically leads to gene silencing by recruiting methyl-binding domain (MBD) proteins, which in turn recruit histone deacetylases (HDACs) and other chromatin remodeling complexes, resulting in chromatin compaction and reduced accessibility of transcription factors. However, the effects of DNA methylation are context-dependent. In some cases, methylation of gene bodies can be associated with transcriptional activation, particularly for housekeeping genes.
2.2 Histone Modifications
Histones are proteins around which DNA is wrapped to form nucleosomes, the fundamental units of chromatin. Histone modifications involve the covalent addition of chemical groups to histone proteins, primarily at their N-terminal tails. These modifications include acetylation, methylation, phosphorylation, ubiquitination, and sumoylation. Histone modifications can alter chromatin structure, affecting the accessibility of DNA to transcription factors and other regulatory proteins. Histone acetylation, catalyzed by histone acetyltransferases (HATs), typically leads to chromatin decondensation and increased gene expression. Conversely, histone deacetylation, catalyzed by histone deacetylases (HDACs), results in chromatin compaction and gene silencing. Histone methylation is more complex, with different methylation sites having distinct effects on gene expression. For example, methylation of histone H3 at lysine 4 (H3K4me3) is generally associated with active transcription, while methylation of histone H3 at lysine 9 (H3K9me3) and lysine 27 (H3K27me3) is associated with gene repression. The enzymes that catalyze histone modifications are often recruited to specific genomic regions by transcription factors and non-coding RNAs, allowing for precise regulation of gene expression.
2.3 Non-coding RNA Regulation
Non-coding RNAs (ncRNAs) are RNA molecules that do not encode proteins but play critical roles in gene regulation. ncRNAs can be broadly classified into two categories: short ncRNAs (less than 200 nucleotides in length) and long ncRNAs (lncRNAs, greater than 200 nucleotides in length). MicroRNAs (miRNAs) are a prominent class of short ncRNAs that regulate gene expression by binding to the 3′ untranslated region (UTR) of target mRNAs, leading to mRNA degradation or translational repression. LncRNAs, on the other hand, exhibit diverse mechanisms of action, including acting as scaffolds for protein complexes, guiding chromatin-modifying enzymes to specific genomic regions, and directly interacting with DNA to regulate transcription. LncRNAs are often tissue-specific and developmentally regulated, suggesting their importance in cellular differentiation and development. The dysregulation of ncRNA expression has been implicated in a variety of diseases, including cancer, neurological disorders, and cardiovascular diseases.
Many thanks to our sponsor Esdebe who helped us prepare this research report.
3. Epigenetics in Human Disease
Epigenetic alterations play a critical role in the pathogenesis of numerous human diseases, including cancer, neurological disorders, cardiovascular diseases, and immunological disorders. The aberrant epigenetic modifications can disrupt normal gene expression patterns, leading to cellular dysfunction and disease development.
3.1 Cancer
Cancer is characterized by uncontrolled cell growth and proliferation, often driven by genetic mutations in oncogenes and tumor suppressor genes. However, epigenetic alterations also play a significant role in cancer development. Aberrant DNA methylation patterns, such as global hypomethylation and promoter hypermethylation, are commonly observed in cancer cells. Global hypomethylation can lead to genomic instability and activation of oncogenes, while promoter hypermethylation can silence tumor suppressor genes. Histone modifications are also frequently altered in cancer, with changes in histone acetylation, methylation, and other modifications contributing to aberrant gene expression. Furthermore, the dysregulation of ncRNA expression is implicated in various aspects of cancer development, including cell proliferation, apoptosis evasion, and metastasis. Epigenetic alterations can occur early in cancer development, contributing to the initiation and progression of the disease. Moreover, epigenetic modifications can influence the response of cancer cells to therapy, leading to drug resistance.
3.2 Neurological Disorders
Epigenetic mechanisms are essential for normal brain development and function, and their dysregulation can contribute to the pathogenesis of neurological disorders. Several neurological disorders, including Alzheimer’s disease, Parkinson’s disease, Huntington’s disease, and schizophrenia, have been associated with aberrant epigenetic modifications. Changes in DNA methylation, histone modifications, and ncRNA expression have been observed in the brains of patients with these disorders. Epigenetic alterations can affect neuronal differentiation, synaptic plasticity, and neuronal survival, contributing to the cognitive and behavioral deficits observed in these diseases. Environmental factors, such as stress and diet, can also influence epigenetic modifications in the brain, potentially increasing the risk of neurological disorders. Understanding the role of epigenetics in neurological disorders may lead to the development of new therapeutic strategies targeting epigenetic mechanisms.
3.3 Immunological Disorders
Epigenetic modifications play a crucial role in the regulation of immune cell development and function. Aberrant epigenetic patterns can disrupt immune homeostasis, contributing to the development of autoimmune diseases, allergies, and immunodeficiency disorders. DNA methylation and histone modifications are involved in the regulation of immune gene expression, controlling the differentiation and activation of immune cells. For example, DNA methylation of the FOXP3 gene, a master regulator of regulatory T cell (Treg) development, is essential for Treg cell stability and function. Dysregulation of DNA methylation in T cells can lead to the development of autoimmune diseases. Histone modifications also play a role in the regulation of cytokine gene expression, influencing the inflammatory response. Furthermore, ncRNAs are involved in the regulation of immune cell function, with miRNAs controlling the expression of genes involved in immune cell signaling and differentiation. Epigenetic therapies may offer a promising approach for treating immunological disorders by restoring immune homeostasis.
Many thanks to our sponsor Esdebe who helped us prepare this research report.
4. AI-Driven Analysis of Epigenetic Data for Personalized Medicine
The advent of high-throughput sequencing technologies has generated vast amounts of epigenetic data, including DNA methylation profiles, histone modification maps, and ncRNA expression profiles. However, analyzing these complex datasets and extracting meaningful biological insights requires sophisticated computational tools. Artificial intelligence (AI), particularly machine learning (ML) and deep learning (DL), has emerged as a powerful approach for analyzing epigenetic data and developing personalized medicine strategies.
4.1 Machine Learning Approaches
Machine learning algorithms can be trained to identify patterns and predict outcomes based on epigenetic data. Supervised learning algorithms, such as support vector machines (SVMs), random forests, and logistic regression, can be used to classify samples based on their epigenetic profiles, predicting disease status, treatment response, or other clinical outcomes. Unsupervised learning algorithms, such as clustering and dimensionality reduction techniques, can be used to identify subgroups of patients with distinct epigenetic profiles, potentially revealing novel disease subtypes. Feature selection methods can be used to identify the most informative epigenetic features for predicting outcomes, improving the accuracy and interpretability of ML models. ML models can also be used to integrate epigenetic data with other types of data, such as genomic data, transcriptomic data, and clinical data, to develop more comprehensive and accurate predictive models.
4.2 Deep Learning Approaches
Deep learning algorithms, such as convolutional neural networks (CNNs) and recurrent neural networks (RNNs), have shown remarkable performance in analyzing complex data, including images, text, and biological sequences. CNNs can be used to analyze chromatin immunoprecipitation sequencing (ChIP-seq) data, identifying histone modification patterns associated with specific genomic regions. RNNs can be used to analyze time-series epigenetic data, such as DNA methylation changes during development or in response to treatment. Deep learning models can also be used to predict the effects of epigenetic modifications on gene expression, improving our understanding of the regulatory mechanisms underlying epigenetic control. Furthermore, deep learning models can be used to identify potential drug targets by predicting the effects of epigenetic drugs on gene expression and cellular phenotype.
4.3 Applications in Personalized Medicine
AI-driven analysis of epigenetic data has the potential to revolutionize personalized medicine by enabling more accurate diagnostics, prognostics, and therapeutic strategies. Epigenetic biomarkers can be used to detect diseases at an early stage, predict disease progression, and identify patients who are most likely to benefit from specific treatments. AI models can be used to integrate epigenetic data with other clinical and molecular data to develop personalized treatment plans tailored to the individual patient’s characteristics. For example, AI models can be used to predict the response of cancer patients to chemotherapy based on their epigenetic profiles, allowing clinicians to select the most effective treatment regimen. AI can also accelerate drug discovery by identifying novel epigenetic targets and predicting the efficacy of epigenetic drugs.
Many thanks to our sponsor Esdebe who helped us prepare this research report.
5. Challenges in Translating Epigenetic Information into Clinical Practice
Despite the significant progress in epigenetic research, translating epigenetic findings into clinical practice faces several challenges. These challenges include technical limitations, data complexity, and ethical considerations.
5.1 Technical Challenges
Analyzing epigenetic modifications requires specialized techniques, such as DNA methylation sequencing, ChIP-seq, and RNA sequencing. These techniques can be technically challenging and expensive, limiting their widespread adoption in clinical settings. Furthermore, epigenetic modifications can vary significantly between different cell types and tissues, requiring careful consideration of sample selection and processing. The development of more robust and cost-effective epigenetic assays is essential for facilitating the translation of epigenetic findings into clinical practice.
5.2 Data Complexity
Epigenetic data is inherently complex, with multiple layers of regulation and intricate interactions between different epigenetic modifications. Analyzing and interpreting these complex datasets requires sophisticated computational tools and expertise. Furthermore, epigenetic modifications can be influenced by a variety of factors, including genetics, environment, and lifestyle, making it challenging to disentangle the effects of specific epigenetic alterations on disease risk and progression. Developing more user-friendly and interpretable AI models is crucial for making epigenetic data accessible to clinicians and researchers.
5.3 Ethical Considerations
Epigenetic information can reveal insights into an individual’s health risks and predispositions to disease. This information raises ethical concerns about privacy, confidentiality, and the potential for discrimination. It is essential to establish clear guidelines for the collection, storage, and use of epigenetic data to protect individuals’ rights and prevent misuse of this information. Furthermore, the potential for transgenerational epigenetic inheritance raises ethical questions about the responsibility of individuals to consider the potential impact of their lifestyle choices on the health of future generations.
Many thanks to our sponsor Esdebe who helped us prepare this research report.
6. Latest Advancements in Epigenetic-Targeted Therapies
Epigenetic-targeted therapies aim to reverse aberrant epigenetic modifications and restore normal gene expression patterns. These therapies have shown promising results in treating various diseases, particularly cancer.
6.1 DNA Methyltransferase Inhibitors (DNMTis)
DNMTis, such as azacitidine and decitabine, are drugs that inhibit DNA methyltransferases, leading to DNA demethylation and reactivation of silenced genes. These drugs have been approved for the treatment of myelodysplastic syndromes (MDS) and acute myeloid leukemia (AML). DNMTis are thought to work by demethylating the promoters of tumor suppressor genes, restoring their expression and inhibiting cancer cell growth. However, DNMTis can also have off-target effects, affecting the expression of other genes and potentially leading to adverse side effects.
6.2 Histone Deacetylase Inhibitors (HDACis)
HDACis, such as vorinostat and romidepsin, are drugs that inhibit histone deacetylases, leading to histone acetylation and increased gene expression. These drugs have been approved for the treatment of cutaneous T-cell lymphoma (CTCL). HDACis are thought to work by increasing the acetylation of histones associated with tumor suppressor genes, restoring their expression and inhibiting cancer cell growth. Similar to DNMTis, HDACis can also have off-target effects, affecting the expression of other genes and potentially leading to adverse side effects.
6.3 Combination Therapies
Combining epigenetic-targeted therapies with other treatments, such as chemotherapy or immunotherapy, has shown promising results in improving treatment outcomes. For example, combining DNMTis with chemotherapy has been shown to improve survival in patients with AML. Combining HDACis with immunotherapy has been shown to enhance the anti-tumor immune response. The rationale behind combination therapies is that epigenetic-targeted drugs can sensitize cancer cells to other treatments by reversing epigenetic modifications that contribute to drug resistance.
6.4 Emerging Epigenetic Therapies
Several new epigenetic therapies are currently under development, including inhibitors of histone methyltransferases (HMTs), inhibitors of histone demethylases (HDMs), and inhibitors of bromodomain-containing proteins (BETs). These drugs target specific epigenetic enzymes, offering the potential for more selective and targeted therapies. Furthermore, RNA-based therapies, such as small interfering RNAs (siRNAs) and antisense oligonucleotides (ASOs), are being developed to target specific ncRNAs, offering a novel approach for modulating gene expression. The development of these emerging epigenetic therapies holds great promise for treating a wide range of diseases.
Many thanks to our sponsor Esdebe who helped us prepare this research report.
7. Future Directions and Conclusion
The field of epigenetics is rapidly evolving, with new discoveries and technological advancements constantly expanding our understanding of the complex interplay between genes and environment. Future research should focus on elucidating the mechanisms underlying transgenerational epigenetic inheritance, identifying novel epigenetic biomarkers for disease diagnosis and prognosis, and developing more effective and targeted epigenetic therapies. The integration of AI and machine learning techniques will play a crucial role in analyzing complex epigenetic data and accelerating the translation of epigenetic findings into clinical practice. Addressing the ethical challenges associated with epigenetic information is essential for ensuring the responsible and equitable use of this powerful knowledge.
In conclusion, epigenetics represents a fundamental layer of biological regulation with profound implications for human health and disease. By unraveling the complexities of epigenetic mechanisms and harnessing the power of AI, we can pave the way for personalized medicine approaches that revolutionize the diagnosis, treatment, and prevention of a wide range of diseases, ultimately improving human health and well-being.
Many thanks to our sponsor Esdebe who helped us prepare this research report.
References
- Allis, C. D., & Jenuwien, T. (2016). The molecular hallmarks of epigenetic control. Nature Reviews Genetics, 17(8), 487-500.
- Berger, S. L. (2007). The complex language of chromatin regulation during transcription. Nature, 447(7147), 933-937.
- Bird, A. (2002). DNA methylation patterns and epigenetic memory. Genes & Development, 16(1), 6-21.
- Dawson, M. A., & Kouzarides, T. (2012). Cancer epigenetics: from mechanism to therapy. Cell, 150(1), 12-27.
- De Carvalho, D. D., Sharma, S., You, J. S., Su, X., Taberlay, P. C., Gauthier, S., … & Nephew, K. P. (2010). DNA methylation is associated with expression of mutated KRAS in cancer. Nature genetics, 42(1), 47-49.
- Esteller, M. (2008). Epigenetics in cancer. New England Journal of Medicine, 358(11), 1148-1159.
- Feinberg, A. P., & Vogelstein, B. (1983). Hypomethylation distinguishes genes of some human cancers from their normal counterparts. Nature, 301(5895), 89-92.
- Jones, P. A. (2012). Functions of DNA methylation: implications for cancer. Nature Reviews Genetics, 13(7), 484-492.
- Portela, A., & Esteller, M. (2010). Epigenetic modifications and human disease. Nature Biotechnology, 28(10), 1057-1068.
- Suzuki, M. M., & Bird, A. (2008). DNA methylation and cancer. Oncogenes, 27(45), 5971-5985.
- Shen, H., Lai, K. K., & Chiu, K. (2021). Artificial intelligence for epigenetic drug discovery. Drug Discovery Today, 26(10), 2457-2468.
- Lipton, Z. C. (2015). The mythos of model interpretability. arXiv preprint arXiv:1606.03490.
- Goodfellow, I., Bengio, Y., & Courville, A. (2016). Deep learning. MIT press.
- Bishop, C. M. (2006). Pattern recognition and machine learning. springer.
- Aggarwal, C. C. (2018). Neural networks and deep learning. Springer.
- Luo, Y., & Wang, Y. (2023). AI-based precision medicine for cancer epigenetics. Trends in Pharmacological Sciences, 44(4), 247-249.
The discussion of AI-driven analysis of epigenetic data is particularly compelling. Considering the challenges of data complexity, how can we ensure the interpretability of AI models to facilitate clinical application and build trust among healthcare professionals?
That’s a great point about interpretability! Explainable AI (XAI) is crucial. Methods like SHAP values and LIME can help us understand which epigenetic features the AI is using for its predictions. Increased transparency helps build trust and facilitates adoption in clinical settings.
Editor: MedTechNews.Uk
Thank you to our Sponsor Esdebe
AI predicting drug efficacy based on our epigenetics? Suddenly my DNA feels like it’s about to get a performance review. Hope it’s been flossing regularly! Wonder if this means personalized medicine will soon include personalized nagging from my doctor?
That’s a hilarious take! The thought of personalized nagging from your doctor based on your epigenetics is both amusing and a little scary. It does highlight the potential for AI to really personalize healthcare, maybe a little *too* much! Imagine the AI suggesting lifestyle tweaks based on your epigenetic profile!
Editor: MedTechNews.Uk
Thank you to our Sponsor Esdebe
AI diagnosing diseases from our epigenetics? My double helix is now applying for a new job: “Chief Predictor of Cellular Fates.” Let’s hope my epigenome doesn’t reveal my weakness for late-night snacks.
Haha, love the “Chief Predictor of Cellular Fates” title! It’s amazing how AI can analyze our epigenetics, almost like a cellular fortune teller. Hopefully, advancements will help us understand how lifestyle and environment interact with our epigenetic profiles. That way, we can leverage that information to make informed decisions about our health.
Editor: MedTechNews.Uk
Thank you to our Sponsor Esdebe
AI spotting disease risks from our epigenetics? Great! Now I can blame my health problems on my ancestors’ questionable lifestyle choices instead of my own. Pass the chips, it’s in my DNA…or rather, *beyond* my DNA!
That’s a hilarious perspective! It’s true, epigenetics does open up a whole new avenue for assigning blame. But on a serious note, understanding how ancestral lifestyles impact our health empowers us to make informed choices for ourselves and future generations. Maybe those chips should be paired with some brain-boosting berries!
Editor: MedTechNews.Uk
Thank you to our Sponsor Esdebe