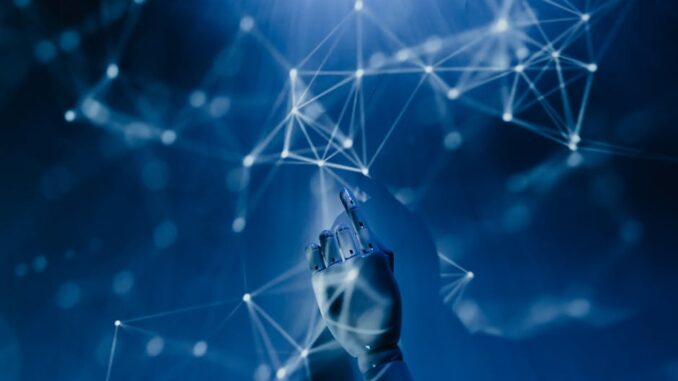
Abstract
Artificial Intelligence (AI) is rapidly transforming various aspects of modern society, offering unprecedented opportunities for innovation and progress. However, the development, deployment, and increasing reliance on AI systems pose significant environmental challenges. This research report provides a comprehensive assessment of the multifaceted environmental impacts associated with AI, moving beyond the often-cited energy consumption and e-waste issues. We delve into the less-explored areas of resource depletion, the carbon footprint of AI-driven manufacturing, the ethical implications of AI in environmental decision-making, and the potential for AI to exacerbate existing environmental inequalities. Furthermore, we critically examine the trade-offs between AI-driven environmental solutions and their own ecological costs. Finally, we analyze the effectiveness of current mitigation strategies, including technological advancements, policy interventions, and international collaborations, and propose a framework for a more sustainable and equitable AI ecosystem. This report aims to provide a holistic understanding of the environmental footprint of AI for experts, policymakers, and researchers.
Many thanks to our sponsor Esdebe who helped us prepare this research report.
1. Introduction
The pervasive influence of Artificial Intelligence (AI) is undeniable. From autonomous vehicles and personalized medicine to climate modeling and resource management, AI applications are reshaping our world at an accelerating pace. The promise of AI lies in its potential to optimize processes, enhance decision-making, and unlock new frontiers of knowledge. However, this transformative power comes with a hidden cost: a growing and increasingly complex environmental burden.
While the energy consumption of data centers and the proliferation of electronic waste (e-waste) have garnered considerable attention, these represent only the tip of the iceberg. A comprehensive assessment of the environmental impact of AI must consider a broader spectrum of factors, including the extraction and processing of raw materials for AI hardware, the carbon footprint of AI-driven manufacturing processes, the indirect impacts of AI on consumption patterns, and the potential for algorithmic bias to exacerbate environmental injustices.
This report argues that a myopic focus on energy efficiency and e-waste management is insufficient to address the environmental challenges posed by AI. A holistic and systems-level approach is needed, one that considers the entire lifecycle of AI systems, from conception to disposal, and that acknowledges the complex interdependencies between AI technologies, human behavior, and the natural environment. The goal of this report is to provide such a comprehensive assessment, identifying key areas of concern, evaluating the effectiveness of existing mitigation strategies, and proposing a framework for a more sustainable and equitable AI future.
Many thanks to our sponsor Esdebe who helped us prepare this research report.
2. Resource Depletion and AI Hardware
The foundation of AI lies in sophisticated hardware: processors, memory chips, and sensors. The manufacturing of these components is a resource-intensive process, demanding significant quantities of rare earth elements, precious metals, and other critical materials. Mining these materials can lead to deforestation, soil degradation, water pollution, and biodiversity loss. Furthermore, the geopolitical implications of relying on a limited number of countries for these resources are significant, creating potential vulnerabilities in the global AI supply chain.
Consider, for example, the demand for lithium, a key component of batteries used in mobile devices and data centers. The extraction of lithium from brine deposits in South America has been linked to water scarcity and ecosystem disruption. Similarly, the mining of cobalt in the Democratic Republic of Congo, often associated with unethical labor practices, raises serious concerns about social and environmental justice.
While efforts are underway to develop alternative materials and improve recycling processes, the current trajectory suggests that the demand for these resources will continue to increase as AI systems become more prevalent. This highlights the need for a circular economy approach to AI hardware, one that prioritizes resource efficiency, reuse, and responsible disposal. Innovative approaches, such as developing AI algorithms that can run on less specialized hardware, or designing hardware that is easier to disassemble and recycle, are crucial for mitigating the resource depletion associated with AI.
Furthermore, the energy required to manufacture AI hardware is often overlooked. The fabrication of semiconductors, in particular, is an energy-intensive process that relies heavily on fossil fuels. Reducing the energy footprint of hardware manufacturing is therefore essential for minimizing the overall environmental impact of AI. This requires investments in renewable energy sources, energy-efficient manufacturing processes, and the development of less energy-intensive materials.
Many thanks to our sponsor Esdebe who helped us prepare this research report.
3. The Carbon Footprint of AI: Beyond Data Centers
The energy consumption of data centers, which power AI training and inference, is a well-documented environmental concern. Large AI models require vast amounts of computational power, leading to significant electricity consumption and greenhouse gas emissions. However, the carbon footprint of AI extends beyond data centers. A more holistic assessment must consider the energy used in the entire lifecycle of AI systems, including:
- Research and Development: The development of new AI algorithms and architectures requires significant computational resources, contributing to the overall carbon footprint.
- Manufacturing: The energy used to manufacture AI hardware, as discussed in the previous section, is a significant component of the carbon footprint.
- Deployment and Use: The energy consumption of AI-powered devices and applications, such as smartphones, autonomous vehicles, and smart home devices, also contributes to the overall carbon footprint.
- Disposal and Recycling: The end-of-life management of AI hardware generates e-waste, which can release harmful pollutants into the environment and require further energy for processing and recycling.
Furthermore, the indirect impacts of AI on energy consumption should not be ignored. For example, AI-powered advertising algorithms can drive increased consumption of goods and services, leading to higher overall energy demand. Similarly, AI-enabled automation can increase the efficiency of industrial processes, but it can also lead to increased production and consumption, offsetting some of the energy savings.
To accurately assess the carbon footprint of AI, life cycle assessments (LCAs) are needed that consider all stages of the AI system’s lifecycle. These LCAs should account for both direct and indirect energy consumption, as well as the environmental impacts of resource extraction, manufacturing, and disposal. The development of standardized methodologies for conducting LCAs of AI systems is crucial for promoting transparency and accountability.
Moreover, the adoption of renewable energy sources to power data centers and AI hardware manufacturing facilities is essential for reducing the carbon footprint of AI. Governments and corporations should incentivize the use of renewable energy and invest in research and development of more energy-efficient AI algorithms and hardware.
Many thanks to our sponsor Esdebe who helped us prepare this research report.
4. Water Usage and the AI Industry
Water scarcity is a growing global challenge, and the AI industry contributes to this problem through the significant water usage of data centers. Data centers require large amounts of water for cooling, particularly in arid and semi-arid regions. The evaporation of water from cooling towers can deplete local water resources, impacting agriculture, ecosystems, and human health.
The water footprint of AI is often overlooked in discussions of its environmental impact. However, the increasing demand for AI is exacerbating water scarcity in many parts of the world. Consider, for example, the impact of large data centers located in water-stressed regions. These facilities can consume millions of gallons of water per day, putting a strain on local water resources and potentially leading to conflicts over water rights.
To mitigate the water footprint of AI, several strategies can be employed:
- Water-Efficient Cooling Technologies: The development and deployment of water-efficient cooling technologies, such as dry cooling systems and advanced evaporative cooling systems, can significantly reduce water consumption in data centers.
- Data Center Location: Choosing locations with abundant water resources or using alternative cooling methods, such as air cooling or immersion cooling, can minimize the impact on water scarcity.
- Water Recycling and Reuse: Implementing water recycling and reuse programs in data centers can reduce the demand for freshwater.
- AI-Driven Water Management: AI can also be used to optimize water management in data centers, reducing water consumption and improving efficiency.
Furthermore, it is important to consider the water footprint of AI hardware manufacturing. The production of semiconductors and other electronic components requires significant amounts of water for cleaning and etching processes. Reducing water consumption in hardware manufacturing is therefore essential for minimizing the overall water footprint of AI.
Many thanks to our sponsor Esdebe who helped us prepare this research report.
5. E-Waste: A Growing Threat Amplified by AI
The rapid obsolescence of AI hardware and the increasing demand for new devices are contributing to a growing global e-waste problem. E-waste contains hazardous materials, such as lead, mercury, and cadmium, which can contaminate soil, water, and air if not properly managed. The informal recycling sector, which often handles e-waste in developing countries, poses significant risks to human health and the environment.
AI is accelerating the rate of e-waste generation in several ways:
- Increased Demand for Hardware: The growing demand for AI is driving increased production of electronic devices, leading to a larger volume of e-waste at the end of their lifecycle.
- Shorter Lifecycles: The rapid pace of technological innovation in AI is leading to shorter lifecycles for AI hardware, increasing the rate of e-waste generation.
- Embedded AI: The integration of AI into a wide range of products, from appliances to vehicles, is increasing the complexity and volume of e-waste streams.
To address the e-waste challenge, a multi-pronged approach is needed:
- Extended Producer Responsibility (EPR): Implementing EPR schemes that hold manufacturers responsible for the end-of-life management of their products can incentivize them to design more durable, repairable, and recyclable devices.
- Improved Recycling Infrastructure: Investing in modern e-waste recycling infrastructure, particularly in developing countries, can ensure that e-waste is processed in an environmentally sound manner.
- Promoting Circular Economy Principles: Encouraging the reuse, repair, and refurbishment of AI hardware can reduce the demand for new devices and minimize e-waste generation.
- Developing Less Hazardous Materials: Researching and developing alternative materials that are less hazardous and easier to recycle can reduce the environmental impact of e-waste.
AI can also play a role in improving e-waste management. AI-powered robotics can be used to automate the sorting and dismantling of e-waste, improving efficiency and reducing human exposure to hazardous materials. AI algorithms can also be used to identify and track e-waste streams, helping to prevent illegal dumping and improve recycling rates.
Many thanks to our sponsor Esdebe who helped us prepare this research report.
6. The Ethical Dimensions: Algorithmic Bias and Environmental Justice
AI algorithms are trained on data, and if that data reflects existing societal biases, the resulting algorithms can perpetuate and even amplify those biases. In the context of environmental decision-making, algorithmic bias can lead to unjust outcomes, disproportionately impacting marginalized communities and exacerbating environmental inequalities.
For example, AI-powered risk assessment tools used by environmental agencies may underestimate the risks faced by low-income communities or communities of color, leading to inadequate environmental protection. Similarly, AI-driven resource allocation models may favor wealthier communities, further disadvantaging those already facing environmental burdens.
To ensure that AI is used ethically in environmental decision-making, it is crucial to address algorithmic bias. This requires:
- Data Diversity: Ensuring that training data is representative of all segments of the population and that it does not reflect existing societal biases.
- Transparency and Explainability: Developing AI algorithms that are transparent and explainable, allowing stakeholders to understand how decisions are being made and to identify potential biases.
- Stakeholder Engagement: Engaging with communities affected by environmental decisions to ensure that their voices are heard and that their concerns are addressed.
- Independent Audits: Conducting independent audits of AI algorithms to identify and mitigate potential biases.
Furthermore, it is important to consider the social and economic impacts of AI-driven environmental solutions. For example, AI-powered automation of agricultural processes may lead to job losses in rural communities. Policymakers must consider these potential impacts and implement measures to mitigate them, such as providing retraining and support for displaced workers.
Many thanks to our sponsor Esdebe who helped us prepare this research report.
7. AI for Environmental Sustainability: A Double-Edged Sword
AI offers tremendous potential for addressing environmental challenges, such as climate change, pollution, and resource depletion. However, it is important to recognize that AI-driven environmental solutions can also have unintended consequences and that their effectiveness depends on careful planning and implementation.
For example, AI-powered smart grids can optimize energy consumption and reduce greenhouse gas emissions. However, the deployment of smart grids requires significant investments in infrastructure and can potentially increase cybersecurity risks. Similarly, AI-driven precision agriculture can reduce the use of fertilizers and pesticides, but it can also lead to increased reliance on technology and potentially displace small farmers.
To maximize the benefits of AI for environmental sustainability while minimizing the risks, it is crucial to:
- Adopt a Systems-Level Approach: Consider the entire lifecycle of AI-driven environmental solutions, from conception to disposal, and assess their potential impacts on the environment, society, and the economy.
- Promote Transparency and Accountability: Ensure that AI algorithms used for environmental decision-making are transparent and accountable, and that stakeholders have access to the data and models used to make those decisions.
- Engage with Communities: Engage with communities affected by AI-driven environmental solutions to ensure that their voices are heard and that their concerns are addressed.
- Prioritize Equity and Justice: Ensure that AI-driven environmental solutions do not exacerbate existing environmental inequalities and that they benefit all segments of the population.
Many thanks to our sponsor Esdebe who helped us prepare this research report.
8. Policy Interventions and International Collaboration
Addressing the environmental impact of AI requires a combination of technological innovation, policy interventions, and international collaboration. Governments and international organizations can play a crucial role in setting standards, promoting best practices, and incentivizing the development and deployment of sustainable AI technologies.
Key policy interventions include:
- Carbon Pricing: Implementing carbon pricing mechanisms, such as carbon taxes or cap-and-trade systems, can incentivize the reduction of greenhouse gas emissions from AI systems.
- Energy Efficiency Standards: Setting energy efficiency standards for data centers and AI hardware can reduce energy consumption and promote the adoption of more efficient technologies.
- E-Waste Regulations: Strengthening e-waste regulations and promoting EPR schemes can improve e-waste management and reduce the environmental impact of electronic devices.
- Water Efficiency Standards: Setting water efficiency standards for data centers and promoting the adoption of water-efficient cooling technologies can reduce water consumption.
- Investing in Research and Development: Investing in research and development of sustainable AI technologies, such as energy-efficient algorithms, renewable energy sources, and alternative materials, can accelerate the transition to a more sustainable AI ecosystem.
International collaboration is also essential for addressing the global environmental challenges posed by AI. This includes:
- Sharing Best Practices: Sharing best practices for sustainable AI development and deployment can help countries learn from each other and accelerate the adoption of more sustainable technologies.
- Developing International Standards: Developing international standards for environmental reporting and life cycle assessment of AI systems can promote transparency and accountability.
- Addressing E-Waste Trade: Addressing the illegal trade in e-waste and promoting responsible e-waste management in developing countries can reduce the environmental and health risks associated with e-waste.
- Promoting Equitable Access to Technology: Promoting equitable access to AI technologies and ensuring that developing countries benefit from the opportunities offered by AI can help to reduce environmental inequalities.
Many thanks to our sponsor Esdebe who helped us prepare this research report.
9. Conclusion: Towards a Sustainable and Equitable AI Future
The environmental impact of AI is a complex and multifaceted issue that requires a holistic and systems-level approach. While AI offers tremendous potential for addressing environmental challenges, it is important to recognize that AI-driven solutions can also have unintended consequences and that their effectiveness depends on careful planning and implementation. The current focus on energy consumption and e-waste, while important, is insufficient to capture the full scope of the environmental burden associated with AI.
To move towards a more sustainable and equitable AI future, we need to:
- Expand the Scope of Assessment: Broaden the scope of environmental impact assessments to include resource depletion, water usage, algorithmic bias, and the social and economic impacts of AI.
- Promote Transparency and Accountability: Ensure that AI algorithms used for environmental decision-making are transparent and accountable, and that stakeholders have access to the data and models used to make those decisions.
- Engage with Communities: Engage with communities affected by AI-driven environmental solutions to ensure that their voices are heard and that their concerns are addressed.
- Prioritize Equity and Justice: Ensure that AI-driven environmental solutions do not exacerbate existing environmental inequalities and that they benefit all segments of the population.
- Invest in Sustainable Technologies: Invest in research and development of sustainable AI technologies, such as energy-efficient algorithms, renewable energy sources, and alternative materials.
- Implement Policy Interventions: Implement policy interventions, such as carbon pricing, energy efficiency standards, and e-waste regulations, to incentivize the development and deployment of sustainable AI technologies.
- Foster International Collaboration: Foster international collaboration to share best practices, develop international standards, and address the global environmental challenges posed by AI.
By taking these steps, we can harness the transformative power of AI while minimizing its environmental footprint and ensuring that its benefits are shared by all.
Many thanks to our sponsor Esdebe who helped us prepare this research report.
References
- Bates, J. L., Fawcett, D., McAllister, M. L., McLaren, D., & Smith, G. P. (2020). Energy and policy considerations for deep learning in NLP. Transactions of the Association for Computational Linguistics, 8, 1468-1482.
- Belkhir, L., & Elmeligi, A. (2019). Assessing ICT global emissions footprint: Trends and projections. Journal of Cleaner Production, 177, 448-463.
- European Commission. (2020). White Paper on Artificial Intelligence: A European approach to excellence and trust. Brussels: European Commission.
- Hilty, L. M., Aebischer, B., & Ruddy, T. F. (2006). ICT and sustainability: Towards a sustainable information society. Swiss Federal Laboratories for Materials Testing and Research.
- Strubell, E., Ganesh, A., & McCallum, A. (2019). Energy and policy considerations for deep learning in NLP. arXiv preprint arXiv:1906.02243.
- United Nations Environment Programme. (2019). Global E-waste Monitor 2019. Bonn/Geneva.
- Wu, J., Ho, M. S., & Li, Q. (2017). Environmental impacts of mining rare earth elements in China. Environmental Science & Technology, 51(6), 3238-3245.
- Luukkanen, J., Toivio, H., Kanerva, M., Kolehmainen, M., & Raugei, M. (2023). Environmental impacts of information and communication technology sector: A review of life cycle assessments. Journal of Cleaner Production, 382, 135383.
- Oliveira Neto, G. C., & Wankhade, P. (2023). Water footprint assessment of data centers: a systematic review. Environmental Science and Pollution Research, 30(2), 3176-3193.
- Akinwale, Y. W., Lindersson, S., Avtar, R., & Strohmeier, P. (2024). The carbon footprint of artificial intelligence: A scoping review. Energy and AI, 15, 100306.
The report’s discussion on algorithmic bias and environmental justice is vital. Further research into how AI can actively promote equitable resource allocation and mitigate existing environmental disparities could offer actionable solutions.
Thanks for highlighting the importance of algorithmic bias and environmental justice! I agree, and I believe exploring how AI can *proactively* address these disparities is crucial. For example, could AI optimize resource distribution based on vulnerability factors to achieve more equitable outcomes? It is a fascinating and pressing issue.
Editor: MedTechNews.Uk
Thank you to our Sponsor Esdebe