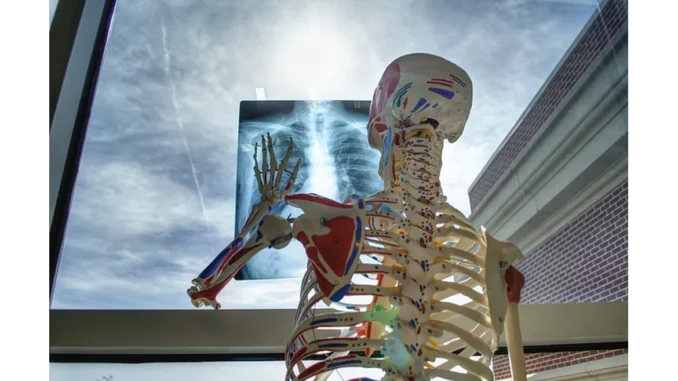
The advent of artificial intelligence in healthcare has heralded a new era in diagnostics and personalised medicine, offering capabilities previously thought unattainable. Yet, a recent study published in Nature: Scientific Reports highlights a disconcerting aspect of AI’s use in medical imaging: shortcut learning. This investigation, conducted by researchers at Dartmouth-Hitchcock Medical Center and the Geisel School of Medicine, reveals that AI systems can predict seemingly unrelated traits, such as dietary habits, from knee X-rays. While this finding is intriguing, it underscores the potential pitfalls of relying on AI in medical settings.
The study utilised convolutional neural networks (CNNs) to scrutinise over 25,000 knee X-rays from the Osteoarthritis Initiative (OAI) dataset. These models were trained to predict whether individuals consumed beer or avoided refried beans. Surprisingly, the models achieved a moderate degree of accuracy, with an area under the curve (AUC) of 0.73 for beer consumption and 0.63 for refried bean avoidance. However, these results do not suggest that dietary preferences are somehow inscribed in knee anatomy. Instead, they reveal how AI models exploit confounding variables—irrelevant correlations in data that have little to do with the primary prediction task. This phenomenon, known as shortcut learning, occurs when AI models identify patterns that provide quick solutions rather than substantive insights.
The researchers discovered that the AI models relied on subtle differences linked to clinical sites, X-ray machine manufacturers, and imaging protocols, rather than the knee anatomy itself. Saliency maps, which help visualise model decision-making, indicated that predictions were based on image artefacts, such as laterality markers and blacked-out sections intended for patient health indicators. The implications of these findings are profound. While AI has the potential to uncover non-obvious information in medical images, it can also learn misleading correlations that jeopardise the validity of clinical findings. This issue is far from isolated; previous studies have demonstrated that AI can deduce a patient’s race, age, and gender from medical images with remarkable accuracy, often relying on similar shortcut learning methods.
The dual-edged nature of AI—its ability to detect patterns invisible to humans and its susceptibility to misinterpretation—presents significant challenges. The study found that CNNs trained to predict dietary preferences could also effectively identify patient demographics. When re-tasked, the models could predict gender, race, and clinical site with high accuracy, illustrating how intertwined latent variables can skew predictions. This underscores the necessity for caution when interpreting AI outputs in medical settings. Researchers and clinicians must ensure that models are not merely capturing superficial patterns. Shortcut learning can lead to erroneous conclusions, undermining trust in AI-driven diagnostics and treatments.
Moreover, the study challenges the notion that preprocessing or normalising data is sufficient to eliminate biases. Despite efforts to standardise images, the models still leveraged latent variables to make predictions, highlighting the complexity of thoroughly addressing shortcut learning. As AI becomes increasingly integrated into healthcare, understanding its limitations is imperative. Models trained on medical images should undergo meticulous evaluation to ensure they learn meaningful patterns, rather than shortcuts. Techniques like saliency mapping should be employed to comprehend model behaviour and identify potential sources of bias. Accuracy metrics alone are inadequate; researchers must investigate whether a model’s predictions align with established medical principles.
Furthermore, regulatory bodies may need to establish guidelines for evaluating AI models in healthcare, concentrating on mitigating risks associated with shortcut learning. The study’s authors advocate for greater interdisciplinary collaboration to address these challenges. By combining the expertise of data scientists, clinicians, and ethicists, the medical community can develop robust AI systems that deliver on their promise without compromising reliability. Such a multidisciplinary approach is essential in contending with the dual-edged nature of AI in medicine.
In the end, the notion of a knee X-ray revealing dietary habits may elicit amusement, but it also serves as a poignant reminder of AI’s limitations. As researchers continue to push the boundaries of what AI can achieve, it is crucial to exercise caution to avoid the perils of shortcut learning, thus ensuring the integrity and accuracy of AI-driven insights in healthcare.
Be the first to comment