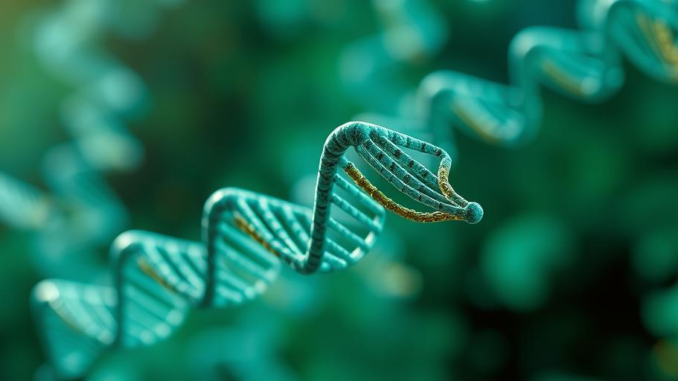
Summary
University of Florida researchers developed PhyloFrame, an AI-powered tool designed to address ancestral bias in genetic data. This tool integrates large databases of healthy human genomes with smaller, disease-specific datasets to improve the accuracy of disease prediction, diagnosis, and treatment across diverse populations. PhyloFrame is expected to significantly enhance precision medicine by ensuring medical research and treatments better serve people of all backgrounds.
Healthcare data growth can be overwhelming scale effortlessly with TrueNAS by Esdebe.
** Main Story**
Alright, let’s talk about this new AI tool coming out of the University of Florida, PhyloFrame. It’s aimed at tackling a problem we’ve all been aware of – ancestral bias in genetic data. The goal? To push precision medicine forward for everyone.
The Ancestry Conundrum
So, here’s the deal: precision medicine, while incredibly promising, has a bit of a diversity issue. Right now, genomic datasets are heavily skewed toward folks of European descent. What that means, practically, is that precision medicine might not be as effective for people from other backgrounds. It’s like trying to tailor a suit with only one set of measurements. Won’t work for everyone, will it?
I remember talking to a colleague in oncology, and she was telling me about how a particular genetic study just wasn’t relevant to many of her patients. That’s the kind of frustration Dr. Kiley Graim, the lead researcher behind PhyloFrame, is trying to address, apparently.
PhyloFrame: Leveling the Playing Field
PhyloFrame’s approach is pretty smart. It uses machine learning to pull together massive databases of healthy human genomes, like gnomAD, with the smaller, disease-specific datasets that we use to train precision medicine models. By injecting this ancestral diversity, PhyloFrame can develop models that are better at handling a wider range of genetic backgrounds. Therefore, it’s theoretically resulting in more accurate predictions and personalized treatments for all patients, no matter their ancestry. Sounds good, right?
Diving into the Tech
How does it actually work, though? Well, PhyloFrame leans on some pretty sophisticated machine learning algorithms to correct for that ancestral bias, integrating functional interaction networks, population genomics data, and transcriptomic training data. It’s like a complex recipe, honestly, but the end result is that it improves the accuracy of disease prediction, particularly for those underrepresented groups, because it actually considers genetic differences linked to ancestry. This tool has already shown some pretty impressive improvements in predictive power across different ancestries, reduced model overfitting – which is always a win – and even a higher likelihood of spotting known cancer-related genes. Pretty neat.
Proof in the Pudding
To make sure it wasn’t just hype, the researchers put PhyloFrame through its paces using genomic data from breast, thyroid, and uterine cancers. And guess what? It outperformed benchmark models across a bunch of different metrics and, crucially, corrected for that ancestral bias. Even better, it was more successful than existing tools at making accurate predictions even when looking at individuals from ancestries that are super underrepresented in the data, like, say, East African populations.
Supercomputing Power
But, processing all that data, you can imagine, requires some serious computing muscle. The team is using the University of Florida’s HiPerGator, which is one of the most powerful supercomputers in the country, to crunch all those numbers. I mean, we’re talking about analyzing genomic information from millions of individuals, processing billions of base pairs of DNA for each person. It’s amazing. Dr. Graim and her doctoral student, Leslie Smith, even said they were surprised at how well it worked! The project started small but has since secured more funding to develop even more sophisticated models and really fine-tune those population definitions.
What This Means for the Future
PhyloFrame, honestly, feels like a big step forward in making precision medicine truly equitable. By taking ancestral diversity into account, it helps to close those gaps in healthcare outcomes and makes sure that precision medicine is actually beneficial for everyone, regardless of their background. It’s pretty crucial for personalizing care across diverse populations and boosting the overall accuracy of precision medicine for all patients. So, with support from the National Institutes of Health, this could really revolutionize how we approach disease prediction, diagnosis, and treatment and improve healthcare outcomes.
- Diverse training data improves model accuracy even for those with European ancestry.
- Dr. Graim and her collaborators are planning to implement PhyloFrame in clinical trials.
And honestly? This is where AI really shines, isn’t it? Using its power to build a more equitable and effective healthcare system for the future. Exciting stuff!
So, HiPerGator is doing all the heavy lifting? Does that mean my laptop barely runs Minesweeper has zero chance of ever curing cancer? Asking for a friend…with a *really* slow laptop.
That’s a funny thought! While HiPerGator definitely speeds things up for large-scale analyses like this, who knows what innovations could come from tinkering, even on a slower machine? Every big discovery starts somewhere! Keep exploring!
Editor: MedTechNews.Uk
Thank you to our Sponsor Esdebe
So, PhyloFrame uses supercomputers to be inclusive? Finally, a legitimate excuse to upgrade my gaming rig… for *science*, of course. Wonder if it can also predict which games I’ll rage quit? Asking for, uh, *me*.
That’s a great point! While supercomputers are essential for handling massive datasets, accessible tools and creative problem-solving are equally crucial for advancing research. Who knows, maybe your upgraded rig could unlock unexpected insights! #PrecisionMedicine #AIHealth
Editor: MedTechNews.Uk
Thank you to our Sponsor Esdebe
The focus on diverse datasets in PhyloFrame is critical. Ensuring representation from various ancestral backgrounds will likely improve not just disease prediction but also drug response predictions across different populations.
Absolutely! You’ve hit on a key point. The implications extend beyond disease prediction; personalized drug response is a huge area. Understanding how different genetic backgrounds affect drug efficacy could revolutionize treatment plans across all populations. Let’s hope PhyloFrame leads the way!
Editor: MedTechNews.Uk
Thank you to our Sponsor Esdebe
Given the reliance on HiPerGator, how scalable is PhyloFrame for institutions without access to similar supercomputing resources? Could cloud-based solutions offer a viable alternative for broader accessibility and implementation?
That’s a really important question! While HiPerGator was key for initial development, exploring cloud-based solutions is absolutely the next step for widespread accessibility. We’re actively investigating strategies to optimize PhyloFrame for more institutions and researchers, potentially through modular design or tiered access. Thanks for sparking this discussion!
Editor: MedTechNews.Uk
Thank you to our Sponsor Esdebe
Precision medicine for *everyone*, huh? So, if I submit my 23andMe data, will PhyloFrame finally tell me why I’m so bad at parallel parking? Asking for a friend… who keeps getting parking tickets.