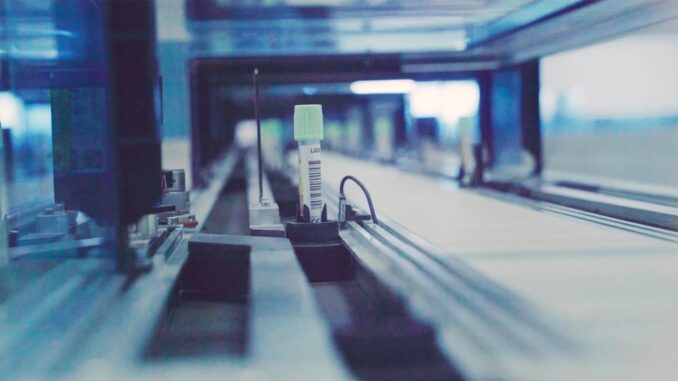
Abstract
The application of Artificial Intelligence (AI) in medical diagnostics has transcended the initial hype surrounding pattern recognition and image analysis. While these areas remain critical, a broader, more nuanced perspective is required to understand the true transformative potential and inherent limitations of AI in this field. This research report explores the multifaceted impact of AI on diagnostics, moving beyond simplistic comparisons with traditional methods. We delve into the evolution of AI diagnostic tools, encompassing not only image-based diagnostics but also predictive analytics, genomic interpretation, and personalized medicine approaches. The report critically assesses the challenges of implementing AI diagnostics in clinical settings, including regulatory complexities, data governance issues, and the critical need for explainable AI (XAI) to build trust and facilitate clinical acceptance. Furthermore, we address the ethical dimensions of AI-driven diagnostics, with a particular focus on algorithmic bias and its disproportionate impact on diverse populations, highlighting the urgent need for fairness-aware AI development and deployment. The report concludes by identifying key areas for future research and development, emphasizing the importance of interdisciplinary collaboration, robust validation methodologies, and a human-centered approach to ensure that AI serves as a powerful tool to enhance, rather than replace, the expertise of medical professionals.
Many thanks to our sponsor Esdebe who helped us prepare this research report.
1. Introduction: The Evolving Landscape of AI in Diagnostics
The integration of Artificial Intelligence (AI) into medical diagnostics represents a paradigm shift with the potential to revolutionize healthcare delivery. Initial enthusiasm centered on AI’s ability to automate and improve the accuracy of tasks such as image analysis in radiology and pathology, offering the promise of faster and more reliable diagnoses. However, the field has matured significantly, recognizing that the true value of AI lies in its capacity to augment human intelligence, uncover hidden patterns in complex datasets, and ultimately personalize diagnostic and treatment strategies.
This report aims to provide a comprehensive overview of the evolving landscape of AI in diagnostics, moving beyond the limited scope of individual applications and focusing on the broader systemic implications. We examine the diverse types of AI diagnostic tools, their performance characteristics, the challenges associated with their implementation, and the ethical considerations that must be addressed to ensure responsible and equitable deployment. The report also emphasizes the importance of integrating AI into existing clinical workflows, highlighting the need for robust validation studies, regulatory frameworks, and user-centered design to facilitate widespread adoption.
Furthermore, it is crucial to acknowledge the limitations of AI. AI systems are only as good as the data they are trained on, and biases present in the training data can lead to inaccurate or discriminatory diagnoses. The “black box” nature of some AI algorithms can also make it difficult to understand how they arrive at their conclusions, which can erode trust and hinder clinical acceptance. These challenges necessitate a critical and nuanced approach to AI development and deployment, emphasizing transparency, explainability, and fairness.
Many thanks to our sponsor Esdebe who helped us prepare this research report.
2. Types of AI Diagnostic Tools: A Broad Spectrum of Applications
AI is being applied to a wide range of diagnostic tasks, leveraging various machine learning techniques to extract meaningful insights from diverse data sources. This section examines some of the key types of AI diagnostic tools, highlighting their strengths, limitations, and potential for future development.
2.1 Image Analysis
Image analysis remains one of the most prominent applications of AI in diagnostics. Convolutional Neural Networks (CNNs) have demonstrated remarkable performance in tasks such as identifying cancerous lesions in medical images, detecting anomalies in X-rays, and segmenting organs in CT scans. Studies have shown that AI algorithms can achieve accuracy rates comparable to, and in some cases exceeding, those of human experts [1, 2].
However, it is important to note that the performance of AI image analysis tools can vary significantly depending on the quality and characteristics of the training data. CNNs are particularly sensitive to variations in image acquisition protocols, patient demographics, and disease prevalence. Therefore, robust validation studies are essential to ensure that these tools generalize well to different clinical settings.
2.2 Predictive Analytics
Predictive analytics leverages machine learning algorithms to identify patients at high risk of developing certain diseases or experiencing adverse events. These tools can analyze a wide range of data, including electronic health records (EHRs), genomic data, and social determinants of health, to generate personalized risk scores and inform preventive interventions.
For example, AI algorithms have been used to predict the likelihood of hospital readmissions, the risk of developing sepsis, and the prognosis of patients with chronic diseases [3, 4]. By identifying high-risk patients early on, clinicians can implement targeted interventions to improve outcomes and reduce healthcare costs.
2.3 Genomic Interpretation
The rapid advancement of genomic sequencing technologies has generated vast amounts of genomic data, creating new opportunities for personalized medicine. AI algorithms can analyze genomic data to identify genetic variants associated with disease risk, predict drug response, and guide treatment decisions.
AI-powered genomic interpretation tools can help clinicians identify patients who are likely to benefit from targeted therapies, avoid adverse drug reactions, and tailor treatment plans to individual patient characteristics [5, 6]. However, the interpretation of genomic data is complex and requires specialized expertise. Therefore, it is crucial to integrate AI genomic interpretation tools with clinical decision support systems to provide clinicians with actionable insights.
2.4 Personalized Medicine Approaches
AI is enabling a shift towards more personalized and precise medicine by integrating various data sources to create individualized diagnostic and treatment strategies. This involves analyzing patient-specific data, including genetic information, lifestyle factors, and environmental exposures, to develop tailored interventions that are most likely to be effective [7].
AI algorithms can be used to predict individual patient responses to different treatments, optimize drug dosages, and personalize rehabilitation programs. By tailoring healthcare interventions to individual patient needs, AI has the potential to improve outcomes, reduce adverse events, and enhance the overall quality of care.
Many thanks to our sponsor Esdebe who helped us prepare this research report.
3. Accuracy Rates and Comparison with Traditional Methods
Assessing the accuracy of AI diagnostic tools and comparing their performance to traditional methods is critical for determining their clinical utility and potential for widespread adoption. While numerous studies have demonstrated the potential of AI to improve diagnostic accuracy, it is important to consider the limitations of these studies and the challenges of conducting rigorous comparative analyses.
3.1 Performance Metrics
Several performance metrics are commonly used to evaluate the accuracy of AI diagnostic tools, including sensitivity, specificity, positive predictive value (PPV), negative predictive value (NPV), and area under the receiver operating characteristic curve (AUC-ROC). Sensitivity measures the ability of the AI algorithm to correctly identify individuals with the disease, while specificity measures the ability to correctly identify individuals without the disease. PPV and NPV indicate the probability that a positive or negative test result is truly positive or negative, respectively. AUC-ROC provides an overall measure of the diagnostic accuracy of the AI algorithm.
3.2 Comparative Studies
Numerous studies have compared the performance of AI diagnostic tools to that of human experts in various clinical domains. For example, several studies have shown that AI algorithms can achieve comparable or even superior accuracy to radiologists in detecting breast cancer on mammograms [8, 9]. Similarly, AI algorithms have demonstrated high accuracy in diagnosing diabetic retinopathy from retinal images, surpassing the performance of human graders in some studies [10].
However, it is important to note that these studies often involve highly specialized datasets and controlled settings, which may not accurately reflect real-world clinical practice. Furthermore, the performance of AI algorithms can be highly dependent on the quality and characteristics of the training data, and biases in the training data can lead to inaccurate or discriminatory diagnoses.
3.3 Challenges in Comparative Analysis
Conducting rigorous comparative analyses of AI diagnostic tools is challenging due to several factors. First, traditional diagnostic methods often involve a complex interplay of clinical judgment, experience, and contextual information, which is difficult to replicate in an AI algorithm. Second, the gold standard for diagnosis may not always be clear-cut, particularly in complex or ambiguous cases. Third, the performance of AI algorithms can be highly dependent on the specific patient population and clinical setting, making it difficult to generalize results across different populations and settings. Finally, the rapid pace of AI development means that algorithms are constantly being improved, making it difficult to conduct long-term comparative studies.
Many thanks to our sponsor Esdebe who helped us prepare this research report.
4. Challenges in Implementing AI Diagnostics in Clinical Settings
The successful implementation of AI diagnostics in clinical settings requires addressing a number of significant challenges, including regulatory hurdles, data privacy concerns, the need for explainable AI, and the integration of AI into existing clinical workflows.
4.1 Regulatory Hurdles
The regulatory landscape for AI-based medical devices is still evolving, and there is a lack of clear and consistent guidelines for the development, validation, and deployment of AI diagnostic tools. Regulatory agencies such as the FDA in the United States and the EMA in Europe are grappling with how to regulate AI algorithms that can learn and adapt over time, posing challenges to traditional regulatory frameworks that are based on fixed algorithms [11].
The lack of clear regulatory guidelines can create uncertainty for developers and manufacturers, hindering innovation and slowing down the adoption of AI diagnostics. There is a need for regulatory frameworks that are flexible, risk-based, and adaptable to the evolving nature of AI technology.
4.2 Data Privacy
AI diagnostic tools rely on large amounts of patient data, raising concerns about data privacy and security. The use of sensitive patient data for AI training and validation must comply with data privacy regulations such as HIPAA in the United States and GDPR in Europe. These regulations require that patient data be anonymized, protected from unauthorized access, and used only for specified purposes [12].
However, even anonymized data can potentially be re-identified using sophisticated data mining techniques. Therefore, it is crucial to implement robust data governance policies and security measures to protect patient privacy and prevent data breaches.
4.3 Explainable AI (XAI)
The “black box” nature of some AI algorithms can make it difficult to understand how they arrive at their conclusions. This lack of transparency can erode trust and hinder clinical acceptance. Explainable AI (XAI) aims to develop AI algorithms that are transparent, interpretable, and understandable to human users [13].
XAI techniques can help clinicians understand the reasoning behind AI-driven diagnoses, identify potential biases in the algorithms, and validate the accuracy of the results. By providing clinicians with insights into the inner workings of AI algorithms, XAI can enhance trust, facilitate collaboration, and improve the overall quality of care.
4.4 Integration into Clinical Workflows
The successful implementation of AI diagnostics requires seamless integration into existing clinical workflows. AI tools should be designed to complement, rather than replace, the expertise of medical professionals. Clinicians need to be trained on how to use AI tools effectively and how to interpret the results in the context of other clinical information.
AI diagnostic tools should be integrated into electronic health records (EHRs) and other clinical information systems to provide clinicians with easy access to relevant data. The user interface should be intuitive and user-friendly, and the AI algorithms should provide clear and concise explanations of their reasoning. Integration into workflows also requires clear definition of responsibility, whether the AI is a tool for the doctor or the doctor is a tool for the AI. Clear definition is necessary to avoid confusion.
Many thanks to our sponsor Esdebe who helped us prepare this research report.
5. Ethical Considerations: Bias, Fairness, and Accountability
The development and deployment of AI diagnostic tools raise a number of important ethical considerations, including algorithmic bias, fairness, and accountability. It is crucial to address these ethical issues proactively to ensure that AI is used responsibly and equitably in healthcare.
5.1 Algorithmic Bias
AI algorithms are trained on data, and biases present in the training data can be amplified and perpetuated by the algorithms. Algorithmic bias can lead to inaccurate or discriminatory diagnoses, particularly for underrepresented or marginalized populations [14].
For example, if an AI algorithm is trained primarily on data from one demographic group, it may not perform well on patients from other demographic groups. This can lead to disparities in healthcare outcomes and exacerbate existing health inequalities. Careful attention must be paid to the composition of training data to ensure that it is representative of the populations that the AI system will be used to diagnose. Data augmentation techniques may also be employed to balance datasets.
5.2 Fairness
Fairness in AI refers to the principle that AI algorithms should not discriminate against individuals or groups based on protected characteristics such as race, ethnicity, gender, or socioeconomic status. AI diagnostic tools should be designed and evaluated to ensure that they perform equitably across different demographic groups [15].
Several fairness metrics can be used to assess the fairness of AI algorithms, including demographic parity, equal opportunity, and predictive parity. These metrics measure different aspects of fairness and can help identify potential biases in the algorithms.
5.3 Accountability
Accountability refers to the principle that individuals or organizations should be held responsible for the decisions made by AI algorithms. When an AI algorithm makes an inaccurate or discriminatory diagnosis, it is important to determine who is responsible and how to remedy the situation [16].
Accountability in AI is a complex issue, as it involves questions of liability, transparency, and oversight. There is a need for clear legal and ethical frameworks that define the responsibilities of developers, manufacturers, and users of AI diagnostic tools.
Many thanks to our sponsor Esdebe who helped us prepare this research report.
6. Future Directions and Research Opportunities
The field of AI in diagnostics is rapidly evolving, and there are numerous opportunities for future research and development. Some of the key areas for future exploration include:
- Multimodal Data Integration: Developing AI algorithms that can integrate data from multiple sources, including imaging, genomics, clinical data, and social determinants of health, to provide a more comprehensive and personalized assessment of patient risk.
- Federated Learning: Developing AI algorithms that can be trained on decentralized data sources without sharing sensitive patient data, addressing data privacy concerns and enabling collaboration across institutions.
- Causal Inference: Developing AI algorithms that can infer causal relationships between risk factors and disease outcomes, providing insights into the underlying mechanisms of disease and informing targeted interventions.
- Continual Learning: Developing AI algorithms that can continuously learn and adapt to new data, improving their accuracy and generalizability over time.
- Human-AI Collaboration: Designing AI systems that facilitate seamless collaboration between humans and machines, leveraging the strengths of both to improve diagnostic accuracy and efficiency.
- Standardization and Validation: Establishing standardized methodologies for validating AI diagnostic tools, ensuring that they are accurate, reliable, and generalizable across different populations and settings.
- Ethical Frameworks: Developing ethical frameworks for the development and deployment of AI diagnostic tools, addressing issues of bias, fairness, and accountability.
Many thanks to our sponsor Esdebe who helped us prepare this research report.
7. Conclusion
The integration of AI into diagnostics holds immense promise for transforming healthcare delivery. AI has the potential to improve diagnostic accuracy, personalize treatment strategies, and reduce healthcare costs. However, realizing the full potential of AI in diagnostics requires a holistic approach that addresses not only the technical challenges but also the regulatory, ethical, and societal implications. As we move forward, it is crucial to prioritize transparency, explainability, and fairness in AI development and deployment, ensuring that these technologies serve as powerful tools to enhance, rather than replace, the expertise of medical professionals. It is also critical to remember that AI is a tool and not a replacement for qualified clinicians, AI should be used responsibly and ethically in the best interests of patients.
Many thanks to our sponsor Esdebe who helped us prepare this research report.
References
[1] Esteva, A., Kuprel, B., Novoa, R. A., Ko, J., Swani, S. M., Blau, H. M., … & Threlfall, C. J. (2017). Dermatologist-level classification of skin cancer with deep neural networks. Nature, 542(7639), 115-118.
[2] Gulshan, V., Peng, L., Coram, M., Stumpe, M. C., Wu, D., Narayanaswamy, A., … & Webster, D. R. (2016). Development and validation of a deep learning algorithm for detection of diabetic retinopathy in retinal fundus photographs. JAMA, 316(22), 2402-2410.
[3] Futoma, J., Hariharan, S., Heller, K., & Shah, N. H. (2015). An improved method for predicting 30-day readmissions. Journal of biomedical informatics, 58, 349-357.
[4] Escobar, G. J., Liu, V. X., Schuler, A., Lawson, B. T., Fee, C., Fremont, R. D., & Gardner, M. N. (2012). Assessment of a clinically comprehensive electronic sepsis prediction tool. Critical care medicine, 40(1), 17-26.
[5] Jameson, J. L., & Long, D. L. (2015). Deeper into genomics: the arc of precision medicine. JAMA, 313(9), 879-880.
[6] Hamburg, M. A., & Collins, F. S. (2010). The path to personalized medicine. New England Journal of Medicine, 363(4), 301-304.
[7] Mirnezami, R., Nicholson, J., & Darzi, A. (2012). Systems medicine: from phenotyping to mechanism. American Journal of Surgery, 203(4), 505-512.
[8] McKinney, S. M., Sieniek, M., Godbole, V., Godwin, J., Antropova, N., Ashrafian, H., … & Shetty, S. (2020). International evaluation of an AI system for breast cancer screening. Nature, 577(7788), 86-91.
[9] Rodriguez-Ruiz, A., Lång, K., Gubern-Mérida, A., Broeders, M., Gennaro, G., Clauser, P., … & Karssemeijer, N. (2019). Stand-alone artificial intelligence for breast cancer detection in mammography: comparison with 101 radiologists. European radiology, 29(9), 4824-4831.
[10] Abramoff, M. D., Gulshan, V., Quinn, T., Wu, J., Clarida, W., Lou, Y., … & Shah, N. H. (2018). Validation of a deep learning system for detecting diabetic retinopathy in India. JAMA ophthalmology, 136(4), 328-334.
[11] Meskó, B., Topol, E. J., & Moretti, F. A. (2018). Artificial intelligence in health care: scope, limitations, and future. Mayo Clinic Proceedings, 93(9), 1265-1279.
[12] Price, W. N., & Cohen, I. G. (2019). Privacy in the age of medical big data. Nature medicine, 25(1), 37-43.
[13] Holzinger, A., Langs, G., Denk, H., Zatloukal, K., & Müller, H. (2019). When do we need explainable AI (XAI) for healthcare? European Journal of Biomedical Informatics, 15(1).
[14] Obermeyer, Z., Powers, B., Vogeli, C., & Mullainathan, S. (2019). Dissecting racial bias in an algorithm used to manage the health of populations. Science, 366(6464), 447-453.
[15] Barocas, S., Hardt, M., & Narayanan, A. (2019). Fairness and machine learning: Limitations and opportunities. MIT Press.
[16] Mittelstadt, B. D. (2016). On the moral responsibility of connected things. The Age of Machines: Quo Vadis Humanitas?, 83-99.
Be the first to comment