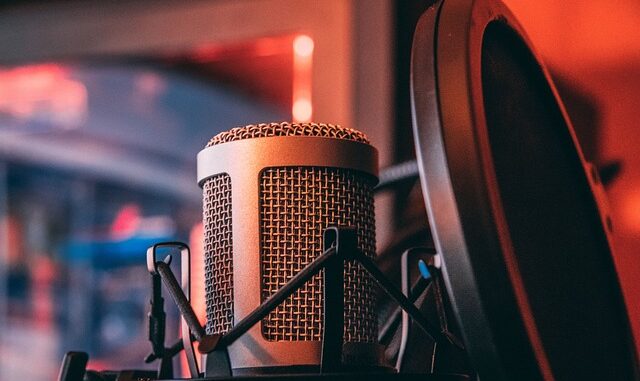
Abstract
Voice assistants (VAs), powered by artificial intelligence (AI), have rapidly evolved from simple command execution devices to sophisticated platforms capable of complex interactions and diverse applications. This research report provides a comprehensive overview of the current state of VAs, encompassing their technical capabilities, expanding applications across various sectors, crucial user interface (UI) and user experience (UX) design considerations, salient data privacy and security implications, pertinent ethical considerations, the competitive landscape driving innovation, advancements in AI poised to revolutionize VA functionalities, integration prospects with other technologies, and an analysis of economic viability alongside strategies for promoting adoption and effective utilization. This report aims to provide a nuanced understanding of VAs for experts, highlighting both their transformative potential and the challenges that must be addressed to ensure responsible and beneficial deployment.
Many thanks to our sponsor Esdebe who helped us prepare this research report.
1. Introduction
Voice assistants (VAs) such as Amazon Alexa, Google Assistant, Apple Siri, and Microsoft Cortana have transitioned from novelty gadgets to integral components of everyday life. Driven by advancements in natural language processing (NLP), machine learning (ML), and cloud computing, VAs now offer a diverse range of functionalities, including information retrieval, task management, home automation, and even healthcare support. The proliferation of smart speakers, smartphones, and other connected devices has further accelerated the adoption of VAs, transforming how individuals interact with technology and the world around them.
However, the increasing pervasiveness of VAs also raises critical questions regarding data privacy, security, ethical implications, and accessibility for diverse user groups. It is imperative to critically examine the current state of VAs, explore their potential benefits and risks, and develop strategies for ensuring their responsible and equitable development and deployment. This report aims to provide an in-depth analysis of these crucial aspects, catering to the specific needs of experts in the field.
Many thanks to our sponsor Esdebe who helped us prepare this research report.
2. Technical Capabilities and Underlying Technologies
The core functionality of VAs relies on a complex interplay of several key technologies:
-
Natural Language Processing (NLP): NLP enables VAs to understand and interpret human language. Key components of NLP include automatic speech recognition (ASR), which converts spoken words into text; natural language understanding (NLU), which analyzes the meaning and intent behind the text; and natural language generation (NLG), which produces coherent and contextually appropriate responses. Recent advances in deep learning, particularly transformer models like BERT, GPT-3, and their successors, have significantly improved the accuracy and fluency of NLP systems, enabling VAs to engage in more natural and nuanced conversations [1, 2].
-
Machine Learning (ML): ML algorithms are used to train VAs to perform various tasks, such as recognizing user commands, predicting user preferences, and personalizing responses. Supervised learning, reinforcement learning, and unsupervised learning techniques are all employed in VA development. For example, supervised learning is used to train VAs to classify user queries into different categories, while reinforcement learning is used to optimize the VA’s response strategies based on user feedback [3].
-
Cloud Computing: Cloud computing provides the infrastructure and resources necessary to store, process, and analyze the vast amounts of data generated by VAs. VAs rely on cloud-based services for tasks such as speech recognition, natural language processing, and data storage. Cloud platforms also enable VAs to be continuously updated and improved through over-the-air updates [4].
-
Knowledge Representation and Reasoning: VAs need to access and process information from various sources to answer user queries and perform tasks. Knowledge representation techniques, such as knowledge graphs and ontologies, are used to structure and organize information in a way that VAs can easily understand and reason with. Reasoning algorithms allow VAs to draw inferences and make decisions based on the available knowledge [5].
The ongoing research and development in these areas are continually expanding the capabilities of VAs. For instance, advancements in zero-shot learning and few-shot learning are enabling VAs to perform new tasks with minimal training data, making them more adaptable to new domains and user needs. Similarly, research in explainable AI (XAI) is aimed at making VAs more transparent and understandable, which is crucial for building trust and accountability.
Many thanks to our sponsor Esdebe who helped us prepare this research report.
3. Applications Across Diverse Sectors
The applications of VAs extend far beyond simple voice commands and entertainment. They are increasingly being integrated into various sectors, transforming workflows and enhancing user experiences:
-
Healthcare: In healthcare, VAs are being used for remote patient monitoring, medication reminders, appointment scheduling, and providing access to health information. They can also assist individuals with disabilities and older adults in managing their daily lives and maintaining their independence [6]. Furthermore, VAs can assist medical professionals with tasks such as transcribing patient notes and accessing clinical guidelines.
-
Education: VAs can provide personalized learning experiences for students by answering questions, providing feedback, and adapting to their individual learning styles. They can also assist teachers with administrative tasks, such as grading assignments and tracking student progress. Educational institutions are exploring the use of VAs to create more engaging and interactive learning environments [7].
-
Retail: VAs are transforming the retail landscape by enabling voice-based shopping, providing product recommendations, and offering customer support. They can also be used to personalize the shopping experience by tracking user preferences and providing targeted promotions. Retailers are leveraging VAs to enhance customer engagement and drive sales [8].
-
Manufacturing: In manufacturing, VAs are being used to control machinery, monitor production processes, and provide real-time information to workers. They can also assist with quality control and maintenance tasks, improving efficiency and reducing downtime. The integration of VAs into industrial settings is contributing to the development of smart factories and the Industrial Internet of Things (IIoT) [9].
-
Finance: VAs are being used to provide financial advice, manage investments, and perform banking transactions. They can also assist with fraud detection and risk management. Financial institutions are exploring the use of VAs to enhance customer service and improve operational efficiency [10].
The proliferation of VAs across these sectors highlights their versatility and adaptability. However, it is crucial to carefully consider the specific needs and requirements of each application area to ensure that VAs are effectively integrated and deliver tangible benefits.
Many thanks to our sponsor Esdebe who helped us prepare this research report.
4. User Interface (UI) and User Experience (UX) Design Considerations
Designing effective UI and UX for VAs requires a different approach compared to traditional graphical user interfaces (GUIs). Key considerations include:
-
Natural Language Interaction: The primary mode of interaction with VAs is through natural language, which requires careful attention to the design of conversational interfaces. The VA should be able to understand a wide range of user expressions, handle ambiguous queries, and provide clear and concise responses. The use of context management techniques is crucial for maintaining coherence and relevance throughout the conversation [11].
-
Personalization and Adaptation: VAs should be able to adapt to the individual user’s preferences, needs, and abilities. This includes personalizing the VA’s voice, language style, and response strategies. The VA should also be able to learn from user interactions and improve its performance over time. Personalization can significantly enhance user satisfaction and engagement [12].
-
Accessibility: VAs should be accessible to users with disabilities, including visual impairments, hearing impairments, and cognitive impairments. This requires designing interfaces that are compatible with assistive technologies and providing alternative input methods, such as text-based commands. Adherence to accessibility guidelines, such as the Web Content Accessibility Guidelines (WCAG), is essential for ensuring inclusivity [13].
-
Context Awareness: VAs should be aware of the user’s context, including their location, time of day, and current activity. This allows the VA to provide more relevant and timely information and suggestions. Context awareness can also be used to proactively anticipate user needs and provide assistance without being explicitly asked [14].
-
Error Handling and Recovery: VAs should be able to gracefully handle errors and recover from unexpected situations. This includes providing clear error messages, offering alternative solutions, and allowing users to easily correct their mistakes. Effective error handling is crucial for maintaining user trust and preventing frustration [15].
Designing effective UI and UX for VAs requires a deep understanding of human-computer interaction principles and a user-centered design approach. It is essential to conduct thorough user research and testing to identify potential usability issues and ensure that the VA meets the needs of its target audience.
Many thanks to our sponsor Esdebe who helped us prepare this research report.
5. Data Privacy and Security Implications
The increasing reliance on VAs raises significant concerns about data privacy and security. VAs collect vast amounts of user data, including voice recordings, location information, and personal preferences. This data can be vulnerable to unauthorized access, misuse, and surveillance. Key concerns include:
-
Data Collection and Storage: VAs typically store user data in the cloud, which raises concerns about data breaches and unauthorized access. It is crucial to implement robust security measures to protect user data from cyberattacks and ensure compliance with data privacy regulations, such as the General Data Protection Regulation (GDPR) and the California Consumer Privacy Act (CCPA) [16, 17].
-
Data Processing and Analysis: VAs use user data to personalize their responses, provide targeted advertising, and improve their performance. However, the use of data for these purposes can raise concerns about bias, discrimination, and manipulation. It is essential to ensure that data processing and analysis are conducted in a fair and transparent manner and that users have control over how their data is used [18].
-
Voice Recording and Storage: VAs typically record and store user voice commands, which raises concerns about privacy and surveillance. It is crucial to provide users with clear information about how their voice recordings are used and to allow them to easily delete their recordings. The use of end-to-end encryption can also help to protect the privacy of voice recordings [19].
-
Third-Party Access: VAs often integrate with third-party services, which can raise concerns about data sharing and privacy. It is essential to carefully vet third-party services and ensure that they comply with data privacy regulations. Users should also be informed about which third-party services their VA is connected to and how their data is being shared [20].
Addressing these data privacy and security concerns requires a multi-faceted approach, including implementing robust security measures, providing users with transparency and control over their data, and developing ethical guidelines for the use of VAs. It is crucial to balance the benefits of VAs with the need to protect user privacy and security.
Many thanks to our sponsor Esdebe who helped us prepare this research report.
6. Ethical Considerations
The deployment of VAs raises several ethical considerations that need careful attention:
-
Bias and Discrimination: VAs can perpetuate and amplify existing biases in data, leading to discriminatory outcomes. For example, a VA trained on biased data might provide different responses to users based on their gender, race, or accent. It is crucial to address bias in VA development by using diverse and representative datasets and by implementing fairness-aware algorithms [21].
-
Privacy and Surveillance: As discussed in the previous section, VAs can collect vast amounts of user data, raising concerns about privacy and surveillance. It is essential to balance the benefits of VAs with the need to protect user privacy and autonomy. This requires developing ethical guidelines for data collection, storage, and use, and ensuring that users have control over their data [22].
-
Transparency and Explainability: VAs are often opaque and difficult to understand, making it challenging to hold them accountable for their actions. It is crucial to make VAs more transparent and explainable by providing users with insights into how they work and why they make certain decisions. This requires developing XAI techniques that can be applied to VAs [23].
-
Job Displacement: The automation capabilities of VAs can lead to job displacement in certain industries. It is essential to mitigate the negative impacts of automation by providing workers with retraining and upskilling opportunities and by exploring alternative economic models that can support a changing workforce [24].
-
Dependence and Social Isolation: Over-reliance on VAs can lead to dependence and social isolation, particularly among vulnerable populations, such as older adults and people with disabilities. It is crucial to promote responsible use of VAs and to encourage users to maintain social connections and engage in meaningful activities [25].
Addressing these ethical considerations requires a collaborative effort involving researchers, developers, policymakers, and the public. It is essential to develop ethical frameworks and guidelines that can guide the responsible development and deployment of VAs.
Many thanks to our sponsor Esdebe who helped us prepare this research report.
7. Competitive Landscape and Future Trends
The VA market is highly competitive, with several major players vying for dominance:
-
Amazon Alexa: Amazon Alexa is one of the leading VAs, with a large installed base and a wide range of skills and integrations. Amazon’s focus on e-commerce and smart home integration has contributed to Alexa’s success [26].
-
Google Assistant: Google Assistant is another leading VA, leveraging Google’s expertise in search and AI. Google Assistant is deeply integrated with Google’s ecosystem of services and is available on a wide range of devices [27].
-
Apple Siri: Apple Siri is the VA integrated into Apple’s devices, including iPhones, iPads, and Macs. Siri benefits from Apple’s loyal customer base and its focus on privacy and security [28].
-
Microsoft Cortana: Microsoft Cortana is the VA integrated into Windows operating systems. Cortana is focused on productivity and enterprise applications [29].
The competitive landscape is driving innovation in VA technology. Key trends include:
-
Improved NLP and Understanding: Ongoing advancements in NLP are enabling VAs to understand and respond to user queries more accurately and fluently.
-
Personalization and Context Awareness: VAs are becoming more personalized and context-aware, adapting to the individual user’s preferences and needs.
-
Integration with Other Technologies: VAs are being integrated with other technologies, such as virtual reality (VR) and augmented reality (AR), to create more immersive and interactive experiences.
-
Edge Computing: VAs are increasingly being deployed on edge devices, such as smartphones and smart speakers, reducing latency and improving privacy.
-
Specialized VAs: There is a growing trend towards specialized VAs that are designed for specific tasks or industries, such as healthcare or finance.
The future of VAs is likely to be characterized by increased intelligence, personalization, and integration with other technologies. VAs will become more seamlessly integrated into our lives, providing assistance and support in a wide range of contexts.
Many thanks to our sponsor Esdebe who helped us prepare this research report.
8. Economic Viability and Adoption Strategies
The economic viability of VAs depends on several factors, including the cost of development, deployment, and maintenance, as well as the potential benefits they provide in terms of increased efficiency, productivity, and customer satisfaction. Key considerations include:
-
Cost-Benefit Analysis: It is essential to conduct a thorough cost-benefit analysis before investing in VA technology. This includes assessing the potential costs and benefits of different VA solutions and comparing them to alternative approaches.
-
Return on Investment (ROI): The ROI of VAs can vary depending on the specific application and the effectiveness of the implementation. It is important to track key performance indicators (KPIs) to measure the ROI and identify areas for improvement.
-
Business Models: Several business models are being used to monetize VA technology, including subscription fees, usage-based pricing, and advertising. The choice of business model depends on the specific application and the target market.
-
Scalability: The scalability of VA solutions is crucial for ensuring their long-term economic viability. It is important to design VA solutions that can be easily scaled to meet growing demand.
Strategies for promoting the adoption and effective use of VAs include:
-
Education and Training: Providing users with education and training on how to use VAs effectively can increase adoption and improve user satisfaction.
-
Demonstration Projects: Conducting demonstration projects to showcase the benefits of VAs in real-world settings can help to overcome skepticism and encourage adoption.
-
Government Support: Government support, such as funding for research and development, can help to accelerate the adoption of VAs.
-
Industry Standards: Developing industry standards for VA technology can promote interoperability and reduce the risk of vendor lock-in.
-
Addressing Ethical Concerns: Addressing ethical concerns related to data privacy, bias, and job displacement can build trust and encourage responsible adoption of VAs.
The successful adoption of VAs requires a combination of technological innovation, economic viability, and ethical considerations. By addressing these factors, we can unlock the full potential of VAs to improve our lives and transform our society.
Many thanks to our sponsor Esdebe who helped us prepare this research report.
9. Conclusion
Voice assistants have evolved significantly and are poised to play an increasingly important role in various aspects of our lives. Their capabilities, driven by advancements in AI and related technologies, are expanding rapidly, opening up new possibilities across healthcare, education, retail, manufacturing, and finance. However, the widespread adoption of VAs also presents significant challenges related to data privacy, security, ethical considerations, and accessibility. Addressing these challenges requires a concerted effort from researchers, developers, policymakers, and the public. By carefully considering the technical, ethical, and economic aspects of VAs, we can ensure that they are developed and deployed in a responsible and beneficial manner, maximizing their potential to improve our lives and transform our society. Further research is needed to address the identified gaps in knowledge and to develop innovative solutions that can mitigate the risks associated with VAs while harnessing their immense potential.
Many thanks to our sponsor Esdebe who helped us prepare this research report.
References
[1] Vaswani, A., Shazeer, N., Parmar, N., Uszkoreit, J., Jones, L., Gomez, A. N., … & Polosukhin, I. (2017). Attention is all you need. Advances in neural information processing systems, 30.
[2] Devlin, J., Chang, M. W., Lee, K., & Toutanova, K. (2018). Bert: Pre-training of deep bidirectional transformers for language understanding. arXiv preprint arXiv:1810.04805.
[3] Sutton, R. S., & Barto, A. G. (2018). Reinforcement learning: An introduction. MIT press.
[4] Armbrust, M., Fox, A., Griffith, R., Joseph, A. D., Katz, R., Konwinski, A., … & Zaharia, M. (2010). A view of cloud computing. Communications of the ACM, 53(4), 50-58.
[5] Ehrig, M. (2006). Ontological engineering: With examples from the areas of knowledge management, e-commerce and semantic web. Springer Science & Business Media.
[6] Hoyle, M. J., & McGregor, A. H. (2016). Voice assistants: are they a worthwhile addition to healthcare?. BMJ innovations, 2(4), 204-208.
[7] Holmes, W., Bialik, M., & Fadel, C. (2019). Artificial intelligence in education: Promises and implications for teaching and learning. Center for Curriculum Redesign.
[8] Davenport, T. H., & Mittal, S. (2016). Knowing what customers want before they do. Harvard Business Review, 94(5), 82-89.
[9] Lee, J., Bagheri, B., & Kao, H. A. (2015). A cyber-physical systems architecture for industry 4.0-based manufacturing systems. Manufacturing letters, 3(1), 15-18.
[10] King, R., & Brown, S. (2018). Artificial intelligence and fintech: an overview. Deloitte Centre for Financial Services.
[11] Clark, B. H. (2018). Conversational AI: Dialogue systems, machine translation, and natural language generation. O’Reilly Media.
[12] Aggarwal, C. C. (2016). Recommender systems: The textbook. Springer.
[13] Kirkpatrick, A., O’Connor, L., & Feathers, M. (2008). Meeting WCAG 2.0: A guide to understanding and implementing Web content accessibility guidelines. W3C/MIT.
[14] Dey, A. K. (2001). Understanding and using context. Personal and ubiquitous computing, 5(1), 4-7.
[15] Nielsen, J. (1994). Usability engineering. Academic Press.
[16] European Parliament. (2016). Regulation (EU) 2016/679 of the European Parliament and of the Council of 27 April 2016 on the protection of natural persons with regard to the processing of personal data and on the free movement of such data, and repealing Directive 95/46/EC (General Data Protection Regulation).
[17] California Legislative Information. (2018). Assembly Bill No. 375: California Consumer Privacy Act of 2018.
[18] O’Neil, C. (2016). Weapons of math destruction: How big data increases inequality and threatens democracy. Crown.
[19] Schneier, B. (2007). Applied cryptography: protocols, algorithms, and source code in C. John Wiley & Sons.
[20] Zittrain, J. (2008). The future of the internet–and how to stop it. Yale University Press.
[21] Friedler, S. A., Scheidegger, C., & Venkatasubramanian, S. (2016). On the (im) possibility of fairness. arXiv preprint arXiv:1609.07236.
[22] Nissenbaum, H. (2004). Privacy as contextual integrity. Washington Law Review, 79(1), 119-158.
[23] Doshi-Velez, F., & Kim, B. (2017). Towards a rigorous science of interpretable machine learning. arXiv preprint arXiv:1702.08608.
[24] Brynjolfsson, E., & McAfee, A. (2014). The second machine age: Work, progress, and prosperity in a time of brilliant technologies. WW Norton & Company.
[25] Turkle, S. (2011). Alone together: Why we expect more from technology and less from each other. Simon and Schuster.
[26] Amazon. (n.d.). Amazon Alexa. Retrieved from https://www.amazon.com/alexa-devices/b?ie=UTF8&node=21334920011
[27] Google. (n.d.). Google Assistant. Retrieved from https://assistant.google.com/
[28] Apple. (n.d.). Siri. Retrieved from https://www.apple.com/siri/
[29] Microsoft. (n.d.). Microsoft Cortana. Retrieved from https://www.microsoft.com/en-us/cortana
The report highlights ethical considerations such as bias in VA responses. Could advancements in federated learning, where models are trained across decentralized devices, offer a potential pathway to mitigate data bias and enhance user privacy simultaneously?
That’s a great point! Federated learning definitely holds promise for reducing bias by training on more diverse datasets while preserving privacy. It will be interesting to see how further research and development affects the widespread adoption of this method for voice assistants.
Editor: MedTechNews.Uk
Thank you to our Sponsor Esdebe