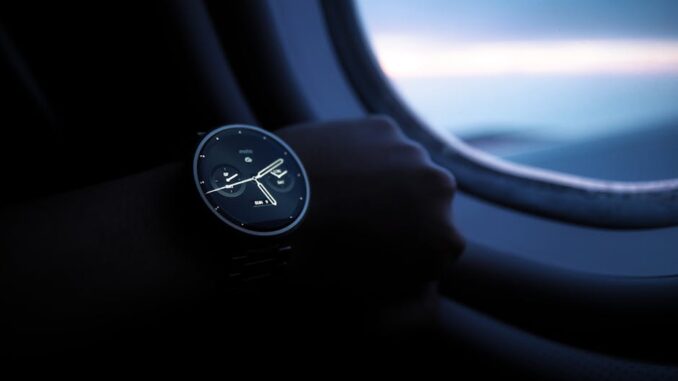
Abstract
Wearable sensor technology has undergone a rapid evolution, transforming healthcare diagnostics, monitoring, and preventative care. While photoplethysmography (PPG) sensors have garnered significant attention as a cornerstone for AI-driven detection systems due to their ability to provide insights into cardiovascular activity, a comprehensive understanding of the broader wearable sensor ecosystem is crucial. This research report delves into the multifaceted aspects of wearable sensors, exploring various types beyond PPG, including accelerometers, gyroscopes, electrocardiogram (ECG) monitors, and emerging modalities. We critically assess their accuracy, reliability, and limitations, while addressing crucial concerns related to data security, privacy, battery life, and user compliance. Furthermore, the report examines the complex regulatory landscape governing medical-grade wearables, the potential for seamless integration with other health monitoring devices and systems, and emerging trends driving innovation in miniaturization, enhanced accuracy, novel sensor functionalities, and AI-driven analytics. This holistic approach aims to provide experts with a nuanced understanding of the current state and future trajectory of wearable sensor technology in healthcare.
Many thanks to our sponsor Esdebe who helped us prepare this research report.
1. Introduction
The proliferation of wearable sensors has ushered in a new era of personalized healthcare, characterized by continuous physiological monitoring, early disease detection, and proactive intervention strategies. These devices, seamlessly integrated into everyday life through wristbands, patches, clothing, and even implantable systems, offer a wealth of real-time data that can be leveraged by artificial intelligence (AI) to improve patient outcomes. While PPG sensors, which utilize optical techniques to measure blood volume changes and derive heart rate, heart rate variability (HRV), and other cardiovascular parameters, have emerged as prominent components of AI-driven diagnostic systems [1], relying solely on PPG overlooks the vast potential of other sensor modalities and their synergistic contributions. This report aims to broaden the scope of investigation by critically analyzing the diverse array of wearable sensors, assessing their respective strengths and weaknesses, and exploring their integration possibilities for enhanced AI-driven healthcare applications.
Wearable sensor technology is not merely about data collection; it encompasses a complex interplay of hardware, software, algorithms, and user experience. The successful implementation of these devices hinges on addressing critical challenges such as data security, privacy, battery life, user compliance, and regulatory compliance. Furthermore, the integration of wearable sensor data with electronic health records (EHRs) and other healthcare IT systems requires robust interoperability standards and seamless data exchange protocols. These challenges necessitate a multidisciplinary approach involving engineers, clinicians, data scientists, ethicists, and policymakers to ensure the responsible and effective deployment of wearable sensor technology in healthcare.
Many thanks to our sponsor Esdebe who helped us prepare this research report.
2. Types of Wearable Sensors in Healthcare
A diverse range of wearable sensors is employed in healthcare, each with its unique capabilities and limitations. This section provides an overview of the major sensor categories and their applications.
2.1 Photoplethysmography (PPG) Sensors
PPG sensors are widely used for measuring heart rate and heart rate variability (HRV) by detecting changes in blood volume in peripheral tissues. The basic principle involves emitting light (typically green or infrared) into the skin and measuring the amount of light reflected or transmitted. The pulsatile component of the signal reflects changes in blood volume due to the cardiac cycle. Beyond heart rate and HRV, PPG signals can also be used to estimate blood pressure [2], oxygen saturation (SpO2), and respiratory rate [3].
Advantages: Non-invasive, relatively inexpensive, and can be easily integrated into wearable devices.
Limitations: Susceptible to motion artifacts, variations in skin pigmentation, and ambient light interference. Signal quality can be affected by poor sensor contact and vasoconstriction.
2.2 Accelerometers
Accelerometers measure acceleration forces, providing information about movement patterns, physical activity levels, and posture. These sensors are based on micro-electromechanical systems (MEMS) technology and can detect acceleration along one, two, or three axes. In healthcare, accelerometers are used for monitoring activity levels in patients with chronic diseases, assessing gait and balance in elderly individuals [4], and tracking sleep patterns [5].
Advantages: Small, low power consumption, and relatively robust to environmental conditions.
Limitations: Limited ability to distinguish between different types of activities. Susceptible to drift and bias errors.
2.3 Gyroscopes
Gyroscopes measure angular velocity, providing information about rotational movements. When combined with accelerometers, gyroscopes can provide a more comprehensive understanding of movement dynamics. In healthcare, gyroscopes are used for assessing motor control in patients with neurological disorders, monitoring tremor in Parkinson’s disease [6], and tracking head movements in virtual reality-based rehabilitation therapies.
Advantages: Highly sensitive to rotational movements, relatively immune to linear accelerations.
Limitations: Susceptible to drift errors, can be affected by temperature changes.
2.4 Electrocardiogram (ECG) Monitors
ECG monitors measure the electrical activity of the heart, providing detailed information about heart rhythm, heart rate, and potential cardiac abnormalities. Wearable ECG monitors can be used for continuous monitoring of patients at risk of arrhythmias, detecting myocardial ischemia, and assessing the effectiveness of cardiac medications [7].
Advantages: High accuracy in detecting cardiac events, can provide detailed diagnostic information.
Limitations: Requires skin contact with electrodes, can be uncomfortable for long-term wear, and may be susceptible to noise from muscle activity.
2.5 Electromyography (EMG) Sensors
EMG sensors measure the electrical activity produced by skeletal muscles. Wearable EMG sensors can be used to monitor muscle fatigue, assess movement disorders, and control prosthetic devices. These sensors are becoming increasingly important in rehabilitation and sports medicine.
Advantages: Can provide real-time information about muscle activity.
Limitations: Sensitive to noise and requires careful electrode placement. Requires conductive gel for optimal signal acquisition.
2.6 Other Emerging Sensor Modalities
Beyond the established sensor types, several emerging modalities are showing promise for healthcare applications:
- Chemical Sensors: These sensors can measure various biomarkers in sweat, saliva, or interstitial fluid, providing information about glucose levels, electrolytes, and other physiological parameters. Microfluidic systems and advanced nanomaterials are driving innovation in this area.
- Pressure Sensors: These sensors can measure pressure exerted on the skin or internal organs, providing information about wound healing, bladder pressure, and other physiological conditions. Flexible and stretchable pressure sensors are being developed for comfortable and conformal integration with the body.
- Temperature Sensors: Core body temperature is a vital sign, and continuous monitoring is important in various clinical contexts. Wearable temperature sensors are being developed for infection detection and sleep monitoring.
Many thanks to our sponsor Esdebe who helped us prepare this research report.
3. Accuracy, Reliability, and Limitations
The accuracy and reliability of wearable sensors are critical for their effective use in healthcare. Several factors can affect sensor performance, including sensor design, signal processing algorithms, environmental conditions, and user behavior. In this section, we critically assess the accuracy and reliability of different sensor types and discuss their limitations.
3.1 Accuracy and Reliability Metrics
The accuracy of wearable sensors is typically assessed by comparing their measurements to those of gold-standard clinical devices. Common accuracy metrics include mean absolute error (MAE), root mean squared error (RMSE), and correlation coefficient. The reliability of wearable sensors refers to their ability to consistently produce similar measurements under the same conditions. Reliability is typically assessed using test-retest reliability, inter-rater reliability, and Cronbach’s alpha.
3.2 Factors Affecting Accuracy and Reliability
Several factors can affect the accuracy and reliability of wearable sensors:
- Motion Artifacts: Movement can introduce noise into sensor signals, particularly in PPG and accelerometer measurements. Advanced signal processing algorithms, such as adaptive filtering and wavelet transforms, can be used to mitigate motion artifacts.
- Skin Contact: Poor skin contact can degrade signal quality, particularly in ECG and EMG measurements. Proper sensor placement and the use of conductive gels can improve skin contact.
- Environmental Conditions: Temperature, humidity, and ambient light can affect sensor performance. Sensors should be designed to be robust to environmental variations.
- User Behavior: User compliance with wearing instructions and adherence to proper sensor placement are crucial for accurate measurements. User education and training can improve compliance.
- Algorithm Design: The algorithms used to process sensor data can significantly impact the accuracy of the final measurements. AI-powered algorithms trained on large datasets are becoming increasingly sophisticated at extracting meaningful information from noisy sensor signals [8].
3.3 Addressing Limitations
Researchers are actively working to address the limitations of wearable sensors through various strategies:
- Sensor Fusion: Combining data from multiple sensors can improve accuracy and robustness. For example, combining accelerometer and gyroscope data can provide a more accurate assessment of physical activity.
- Advanced Signal Processing: Developing advanced signal processing algorithms to mitigate noise and artifacts is crucial for improving sensor performance.
- Personalized Calibration: Calibrating sensors to individual users can improve accuracy by accounting for individual differences in physiology and anatomy.
- AI-Driven Error Correction: Using AI to detect and correct errors in sensor data can significantly improve the reliability of measurements.
Many thanks to our sponsor Esdebe who helped us prepare this research report.
4. Data Security and Privacy Concerns
The collection and storage of sensitive health data by wearable sensors raise significant data security and privacy concerns. This section discusses the key challenges and potential solutions.
4.1 Data Security Threats
Wearable sensor data is vulnerable to various security threats, including:
- Unauthorized Access: Hackers can gain access to sensor data through vulnerabilities in the device, the communication protocols, or the cloud storage system.
- Data Interception: Data can be intercepted during transmission between the sensor and the smartphone or the cloud server.
- Malware Infections: Wearable devices can be infected with malware, which can compromise data security and privacy.
- Phishing Attacks: Users can be tricked into providing their login credentials to fake websites or emails.
4.2 Privacy Risks
The collection and use of wearable sensor data can pose several privacy risks:
- Re-identification: Anonymized data can be re-identified through the use of sophisticated data mining techniques.
- Data Sharing: Data can be shared with third parties without the user’s explicit consent.
- Discriminatory Practices: Data can be used to discriminate against individuals in areas such as employment, insurance, and housing.
- Surveillance: Data can be used for surveillance purposes by governments or corporations.
4.3 Mitigation Strategies
Several strategies can be used to mitigate data security and privacy risks:
- Encryption: Encrypting data both at rest and in transit can protect it from unauthorized access.
- Access Controls: Implementing strong access controls can limit access to sensitive data to authorized personnel.
- Data Anonymization: Anonymizing data can reduce the risk of re-identification.
- Privacy-Enhancing Technologies: Using privacy-enhancing technologies, such as differential privacy, can protect user privacy while still allowing for data analysis.
- Transparency and Consent: Being transparent about data collection and usage practices and obtaining explicit consent from users can build trust and ensure compliance with privacy regulations.
- Regular Security Audits: Conducting regular security audits can identify and address vulnerabilities in the system.
Many thanks to our sponsor Esdebe who helped us prepare this research report.
5. Battery Life and User Compliance Challenges
Battery life and user compliance are critical factors for the long-term success of wearable sensors in healthcare. This section discusses the challenges and potential solutions.
5.1 Battery Life Limitations
The limited battery life of wearable sensors can be a major obstacle to continuous monitoring. Frequent charging can be inconvenient for users and can lead to non-compliance. Battery life is affected by several factors, including sensor type, sampling rate, data processing algorithms, and communication protocols.
5.2 User Compliance Issues
User compliance refers to the extent to which users adhere to the recommended usage guidelines for wearable sensors. Non-compliance can lead to inaccurate data and reduced effectiveness of the monitoring system. Factors affecting user compliance include comfort, ease of use, aesthetic appeal, and perceived value.
5.3 Strategies for Improving Battery Life and User Compliance
Several strategies can be used to improve battery life and user compliance:
- Low-Power Sensor Design: Designing sensors with low power consumption can extend battery life.
- Adaptive Sampling: Using adaptive sampling techniques, which adjust the sampling rate based on activity levels, can reduce power consumption.
- Energy Harvesting: Exploring energy harvesting techniques, such as solar energy or kinetic energy, can supplement battery power.
- Wireless Charging: Implementing wireless charging can make it more convenient for users to recharge their devices.
- Ergonomic Design: Designing comfortable and aesthetically appealing devices can improve user compliance.
- User-Friendly Interface: Developing a user-friendly interface can make it easier for users to interact with the device and understand the data.
- Gamification: Using gamification techniques can motivate users to wear and use the device regularly.
- Personalized Feedback: Providing personalized feedback based on the sensor data can increase the perceived value of the device and improve user compliance.
Many thanks to our sponsor Esdebe who helped us prepare this research report.
6. Regulatory Landscape for Medical-Grade Wearables
The regulatory landscape for medical-grade wearables is complex and evolving. In the United States, the Food and Drug Administration (FDA) regulates medical devices to ensure their safety and effectiveness. This section provides an overview of the regulatory framework and its implications for wearable sensor technology.
6.1 FDA Regulation of Medical Devices
The FDA classifies medical devices into three classes based on their risk level: Class I, Class II, and Class III. Class I devices are considered low-risk and are subject to general controls, such as good manufacturing practices. Class II devices are considered moderate-risk and are subject to special controls, such as performance standards and premarket notification. Class III devices are considered high-risk and are subject to premarket approval, which requires extensive clinical testing to demonstrate safety and effectiveness.
6.2 Regulatory Considerations for Wearable Sensors
The regulatory requirements for wearable sensors depend on their intended use and risk level. Wearable sensors that are intended to diagnose, treat, or prevent disease are considered medical devices and are subject to FDA regulation. Wearable sensors that are intended for general wellness purposes, such as tracking activity levels or sleep patterns, are generally not regulated by the FDA.
6.3 Challenges in Regulatory Compliance
Several challenges arise in the regulatory compliance of wearable sensors:
- Defining Intended Use: Determining the intended use of a wearable sensor can be challenging, particularly for devices with multiple functions.
- Demonstrating Safety and Effectiveness: Demonstrating the safety and effectiveness of wearable sensors can require extensive clinical testing, which can be costly and time-consuming.
- Evolving Regulatory Landscape: The regulatory landscape for wearable sensors is constantly evolving, making it difficult for manufacturers to stay up-to-date with the latest requirements.
6.4 International Regulatory Frameworks
Beyond the FDA, other countries have their own regulatory frameworks for medical devices. For example, the European Union has the Medical Device Regulation (MDR), which sets strict requirements for the safety and performance of medical devices. Compliance with international regulations is essential for manufacturers seeking to market their products globally.
Many thanks to our sponsor Esdebe who helped us prepare this research report.
7. Integration with Other Health Monitoring Devices and Systems
The integration of wearable sensor data with other health monitoring devices and systems is crucial for creating a comprehensive and integrated healthcare ecosystem. This section explores the potential for integration with electronic health records (EHRs), remote patient monitoring (RPM) systems, and telehealth platforms.
7.1 Integration with Electronic Health Records (EHRs)
Integrating wearable sensor data with EHRs can provide clinicians with a more complete picture of the patient’s health status. This integration can enable more informed decision-making, personalized treatment plans, and improved patient outcomes. However, several challenges exist in integrating wearable sensor data with EHRs, including data standardization, interoperability, and data security.
7.2 Integration with Remote Patient Monitoring (RPM) Systems
Integrating wearable sensors with RPM systems can enable continuous monitoring of patients in their homes, reducing the need for frequent hospital visits. This integration can improve patient access to care, reduce healthcare costs, and improve patient outcomes. RPM systems typically include wearable sensors, communication devices, and data analytics platforms.
7.3 Integration with Telehealth Platforms
Integrating wearable sensors with telehealth platforms can enable remote consultations, virtual check-ups, and remote rehabilitation. This integration can improve patient access to care, reduce travel costs, and improve patient convenience. Telehealth platforms typically include video conferencing, secure messaging, and remote monitoring capabilities.
7.4 Interoperability Standards
Interoperability standards, such as HL7 and FHIR, are essential for enabling seamless data exchange between different healthcare systems. These standards define the format and content of data messages, allowing different systems to communicate with each other. Adopting interoperability standards is crucial for facilitating the integration of wearable sensor data with other healthcare systems.
Many thanks to our sponsor Esdebe who helped us prepare this research report.
8. Future Trends in Wearable Sensor Technology
Wearable sensor technology is rapidly evolving, driven by advancements in materials science, microelectronics, and artificial intelligence. This section explores some of the key future trends in this field.
8.1 Miniaturization
The trend towards miniaturization will continue, leading to smaller and more discreet wearable sensors. This will improve user comfort and compliance, making it easier for people to wear sensors for extended periods of time. Nanotechnology and microfabrication techniques are enabling the development of ultra-small sensors that can be integrated into clothing or even implanted into the body.
8.2 Improved Accuracy and Reliability
Ongoing research is focused on improving the accuracy and reliability of wearable sensors. This includes developing new sensor materials, advanced signal processing algorithms, and personalized calibration techniques. AI-powered algorithms are playing an increasingly important role in improving sensor performance by mitigating noise and artifacts and extracting meaningful information from complex sensor signals.
8.3 New Sensor Modalities
New sensor modalities are being developed to measure a wider range of physiological parameters. This includes chemical sensors for measuring biomarkers in sweat, saliva, and interstitial fluid, as well as pressure sensors for monitoring wound healing and bladder pressure. These new sensor modalities will enable more comprehensive and personalized health monitoring.
8.4 AI-Driven Analytics
Artificial intelligence (AI) is playing an increasingly important role in wearable sensor technology. AI algorithms can be used to analyze sensor data, detect patterns, and predict health outcomes. AI-driven analytics can enable early disease detection, personalized treatment plans, and proactive intervention strategies. Furthermore, AI can be used to improve sensor performance, mitigate noise and artifacts, and personalize sensor calibration.
8.5 Ubiquitous Sensing
In the future, wearable sensors will become more ubiquitous, seamlessly integrated into our daily lives. This includes sensors embedded in clothing, accessories, and even implanted into the body. Ubiquitous sensing will enable continuous and pervasive health monitoring, providing valuable insights into our health and well-being.
Many thanks to our sponsor Esdebe who helped us prepare this research report.
9. Conclusion
Wearable sensor technology holds immense promise for transforming healthcare, offering unprecedented opportunities for personalized monitoring, early disease detection, and proactive intervention. While PPG sensors have established their significance as a fundamental component of AI-driven detection systems, a more holistic perspective encompassing the diverse array of wearable sensor modalities is critical. The integration of accelerometers, gyroscopes, ECG monitors, and emerging sensor technologies presents synergistic advantages, enhancing the accuracy, reliability, and comprehensiveness of health monitoring.
However, realizing the full potential of wearable sensors requires addressing several challenges, including data security and privacy concerns, battery life limitations, user compliance issues, and regulatory complexities. Mitigation strategies such as robust encryption, stringent access controls, privacy-enhancing technologies, and user-centered design are essential to foster trust and ensure responsible deployment. Furthermore, interoperability standards and seamless integration with existing healthcare systems are crucial for maximizing the clinical utility of wearable sensor data.
Looking ahead, the future of wearable sensor technology is characterized by miniaturization, enhanced accuracy, novel sensor modalities, and the increasing prevalence of AI-driven analytics. These advancements will enable more personalized, proactive, and ultimately, more effective healthcare delivery. By embracing a multidisciplinary approach that integrates engineering, clinical expertise, data science, ethical considerations, and policy frameworks, we can unlock the transformative potential of wearable sensors and usher in a new era of data-driven healthcare.
Many thanks to our sponsor Esdebe who helped us prepare this research report.
References
[1] Allen, J. (2007). Photoplethysmography and its application in clinical physiological measurement. Physiological Measurement, 28(3), R1.
[2] Elgendi, M., Norton, I., Breuss, M., Abbott, D., Schuurmans, C., & Howard, N. (2018). Systolic blood pressure estimation using a wrist-worn device incorporating photoplethysmography and accelerometry. Scientific Reports, 8(1), 1-10.
[3] Charlton, P. H., Bonnici, T., Tarassenko, L., Clifton, D. A., Beale, R., & Watkinson, P. J. (2016). An assessment of algorithms to estimate respiratory rate from the photoplethysmogram. Physiological Measurement, 37(10), 1618.
[4] Weiss, A., Galperin, I., Dorfman, M., & Herman, T. (2013). Accelerometers for gait and motion analysis: a review. Journal of Neuroengineering and Rehabilitation, 10(1), 67.
[5] Marino, M., Li, Y., Rueschman, M. N., Buzzell, G. A., Hayes, A. L., Youngstedt, S. D., … & Buysse, D. J. (2013). Measuring sleep: accuracy, sensitivity, and specificity of wrist actigraphy compared to polysomnography. Sleep, 36(12), 1747-1755.
[6] Elble, R. J., & Koller, W. C. (1990). Tremor. Johns Hopkins University Press.
[7] Goldberger, J. J., Buxton, A. E., Cain, M. E., Estes, N. A. M., Josephson, M. E., Klein, G. J., … & Zipes, D. P. (2008). ACC/AHA/HRS 2008 guidelines for device-based therapy of cardiac rhythm abnormalities: a report of the American College of Cardiology/American Heart Association Task Force on Practice Guidelines (Writing Committee to Revise the ACC/AHA/NASPE 2002 Guideline Update for Implantation of Cardiac Pacemakers and Antiarrhythmia Devices). Circulation, 117(21), e350-e408.
[8] Acharya, U. R., Oh, S. L., Hagiwara, Y., Tan, J. H., Adam, M., Gertych, A., … & Sharma, A. (2017). A deep convolutional neural network framework to classify diabetes retinopathy stages using fundus images. Computers in Biology and Medicine, 84, 276-282.
So, if my Fitbit *also* measured my hydration levels via sweat, would that finally justify my love of salty snacks as a crucial part of my ‘health regime’? Asking for a friend, obviously.