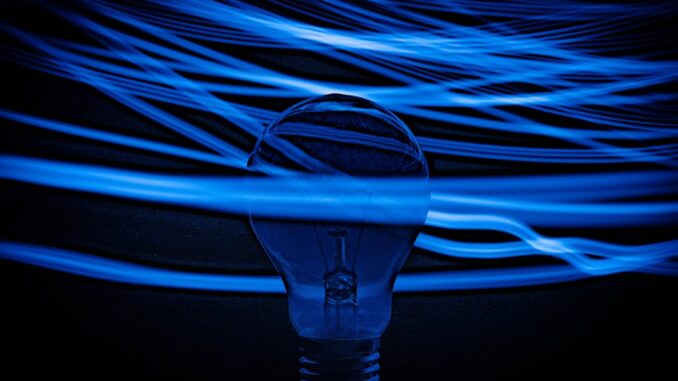
Abstract
Brain waves, the rhythmic electrical activity generated by synchronized neuronal populations, serve as a fundamental marker of brain state and function. This report provides a comprehensive review of brain waves, encompassing their neurophysiological origins, frequency bands (delta, theta, alpha, beta, and gamma), spatiotemporal dynamics, and functional roles in cognition, behavior, and clinical conditions. We explore the mechanisms underlying the generation and modulation of brain waves, including the roles of intrinsic neuronal properties, synaptic interactions, and network connectivity. Furthermore, we delve into the cognitive functions associated with different brain wave frequencies, such as delta waves in sleep, theta waves in memory and navigation, alpha waves in attention and relaxation, beta waves in active thinking, and gamma waves in sensory processing and cognitive integration. This review also discusses the application of brain wave analysis in clinical settings, including the diagnosis and monitoring of neurological and psychiatric disorders. Finally, we examine the use of advanced signal processing techniques and artificial intelligence (AI) algorithms in the analysis and interpretation of brain wave data, highlighting the potential for personalized medicine and novel therapeutic interventions.
Many thanks to our sponsor Esdebe who helped us prepare this research report.
1. Introduction
The human brain, a complex and dynamic organ, generates electrical activity that can be measured non-invasively using electroencephalography (EEG) or magnetoencephalography (MEG). These recordings reveal rhythmic fluctuations in electrical potential, commonly referred to as brain waves. These oscillations reflect the synchronized activity of large populations of neurons and provide a window into the underlying neural processes that govern cognition, behavior, and consciousness. Brain waves are not merely epiphenomena; they actively contribute to brain function by orchestrating neuronal communication, regulating synaptic plasticity, and shaping neural representations. Understanding the nature and function of brain waves is crucial for deciphering the neural code and developing effective interventions for neurological and psychiatric disorders.
The study of brain waves has a rich history, dating back to Hans Berger’s discovery of the human EEG in the 1920s (Berger, 1929). Since then, extensive research has characterized the different frequency bands of brain waves and their association with various brain states and cognitive processes. The five primary frequency bands are delta (0.5-4 Hz), theta (4-8 Hz), alpha (8-12 Hz), beta (12-30 Hz), and gamma (30-100 Hz). Each frequency band is associated with distinct neurophysiological mechanisms and functional roles. Furthermore, the amplitude, frequency, and spatial distribution of brain waves can vary significantly depending on the task, cognitive state, and individual differences.
This report aims to provide a comprehensive overview of brain waves, covering their neurophysiological origins, functional roles, clinical applications, and advanced analysis techniques. We will explore the mechanisms underlying the generation and modulation of brain waves, the cognitive functions associated with different frequency bands, the application of brain wave analysis in clinical settings, and the use of AI algorithms in the interpretation of brain wave data. This review is intended to be of interest to experts in the field of neuroscience, as well as researchers and clinicians interested in the study of brain waves.
Many thanks to our sponsor Esdebe who helped us prepare this research report.
2. Neurophysiological Basis of Brain Waves
Brain waves arise from the synchronized activity of large populations of neurons. The electrical activity of individual neurons is generated by the flow of ions across the cell membrane, primarily through ion channels. These ionic currents create local field potentials (LFPs), which represent the sum of electrical activity from multiple neurons in a local area. When a sufficient number of neurons fire synchronously, their LFPs summate and generate measurable brain waves. The precise mechanisms underlying the synchronization of neuronal activity are complex and involve a combination of intrinsic neuronal properties, synaptic interactions, and network connectivity.
2.1 Intrinsic Neuronal Properties
Intrinsic neuronal properties, such as membrane excitability, ion channel expression, and firing patterns, play a crucial role in shaping the frequency and amplitude of brain waves. Certain neurons, such as thalamic neurons, possess intrinsic oscillatory properties that allow them to generate rhythmic activity even in the absence of external input (Llinás, 1988). These intrinsic oscillations can be modulated by synaptic input and contribute to the generation of specific brain wave frequencies.
2.2 Synaptic Interactions
Synaptic interactions between neurons are essential for synchronizing neuronal activity and generating brain waves. Excitatory and inhibitory synaptic connections can create feedback loops that promote rhythmic firing patterns. For example, the interplay between excitatory pyramidal neurons and inhibitory interneurons in the cortex is thought to be critical for generating alpha and gamma oscillations (Buzsáki, 2006). The balance between excitation and inhibition (E/I balance) is crucial for maintaining stable brain activity and preventing pathological conditions such as epilepsy.
2.3 Network Connectivity
The architecture of neuronal networks also influences the generation and propagation of brain waves. The brain is organized into hierarchical networks with interconnected nodes and pathways. The connectivity between different brain regions allows for the coordinated activity of distributed neuronal populations and the generation of large-scale brain waves. White matter tracts, which connect distant brain regions, play a crucial role in transmitting electrical signals and synchronizing activity across the brain. The disruption of network connectivity can lead to abnormal brain wave patterns and cognitive dysfunction.
Many thanks to our sponsor Esdebe who helped us prepare this research report.
3. Brain Wave Frequency Bands and Cognitive Functions
Brain waves are typically classified into five main frequency bands: delta (0.5-4 Hz), theta (4-8 Hz), alpha (8-12 Hz), beta (12-30 Hz), and gamma (30-100 Hz). Each frequency band is associated with distinct neurophysiological mechanisms and functional roles. However, it is important to note that the boundaries between these frequency bands are not always clear-cut, and there can be considerable overlap in their functional roles.
3.1 Delta Waves (0.5-4 Hz)
Delta waves are the slowest brain waves and are predominantly observed during deep sleep (stages 3 and 4 of non-rapid eye movement (NREM) sleep). They are generated by synchronized activity in the thalamocortical network and are thought to play a role in sleep consolidation and restorative processes (Nir et al., 2011). Delta waves are also observed in infants and young children, as well as in certain pathological conditions such as coma and brain injury.
3.2 Theta Waves (4-8 Hz)
Theta waves are associated with various cognitive processes, including memory, navigation, and attention. They are prominently observed in the hippocampus, a brain region critical for spatial learning and memory. Theta oscillations in the hippocampus are thought to play a role in encoding and retrieving spatial information (Buzsáki, 2005). Theta waves are also observed during meditation and relaxation, as well as in certain sleep stages (stage 1 and 2 of NREM sleep).
3.3 Alpha Waves (8-12 Hz)
Alpha waves are characterized by their prominence during relaxed wakefulness with eyes closed. They are generated by synchronized activity in the visual cortex and are thought to reflect a state of cortical idling or inhibition (Pfurtscheller, 1992). Alpha waves are suppressed by visual attention and cognitive tasks. The amplitude of alpha waves is inversely correlated with cortical activity, meaning that higher alpha amplitude indicates lower cortical activity. Alpha waves are also thought to play a role in sensory gating and filtering out irrelevant information.
3.4 Beta Waves (12-30 Hz)
Beta waves are associated with active thinking, problem-solving, and attention. They are observed during focused concentration and cognitive tasks. Beta waves are generated by synchronized activity in the frontal cortex and are thought to reflect a state of cortical activation. Beta waves are also associated with anxiety and stress, and their amplitude can be increased by caffeine and other stimulants. Importantly, beta waves are also linked to motor control.
3.5 Gamma Waves (30-100 Hz)
Gamma waves are the fastest brain waves and are associated with sensory processing, cognitive integration, and consciousness. They are thought to play a role in binding together different features of an object or scene into a coherent percept (Singer, 1999). Gamma oscillations are observed in various brain regions, including the visual cortex, auditory cortex, and prefrontal cortex. Gamma waves are also associated with attention, memory, and decision-making. However, the precise functional role of gamma waves is still a subject of ongoing research, with some researchers arguing that gamma waves may be a byproduct of neuronal activity rather than a functional signal.
Many thanks to our sponsor Esdebe who helped us prepare this research report.
4. Brain Waves in Sleep
Sleep is a complex physiological state characterized by distinct brain wave patterns. The sleep cycle consists of two main stages: non-rapid eye movement (NREM) sleep and rapid eye movement (REM) sleep. NREM sleep is further divided into four stages: stage 1, stage 2, stage 3, and stage 4. Each sleep stage is associated with distinct brain wave patterns.
4.1 NREM Sleep
- Stage 1: This is the transition stage between wakefulness and sleep. Brain waves slow down, and alpha waves are replaced by theta waves. Individuals in stage 1 sleep may experience hypnic jerks (sudden muscle contractions).
- Stage 2: This stage is characterized by the presence of sleep spindles (bursts of high-frequency activity) and K-complexes (sharp, negative deflections in the EEG). Sleep spindles are thought to play a role in sleep consolidation, while K-complexes may represent a response to external stimuli.
- Stage 3: This stage is marked by the appearance of delta waves, which gradually increase in amplitude and prevalence. Stage 3 sleep is also known as slow-wave sleep (SWS) or deep sleep.
- Stage 4: This is the deepest stage of sleep, characterized by a predominance of delta waves. During stage 4 sleep, individuals are difficult to awaken and may experience sleepwalking or night terrors.
4.2 REM Sleep
REM sleep is characterized by rapid eye movements, muscle atonia (paralysis of skeletal muscles), and vivid dreaming. The EEG during REM sleep resembles that of wakefulness, with a mixture of theta, alpha, and beta waves. REM sleep is thought to play a role in memory consolidation and emotional processing.
4.3 Abnormal Brain Wave Activity in Sleep
Abnormal brain wave activity during sleep can indicate various sleep disorders, such as insomnia, sleep apnea, and narcolepsy. For example, individuals with insomnia may exhibit increased beta activity and decreased delta activity during sleep. Individuals with sleep apnea may experience frequent arousals from sleep, which are associated with changes in brain wave patterns. Individuals with narcolepsy may enter REM sleep prematurely, without progressing through the normal NREM sleep stages.
Many thanks to our sponsor Esdebe who helped us prepare this research report.
5. Clinical Applications of Brain Wave Analysis
Brain wave analysis has numerous clinical applications, including the diagnosis and monitoring of neurological and psychiatric disorders. EEG is a widely used diagnostic tool for epilepsy, sleep disorders, and other neurological conditions. MEG is a more advanced technique that provides higher spatial resolution and is particularly useful for localizing seizure foci.
5.1 Epilepsy
Epilepsy is a neurological disorder characterized by recurrent seizures. EEG is the primary diagnostic tool for epilepsy, as it can detect abnormal brain wave activity associated with seizures, such as spikes, sharp waves, and slow waves. EEG can also be used to classify different types of seizures and to monitor the effectiveness of anti-epileptic medications.
5.2 Sleep Disorders
Brain wave analysis is essential for diagnosing and managing sleep disorders. Polysomnography (PSG), a comprehensive sleep study that includes EEG, electromyography (EMG), and electrooculography (EOG), is used to assess sleep architecture, identify sleep stages, and detect abnormal brain wave activity during sleep. PSG is used to diagnose various sleep disorders, such as insomnia, sleep apnea, narcolepsy, and restless legs syndrome.
5.3 Psychiatric Disorders
Brain wave analysis is increasingly being used in the study of psychiatric disorders, such as depression, anxiety, and schizophrenia. EEG studies have shown that individuals with depression may exhibit altered alpha activity and reduced delta activity. Individuals with anxiety may exhibit increased beta activity. Individuals with schizophrenia may exhibit abnormal gamma activity and reduced coherence between different brain regions.
5.4 Brain-Computer Interfaces (BCIs)
Brain-computer interfaces (BCIs) are devices that allow individuals to control external devices using their brain activity. BCIs typically rely on EEG to detect and interpret brain wave patterns. BCIs have been used to develop assistive technologies for individuals with paralysis, such as wheelchair control and communication devices. BCIs are also being explored for use in gaming, education, and other applications.
Many thanks to our sponsor Esdebe who helped us prepare this research report.
6. Advanced Analysis Techniques and Artificial Intelligence
Advanced signal processing techniques and artificial intelligence (AI) algorithms are increasingly being used to analyze and interpret brain wave data. These techniques can extract meaningful information from complex brain wave patterns and improve the accuracy and efficiency of brain wave analysis.
6.1 Signal Processing Techniques
- Time-Frequency Analysis: Time-frequency analysis techniques, such as wavelet transform and short-time Fourier transform (STFT), can be used to analyze the frequency content of brain waves over time. These techniques can reveal transient changes in brain wave activity that may be missed by traditional EEG analysis.
- Connectivity Analysis: Connectivity analysis techniques, such as coherence and Granger causality, can be used to assess the functional connectivity between different brain regions based on brain wave activity. These techniques can reveal how different brain regions interact and communicate with each other.
- Source Localization: Source localization techniques, such as dipole modeling and beamforming, can be used to estimate the location of brain activity sources based on EEG or MEG data. These techniques can help to identify the brain regions that are generating specific brain wave patterns.
6.2 Artificial Intelligence Algorithms
- Machine Learning: Machine learning algorithms, such as support vector machines (SVMs) and artificial neural networks (ANNs), can be trained to classify different brain states based on brain wave data. These algorithms can be used to diagnose neurological and psychiatric disorders, predict seizure onset, and control BCIs.
- Deep Learning: Deep learning algorithms, such as convolutional neural networks (CNNs) and recurrent neural networks (RNNs), are particularly well-suited for analyzing complex brain wave data. These algorithms can automatically learn features from raw EEG or MEG data and achieve high accuracy in classification and prediction tasks. For example, AI models are now used to predict sleep stages, allowing sleep researchers to rapidly assess many hours of sleep data (e.g. PFTSleep analysis as noted in the prompt).
- Explainable AI (XAI): As AI algorithms become more complex, it is important to develop methods for understanding how these algorithms make decisions. Explainable AI (XAI) techniques can provide insights into the features that are most important for predicting brain states or diagnosing diseases. XAI can help to build trust in AI-based brain wave analysis systems and to improve the interpretability of results.
Many thanks to our sponsor Esdebe who helped us prepare this research report.
7. Conclusion
Brain waves are a fundamental marker of brain state and function. This report has provided a comprehensive review of brain waves, encompassing their neurophysiological origins, frequency bands, functional roles, clinical applications, and advanced analysis techniques. Understanding the nature and function of brain waves is crucial for deciphering the neural code and developing effective interventions for neurological and psychiatric disorders.
The study of brain waves is a rapidly evolving field, with ongoing research exploring the complex interactions between brain waves, cognition, behavior, and disease. Advanced signal processing techniques and AI algorithms are revolutionizing the way we analyze and interpret brain wave data, opening up new possibilities for personalized medicine and novel therapeutic interventions. Future research should focus on developing more sophisticated models of brain wave generation and modulation, exploring the role of brain waves in higher-level cognitive functions, and developing more effective BCIs for individuals with disabilities.
Many thanks to our sponsor Esdebe who helped us prepare this research report.
References
- Berger, H. (1929). Über das Elektrenkephalogramm des Menschen. Archiv für Psychiatrie und Nervenkrankheiten, 87(1), 527-570.
- Buzsáki, G. (2005). Theta rhythm and hippocampal path integration. Neuron, 46(3), 311-322.
- Buzsáki, G. (2006). Rhythms of the brain. Oxford University Press.
- Llinás, R. R. (1988). Intrinsic electrophysiological properties of mammalian neurons: insights into central nervous system function. Science, 242(4886), 1654-1664.
- Nir, Y., Tononi, G., & Cirelli, C. (2011). Sleep and synaptic homeostasis. Neuron, 69(4), 649-666.
- Pfurtscheller, G. (1992). Event-related synchronization (ERS): an electrophysiological correlate of cortical areas at rest. Electroencephalography and clinical neurophysiology, 83(1), 62-69.
- Singer, W. (1999). Neuronal synchrony: a versatile code for the definition of relations? Neuron, 24(1), 49-65, 111-125.
The point about AI models predicting sleep stages is fascinating. How might these advanced analyses, particularly deep learning, be further developed to personalize sleep interventions based on individual brain wave patterns?
That’s a great question! Personalizing sleep interventions using deep learning and brainwave patterns could revolutionize sleep medicine. Imagine AI tailoring soundscapes or gentle light patterns to nudge individuals into specific, restorative sleep stages based on real-time EEG analysis. Further research into individual variations and long-term efficacy is definitely needed!
Editor: MedTechNews.Uk
Thank you to our Sponsor Esdebe
Fascinating deep dive into brain waves! Makes you wonder if our thoughts are just complex symphonies playing out in our heads. Are we just conductors waving batons of consciousness?
Thanks for your insightful comment! The analogy of thoughts as complex symphonies is quite compelling. It sparks a thought, perhaps our brains are not just conductors but also composers, constantly creating and reinterpreting these symphonies based on our experiences and internal states. What do you think?
Editor: MedTechNews.Uk
Thank you to our Sponsor Esdebe
This comprehensive review highlights the fascinating potential of brain wave analysis in understanding cognitive functions. The discussion of gamma waves and their role in cognitive integration particularly intrigues me. Could advancements in decoding these complex patterns lead to new insights into consciousness itself?
Thank you for your insightful comment! The role of gamma waves in cognitive integration is indeed a hot topic. Exploring how advancements in decoding these patterns could unlock secrets about consciousness is definitely a fascinating avenue for future research! It would be interesting to explore the role of gamma wave synchronicity in the emergence of consciousness.
Editor: MedTechNews.Uk
Thank you to our Sponsor Esdebe
So, if our brains are constantly humming with these electrical symphonies, does that mean we can blame our brainwaves for those embarrassing song lyrics we can’t get out of our heads? Asking for a friend, of course!
That’s a hilarious question! It certainly raises the possibility that our brainwaves might be to blame for those pesky earworms. Perhaps specific wave patterns are more susceptible to catchy tunes? Further investigation is needed (for a friend, naturally!).
Editor: MedTechNews.Uk
Thank you to our Sponsor Esdebe