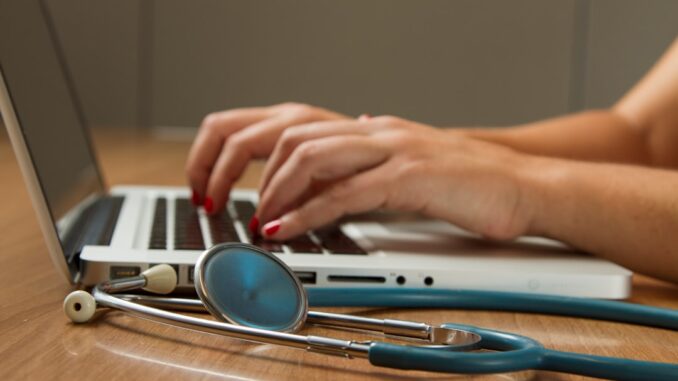
Abstract
Radiology, a cornerstone of modern medicine, is undergoing a profound transformation driven by artificial intelligence (AI). This research report provides a comprehensive overview of the expanding role of radiology in the healthcare ecosystem, emphasizing the impact of AI on its various facets. Beyond the immediate applications of AI-driven image analysis, this report examines the evolving landscape of radiological practice, research, and education. We explore the increasing significance of quantitative imaging, multi-omics integration, and the radiologist’s role as a crucial node in multidisciplinary clinical decision-making. We also address challenges related to the responsible implementation of AI, including data governance, bias mitigation, and the need for robust validation. Furthermore, the report discusses the potential for AI to personalize radiological services, enhance patient engagement, and improve healthcare accessibility. This report is intended for experts in the field, providing a holistic perspective on the current state and future trajectory of radiology in the age of AI.
Many thanks to our sponsor Esdebe who helped us prepare this research report.
1. Introduction
Radiology has evolved from a diagnostic specialty primarily focused on visual interpretation of anatomical images to a highly sophisticated field with a pivotal role in diagnosis, treatment planning, and monitoring disease progression. The advent of digital imaging technologies, such as computed tomography (CT), magnetic resonance imaging (MRI), and positron emission tomography (PET), has enabled the acquisition of increasingly detailed and quantitative data. This has paved the way for the integration of artificial intelligence (AI) into radiological workflows, promising to revolutionize various aspects of the field [1].
While much of the initial focus has been on AI-driven image analysis for tasks such as lesion detection and segmentation, the transformative potential of AI extends far beyond these applications. AI is poised to reshape radiological education, research, and clinical practice, enabling radiologists to provide more personalized, efficient, and accurate care [2]. This report aims to provide a comprehensive overview of the expanding horizon of radiology, examining the impact of AI on its various facets and highlighting the challenges and opportunities that lie ahead.
Many thanks to our sponsor Esdebe who helped us prepare this research report.
2. The Rise of Quantitative Imaging and Radiomics
The shift from qualitative to quantitative imaging represents a fundamental change in radiological practice. Quantitative imaging techniques extract quantifiable metrics from medical images, providing objective and reproducible data that can be used for diagnosis, prognosis, and treatment response assessment [3]. Radiomics, a field that leverages AI and machine learning algorithms to extract a large number of quantitative features from radiological images, has emerged as a powerful tool for biomarker discovery and personalized medicine [4].
Radiomic features can capture subtle changes in tumor size, shape, texture, and heterogeneity that may not be readily apparent to the human eye. These features can be correlated with clinical outcomes, genetic information, and other relevant data to develop predictive models that can guide treatment decisions [5]. For example, radiomic features extracted from CT scans of lung cancer patients have been shown to be predictive of response to chemotherapy and overall survival [6].
The implementation of radiomics workflows presents several challenges, including the need for standardized image acquisition protocols, robust image segmentation algorithms, and rigorous validation studies. However, the potential benefits of radiomics in terms of improved diagnostic accuracy, personalized treatment planning, and reduced healthcare costs are significant [7]. AI plays a crucial role in automating radiomic feature extraction and building predictive models, making it an essential tool for quantitative imaging analysis.
Many thanks to our sponsor Esdebe who helped us prepare this research report.
3. Integration with Multi-Omics Data
In the era of precision medicine, integrating radiological data with other omics data, such as genomics, proteomics, and metabolomics, is becoming increasingly important. This multi-omics approach can provide a more comprehensive understanding of disease biology and enable the development of more targeted and effective therapies [8].
Radiogenomics, a field that combines radiological and genomic data, has shown particular promise. By correlating radiomic features with genetic mutations, researchers can identify imaging biomarkers that are associated with specific genetic profiles. This can help to stratify patients into different risk groups and predict their response to targeted therapies [9]. For example, radiogenomic studies have identified imaging biomarkers that are associated with EGFR mutations in lung cancer patients and BRAF mutations in melanoma patients [10].
The integration of radiological data with other omics data requires sophisticated data integration and analysis techniques. AI algorithms can be used to identify complex relationships between radiological features and omics data, providing insights that would be difficult to obtain using traditional statistical methods. This integrated approach holds great promise for advancing our understanding of disease and developing more personalized treatment strategies [11].
Many thanks to our sponsor Esdebe who helped us prepare this research report.
4. The Evolving Role of the Radiologist
The increasing use of AI in radiology is prompting a re-evaluation of the radiologist’s role in the healthcare ecosystem. While some have expressed concerns that AI may eventually replace radiologists, the more likely scenario is that AI will augment the radiologist’s capabilities, allowing them to focus on more complex and value-added tasks [12].
Radiologists will increasingly need to be skilled in interpreting and validating AI-generated findings. They will also need to be able to integrate AI-derived information with other clinical data to make informed decisions. In addition, radiologists will play a crucial role in developing and implementing AI algorithms, ensuring that they are accurate, reliable, and ethically sound [13].
Furthermore, radiologists will need to become more active participants in multidisciplinary clinical decision-making. As imaging becomes more quantitative and integrated with other omics data, radiologists will be in a unique position to provide valuable insights that can inform treatment decisions. This requires radiologists to have strong communication skills and a deep understanding of clinical medicine [14]. The radiologist of the future will be a data scientist, a clinical consultant, and a technology innovator, playing a central role in the delivery of personalized and precision medicine.
Many thanks to our sponsor Esdebe who helped us prepare this research report.
5. Addressing Challenges and Ethical Considerations
The widespread adoption of AI in radiology presents several challenges that must be addressed to ensure responsible and ethical implementation. These challenges include data privacy, bias mitigation, regulatory hurdles, and integration with existing systems [15].
Data privacy is a major concern, as radiological images contain sensitive patient information. Robust data governance policies and security measures are needed to protect patient privacy and comply with regulations such as HIPAA [16]. Furthermore, AI algorithms can perpetuate and amplify existing biases in data, leading to inaccurate or unfair results. It is crucial to develop strategies for identifying and mitigating bias in AI algorithms, ensuring that they are fair and equitable for all patients [17].
Regulatory hurdles also pose a significant challenge. AI-based diagnostic tools are considered medical devices and are subject to regulatory approval by agencies such as the FDA. The regulatory pathway for AI-based medical devices is still evolving, and clear guidelines are needed to ensure that these devices are safe and effective [18]. In addition, integrating AI algorithms with existing radiology information systems (RIS) and picture archiving and communication systems (PACS) can be complex and costly. Standardized interfaces and data formats are needed to facilitate seamless integration [19].
Furthermore, the “black box” nature of some AI algorithms raises concerns about transparency and explainability. It is important to develop AI algorithms that are transparent and interpretable, allowing radiologists to understand how they arrive at their conclusions. This is particularly important for high-stakes decisions, such as cancer diagnosis and treatment planning [20]. Ethical considerations surrounding the use of AI in radiology must be carefully addressed to ensure that these technologies are used responsibly and for the benefit of patients.
Many thanks to our sponsor Esdebe who helped us prepare this research report.
6. The Impact on Radiological Education and Training
The integration of AI into radiology requires a significant shift in radiological education and training. Radiologists of the future will need to be proficient in AI concepts, machine learning algorithms, and data science techniques. They will also need to be able to critically evaluate AI-generated findings and integrate them with other clinical data [21].
Radiology residency programs need to incorporate AI training into their curricula, providing residents with hands-on experience in using AI tools and developing AI algorithms. This training should include topics such as image processing, machine learning, data visualization, and ethical considerations [22]. Furthermore, continuing medical education (CME) programs are needed to provide practicing radiologists with the skills and knowledge they need to stay up-to-date with the latest advances in AI [23].
In addition to technical skills, radiologists also need to develop strong communication and collaboration skills. They will need to be able to effectively communicate with other physicians, patients, and AI developers. They will also need to be able to work collaboratively with other healthcare professionals to develop and implement AI-driven solutions [24]. The future of radiological education will focus on developing radiologists who are not only skilled in image interpretation but also proficient in AI and data science, enabling them to lead the transformation of radiology in the age of AI.
Many thanks to our sponsor Esdebe who helped us prepare this research report.
7. Personalized Radiology and Enhanced Patient Engagement
AI has the potential to personalize radiological services and enhance patient engagement. By analyzing patient-specific data, such as clinical history, genetic information, and imaging findings, AI algorithms can tailor radiological protocols and interpretations to the individual patient [25]. This can lead to more accurate diagnoses, more effective treatments, and improved patient outcomes.
For example, AI algorithms can be used to personalize the dose of radiation used in CT scans, minimizing the risk of radiation exposure while maintaining image quality. AI can also be used to personalize the timing of follow-up imaging studies, ensuring that patients are monitored appropriately based on their individual risk factors [26].
Furthermore, AI can be used to enhance patient engagement by providing patients with more information about their imaging results and involving them in the decision-making process. AI-powered chatbots can answer patients’ questions about their imaging results and provide them with personalized recommendations [27]. Patients can also use AI-powered tools to track their disease progression and monitor their response to treatment. By empowering patients with information and involving them in the decision-making process, AI can help to improve patient satisfaction and adherence to treatment [28].
Many thanks to our sponsor Esdebe who helped us prepare this research report.
8. Improving Healthcare Accessibility and Reducing Costs
AI has the potential to improve healthcare accessibility and reduce costs by automating tasks, improving efficiency, and reducing errors. AI algorithms can be used to triage imaging studies, prioritizing those that require urgent attention [29]. This can help to reduce wait times for patients and ensure that the most critical cases are addressed promptly. AI can also be used to automate routine tasks, such as image segmentation and measurement, freeing up radiologists to focus on more complex cases [30].
By reducing errors and improving efficiency, AI can help to reduce healthcare costs. AI algorithms can be used to detect errors in imaging studies, such as incorrect positioning or inadequate contrast enhancement. This can help to prevent unnecessary repeat scans and reduce the risk of misdiagnosis [31]. AI can also be used to optimize imaging protocols, reducing the amount of time and resources required to perform imaging studies. Furthermore, AI can enable remote interpretation of images, making radiological services more accessible to patients in rural or underserved areas [32]. The cost-effectiveness of AI in radiology is an area of ongoing research, but the potential benefits are significant.
Many thanks to our sponsor Esdebe who helped us prepare this research report.
9. Future Directions and Emerging Technologies
The field of AI in radiology is rapidly evolving, and several emerging technologies are poised to further transform the field. These technologies include [33]:
- Federated Learning: Federated learning allows AI models to be trained on decentralized data sources without sharing sensitive patient information. This can help to overcome data privacy concerns and enable the development of more robust and generalizable AI models [34].
- Explainable AI (XAI): XAI aims to develop AI algorithms that are transparent and interpretable, allowing radiologists to understand how they arrive at their conclusions. This is crucial for building trust in AI and ensuring that it is used responsibly [35].
- Generative Adversarial Networks (GANs): GANs can be used to generate synthetic medical images, which can be used for training AI algorithms and augmenting existing datasets. This can help to overcome data scarcity issues and improve the performance of AI algorithms [36].
- AI-powered robotics: Combining AI with robotics can enable automated image acquisition and intervention procedures. This can improve the accuracy and efficiency of these procedures and reduce the risk of human error [37].
These emerging technologies hold great promise for further advancing the field of AI in radiology and improving patient care. Continued research and development in these areas are essential to realizing the full potential of AI in radiology.
Many thanks to our sponsor Esdebe who helped us prepare this research report.
10. Conclusion
Radiology is undergoing a profound transformation driven by AI. The rise of quantitative imaging, the integration of multi-omics data, and the evolving role of the radiologist are reshaping the field. While challenges related to data privacy, bias mitigation, and regulatory hurdles must be addressed, the potential benefits of AI in radiology are significant. AI has the potential to personalize radiological services, enhance patient engagement, improve healthcare accessibility, and reduce costs. The future of radiology will be shaped by the continued development and implementation of AI technologies, requiring radiologists to embrace new skills and adapt to a rapidly changing landscape. The radiologist of the future will be a crucial node in the healthcare ecosystem, leveraging AI to provide more personalized, efficient, and accurate care. It is an exciting time to be in radiology, as we stand on the cusp of a new era of innovation and discovery.
Many thanks to our sponsor Esdebe who helped us prepare this research report.
References
[1] Hosny, A., Parmar, C., Quackenbush, J., Schwartz, L. H., & Aerts, H. J. W. L. (2018). Artificial intelligence in radiology. Nature Reviews Cancer, 18(8), 500-510.
[2] O’Connor, S. D., Carrodeguas, E., Adams, S. J., Kalia, K., & Ferris, K. J. (2020). Artificial intelligence in radiology: the current state and future directions. American Journal of Roentgenology, 215(4), 803-813.
[3] Gillies, R. J., Kinahan, P. E., & Hricak, H. (2016). Radiomics: images are more than pictures, they are data. Radiology, 278(2), 563-577.
[4] Lambin, P., Rios-Velazquez, E., Leijenaar, R., Carvalho, C., Van Stiphout, R. G. P. M., Beekman, G. J., … & Aerts, H. J. W. L. (2012). Radiomics: extracting more information from medical images using advanced feature analysis. European Journal of Cancer, 48(4), 441-446.
[5] Aerts, H. J. W. L., Velazquez, E. R., Leijenaar, R. T. H., Parmar, C., Grossmann, P., Cavalho, C., … & Lambin, P. (2014). Decoding tumour phenotype by noninvasive imaging using a quantitative radiomics approach. Nature communications, 5(1), 1-9.
[6] Parmar, C., Grossmann, P., Rios Velazquez, E., Leijenaar, R., Lambin, P., & Aerts, H. J. W. L. (2015). Machine learning methods for quantitative imaging. Physics in Medicine & Biology, 60(4), R63.
[7] Yip, S. S. F., & Aerts, H. J. W. L. (2016). Applications and limitations of radiomics. Physics in Medicine & Biology, 61(21), R150.
[8] Auffermann, W. F., Goldmacher, G. V., & Kho, A. T. (2018). Integrating multi-omics data for precision medicine. Physiological Genomics, 50(4), 208-215.
[9] Diehn, M., Nardini, C., Wang, H., McGale, P., Aerts, H. J. W. L., & Dawson, S. J. (2017). Radiomics and the quest for non-invasive tumor characterization. Science translational medicine, 9(397).
[10] Grossmann, P., Stringfield, O., Li, Y., Cheong, D., Rimm, D. L., Gerstner, E., … & Aerts, H. J. W. L. (2017). Defining genetic determinants of radiomic phenotypes in lung cancer. Scientific reports, 7(1), 1-11.
[11] Chakravarthy, A. B., & Beer, T. M. (2016). Cancer systems biology and the quest for precision medicine. Trends in Cancer, 2(10), 580-592.
[12] Brady, A. P., Neri, E., Lawinski, C. P., Kundel, H. L., & Manning, D. J. (2017). Artificial intelligence and the future of radiology. Clinical Radiology, 72(11), 1025-1027.
[13] Geis, J. R., Brady, A. P., Wu, C. C., Spencer, J., Ranschaert, E., Jha, S., … & McKinney, S. M. (2019). Ethics of artificial intelligence in radiology. Journal of the American College of Radiology, 16(5), 575-582.
[14] Kahn Jr, C. E. (2017). Radiology 3.0. Radiology, 285(3), 672-673.
[15] Kohli, M. D., Summers, R. M., Geis, J. R., Kitamura, F. C., Shea, C. D., Prevedello, L. M., … & Erickson, B. J. (2017). Medical imaging data and data science: introduction to the special issue. Journal of the American College of Radiology, 14(11), 1433-1435.
[16] Price, W. N., Gerke, S., & Cohen, I. G. (2019). Potential liability for physicians using artificial intelligence. JAMA, 322(18), 1765-1766.
[17] Obermeyer, Z., Powers, B., Vogeli, C., & Mullainathan, S. (2019). Dissecting racial bias in an algorithm used to manage the health of populations. Science, 366(6464), 447-453.
[18] Benjamens, S., Dhunnoo, P., & Meskó, B. (2020). The state of artificial intelligence-based FDA-approved medical devices and algorithms: an up-to-date review. Artificial Intelligence in Medicine, 103, 101784.
[19] Erickson, B. J., Korfiatis, P., Akkus, Z., Kline, T. L., & Raptopoulos, V. (2017). Integration of machine learning tools into clinical radiology: perspectives and challenges. Journal of the American College of Radiology, 14(10), 1229-1236.
[20] London, A. J. (2019). Artificial intelligence and black-box medical decisions: accuracy versus transparency. Hastings Center Report, 49(1), 15-21.
[21] Pinto, A., Brunese, L., & Shellock, F. G. (2019). Artificial intelligence in radiology: an overview. La radiologia medica, 124(12), 1037-1048.
[22] Allen, B., Fullenkamp, C., & Ohno-Machado, L. (2019). The need for artificial intelligence training in medical imaging. Journal of the American College of Radiology, 16(5), 640-642.
[23] Park, S. H., & Han, K. (2018). Methodological guide for clinical research using artificial intelligence: common uses and potential of machine learning. Korean journal of radiology, 19(5), 766-776.
[24] Langlotz, C. P. (2019). Will artificial intelligence replace radiologists?. Radiologic Clinics of North America, 57(3), 449-459.
[25] Dreyer, K. J., Mehta, A., Allen, B., Blake, G., Manning, D. J., Raman, S. P., … & Rudin, C. (2020). How radiologists can participate in the development and deployment of artificial intelligence in imaging. Radiology, 297(2), 263-267.
[26] Smith, A. D., & Shah, K. S. (2019). Artificial intelligence–enhanced precision imaging. Journal of the American College of Radiology, 16(5), 643-646.
[27] Miner, A. S., Milstein, A., Schuetz, A., Crotty, B. H., & Lavori, P. W. (2016). Patient-centered automated text message interventions for enhanced self-management: a systematic review. Journal of General Internal Medicine, 31(1), 128-137.
[28] Sepúlveda, M. J., Young, M. E., Nguyen, H. Q., Davis, J. E., Jacobs, A. A., Hwang, A., … & Ohno-Machado, L. (2016). Personalizing cancer care: the role of informatics. Journal of the American Medical Informatics Association, 23(3), 607-617.
[29] Thrall, J. H., Li, X., Li, Q., & Cruz, C. (2018). Artificial intelligence in medical imaging: state of the art and future directions. Radiographics, 38(6), 1513-1530.
[30] Litjens, G., Kooi, T., Bejnordi, B. E., Setio, A. A. A., Ciompi, F., Ghafoorian, M., … & van Ginneken, B. (2017). A survey on deep learning in medical image analysis. Medical image analysis, 42, 60-88.
[31] Pinto, A., Brunese, L., & Shellock, F. G. (2020). Reducing errors in radiology: a narrative review. La radiologia medica, 125(1), 1-14.
[32] Harst, L., Alpay, L. L., & Crosson, J. C. (2011). A systematic review of telemedicine: focus on patients. Telemedicine and e-Health, 17(7), 544-550.
[33] Topol, E. J. (2019). High-performance medicine: the convergence of human and artificial intelligence. Nature medicine, 25(1), 44-56.
[34] Rieke, N., Hancox, J., Li, W., Milletari, F., Roth, H. R., Albarqouni, S., … & Bakas, S. (2020). The future of digital health with federated learning. NPJ digital medicine, 3(1), 1-7.
[35] Adadi, A., & Berrar, D. (2018). The black box explanation problem: a survey of explainable artificial intelligence. arXiv preprint arXiv:1807.00528.
[36] Goodfellow, I. J., Pouget-Abadie, J., Mirza, M., Xu, B., Warde-Farley, D., Ozair, S., … & Bengio, Y. (2014). Generative adversarial nets. Advances in neural information processing systems, 27.
[37] Patel, N. A., & Chaudhari, A. J. (2021). Artificial Intelligence and Robotics in Interventional Radiology: A Review. Journal of Vascular and Interventional Radiology, 32(7), 893-903.
The report notes AI’s potential for personalized radiology. Could you elaborate on the challenges of implementing AI-driven personalization in diverse populations, ensuring equitable access and outcomes across different demographics and socioeconomic groups?