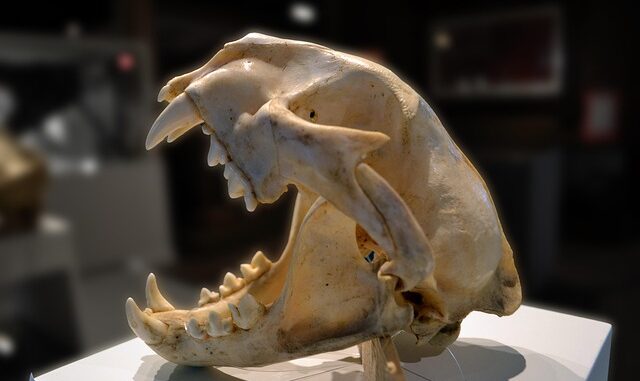
Deconstructing Death: A Multifaceted Exploration of Mortality and its Evolving Landscape
Abstract
Mortality, the ultimate and unavoidable endpoint of life, is a complex phenomenon shaped by a confluence of biological, social, economic, and environmental factors. This research report undertakes a comprehensive exploration of mortality, moving beyond simplistic definitions to examine its multifaceted nature and the evolving challenges in its study. We delve into historical trends, geographical variations, and the impact of disease, socioeconomic disparities, and environmental changes on mortality rates. Furthermore, we critically assess the limitations of traditional mortality metrics and explore emerging approaches to risk assessment and prediction, including the integration of advanced statistical methods and machine learning techniques. We also address the ethical considerations surrounding mortality prediction and end-of-life care. Finally, we discuss future research directions aimed at improving our understanding of mortality and developing more effective strategies to promote longevity and quality of life.
1. Introduction: Defining and Contextualizing Mortality
Mortality, defined as the state of being subject to death, is a fundamental aspect of the human experience. It is both a biological certainty and a societal concern, influencing public health policy, healthcare resource allocation, and individual life choices. Understanding mortality requires a nuanced approach that considers its diverse dimensions and the interplay of factors that contribute to its occurrence.
Historically, mortality rates have served as key indicators of population health and well-being. High mortality rates were once commonplace, particularly among infants and children, reflecting the prevalence of infectious diseases, malnutrition, and inadequate sanitation. Over the past century, significant advancements in medicine, public health, and living standards have led to a dramatic decline in mortality rates in many parts of the world. However, these gains have not been universally shared, and disparities in mortality persist across countries, regions, and socioeconomic groups.
The study of mortality involves a wide range of disciplines, including epidemiology, demography, biostatistics, and gerontology. Epidemiologists investigate the causes and patterns of mortality, identifying risk factors and developing strategies for prevention. Demographers analyze mortality trends and their impact on population structure and growth. Biostatisticians develop statistical models to analyze mortality data and predict future trends. Gerontologists focus on the aging process and the factors that contribute to mortality in older adults.
This research report aims to provide a comprehensive overview of mortality, examining its historical context, current trends, and future challenges. We will explore the various factors that influence mortality, critically assess the limitations of current measurement approaches, and discuss emerging strategies for risk assessment and prevention. Our goal is to provide a nuanced understanding of mortality that informs public health policy, healthcare practice, and individual decision-making.
2. Historical Trends and Geographical Variations in Mortality
The trajectory of mortality rates throughout history provides valuable insights into the evolving determinants of human health. In pre-industrial societies, mortality was primarily driven by infectious diseases, famine, and warfare. Infant and child mortality rates were exceedingly high, with a significant proportion of children dying before reaching adulthood. The advent of sanitation, improved nutrition, and rudimentary medical interventions gradually led to a decline in mortality rates, albeit at a slow pace.
The Industrial Revolution marked a turning point, bringing about both challenges and opportunities for population health. While urbanization and industrial pollution initially led to increased mortality in some areas, advances in public health infrastructure, such as clean water supplies and sewage systems, eventually contributed to a significant reduction in infectious diseases. The development of vaccines and antibiotics in the 20th century further accelerated the decline in mortality rates, leading to a dramatic increase in life expectancy in many parts of the world.
However, the decline in mortality has not been uniform across the globe. Significant disparities persist between developed and developing countries. In high-income countries, chronic diseases, such as heart disease, cancer, and diabetes, have become the leading causes of death. In low-income countries, infectious diseases, malnutrition, and maternal mortality remain major challenges. Geographical variations in mortality also reflect differences in access to healthcare, socioeconomic conditions, and environmental factors.
For example, Sub-Saharan Africa continues to experience higher mortality rates compared to other regions, largely due to the prevalence of HIV/AIDS, malaria, and other infectious diseases. Conflict and political instability also contribute to increased mortality in many African countries. In contrast, countries in Western Europe and North America have some of the lowest mortality rates in the world, reflecting their advanced healthcare systems, high living standards, and effective public health programs.
Understanding these historical trends and geographical variations in mortality is crucial for identifying the underlying drivers of population health and developing targeted interventions to reduce disparities and improve health outcomes globally.
3. Key Determinants of Mortality: A Multifactorial Perspective
Mortality is not solely determined by biological factors but is rather a complex interplay of numerous determinants spanning biological, social, economic, and environmental domains. Understanding these multifaceted influences is crucial for devising effective strategies to reduce mortality and promote healthier lives.
-
Biological Factors: Age is the most significant predictor of mortality, with mortality rates increasing exponentially with age. Genetic predispositions also play a role, influencing susceptibility to certain diseases. Sex differences in mortality have been observed, with women generally having longer life expectancies than men, although this gap is narrowing in some populations. The presence of chronic diseases, such as cardiovascular disease, cancer, and diabetes, significantly increases the risk of mortality.
-
Socioeconomic Factors: Socioeconomic status (SES) is strongly associated with mortality. Individuals with lower SES tend to experience higher mortality rates due to factors such as limited access to healthcare, poor nutrition, exposure to environmental hazards, and increased stress. Education level is also a key determinant of mortality, with higher education levels being associated with lower mortality rates. Income inequality within a society can also contribute to increased mortality, as it can lead to social unrest, limited access to resources, and chronic stress.
-
Lifestyle Factors: Lifestyle choices play a significant role in determining mortality risk. Smoking, excessive alcohol consumption, unhealthy diets, and physical inactivity are all associated with increased mortality. Conversely, healthy lifestyle choices, such as regular exercise, a balanced diet, and avoiding smoking and excessive alcohol consumption, can significantly reduce mortality risk.
-
Environmental Factors: Environmental factors, such as air and water pollution, exposure to toxic substances, and climate change, can significantly impact mortality rates. Air pollution, for example, is a major contributor to respiratory diseases and cardiovascular disease, leading to increased mortality. Climate change can exacerbate existing health problems and contribute to new ones, such as heatstroke and infectious diseases.
-
Healthcare Access and Quality: Access to quality healthcare is a critical determinant of mortality. Individuals who have limited access to healthcare, or who receive poor quality care, are at increased risk of mortality. Factors such as insurance coverage, availability of healthcare providers, and cultural barriers can all affect access to healthcare. The quality of healthcare also plays a role, with individuals who receive high-quality care being more likely to survive and have better health outcomes.
-
Social Support and Networks: Social support and strong social networks can have a protective effect against mortality. Individuals who have strong social connections are more likely to have better mental and physical health, and are more likely to engage in healthy behaviors. Social isolation, on the other hand, can increase the risk of mortality.
Understanding the complex interplay of these factors is essential for developing effective interventions to reduce mortality and improve population health. Public health policies should address not only individual behaviors but also the broader social, economic, and environmental determinants of health.
4. Limitations of Traditional Mortality Metrics and Emerging Approaches
While traditional mortality metrics, such as crude mortality rate and age-specific mortality rate, provide valuable insights into population health, they have limitations. Crude mortality rate, defined as the number of deaths per 1,000 population, is a simple measure but does not account for age structure or other confounding factors. Age-specific mortality rates provide a more nuanced picture but do not capture the complexities of competing causes of death or the impact of multiple risk factors.
One major limitation of traditional mortality metrics is their reliance on aggregate data, which can mask disparities within populations. For example, an overall mortality rate may not reveal significant differences in mortality between different socioeconomic groups or racial/ethnic groups. Another limitation is their inability to capture the impact of chronic diseases and the burden of disability. Mortality rates only reflect the occurrence of death, not the quality of life or the years lived with disability.
To address these limitations, researchers have developed emerging approaches to mortality measurement that incorporate more complex statistical methods and data sources.
-
Life Expectancy Adjusted for Quality of Life (QALYs): QALYs combine information about both the length and quality of life. A QALY represents one year of perfect health. By adjusting life expectancy for the quality of life, QALYs provide a more comprehensive measure of health outcomes.
-
Disability-Adjusted Life Years (DALYs): DALYs measure the total burden of disease, combining years of life lost due to premature mortality with years lived with disability. DALYs provide a more comprehensive picture of the impact of disease on population health than mortality rates alone.
-
Competing Risks Analysis: Competing risks analysis is a statistical method that accounts for the fact that individuals can die from multiple causes. This method allows researchers to estimate the probability of dying from a specific cause, taking into account the risk of dying from other causes. This is important because traditional methods can overestimate the probability of dying from a specific cause if they do not account for competing risks.
-
Machine Learning Models: Machine learning models are increasingly being used to predict mortality risk. These models can incorporate a wide range of risk factors and can identify complex patterns that may not be apparent using traditional statistical methods. Machine learning models have the potential to improve risk stratification and personalize healthcare interventions. However, they must be used carefully to avoid bias and ensure fairness. As noted in the prompt, such models have faced failures. The challenge often lies in the complex interplay of factors influencing mortality, data limitations, and the inherent unpredictability of individual outcomes. Moreover, ethical considerations surrounding the use of such models for decision-making are paramount.
-
Longitudinal Data Analysis: Longitudinal studies that follow individuals over time provide valuable data for understanding the trajectory of health and mortality. These studies allow researchers to identify risk factors that predict mortality and to examine the impact of interventions on health outcomes.
These emerging approaches to mortality measurement offer a more nuanced and comprehensive understanding of population health. By incorporating information about quality of life, disability, and competing causes of death, these approaches can provide valuable insights for public health policy and healthcare practice.
5. Ethical Considerations in Mortality Prediction and End-of-Life Care
The ability to predict mortality raises significant ethical considerations, particularly in the context of end-of-life care. While accurate mortality prediction can inform medical decision-making and resource allocation, it also raises concerns about patient autonomy, privacy, and potential for discrimination.
One key ethical challenge is ensuring that mortality predictions are used in a way that respects patient autonomy and values. Patients have the right to make informed decisions about their healthcare, and mortality predictions should not be used to pressure patients into making choices that they do not want to make. It is also important to ensure that patients understand the limitations of mortality predictions and that they are not used to create a sense of hopelessness or despair.
Another ethical concern is protecting patient privacy. Mortality predictions are based on sensitive patient data, and it is important to ensure that this data is protected from unauthorized access and use. Data security measures should be in place to prevent data breaches, and patients should be informed about how their data will be used.
There is also a risk of discrimination based on mortality predictions. If mortality predictions are used to allocate healthcare resources, there is a risk that individuals with higher predicted mortality rates will be denied access to care. This could lead to inequities in healthcare access and outcomes.
In addition to these ethical concerns, there are also practical challenges in implementing mortality prediction models. One challenge is ensuring that the models are accurate and reliable. Mortality predictions should be based on sound scientific evidence, and the models should be validated to ensure that they perform well in different populations. Another challenge is integrating mortality prediction models into clinical practice. Healthcare providers need to be trained on how to use the models effectively and how to communicate the results to patients.
Ethical considerations surrounding end-of-life care are equally crucial. Palliative care aims to improve the quality of life for patients and their families facing life-limiting illnesses. Ethical principles such as beneficence (acting in the patient’s best interest), non-maleficence (avoiding harm), autonomy (respecting the patient’s right to self-determination), and justice (ensuring fair and equitable access to care) are paramount in guiding end-of-life care decisions. Advance care planning, including the creation of advance directives, allows patients to express their wishes regarding medical treatment in the event that they are unable to do so themselves.
Addressing these ethical challenges requires a collaborative effort involving healthcare providers, ethicists, policymakers, and the public. Open and transparent discussions about the ethical implications of mortality prediction and end-of-life care are essential for ensuring that these technologies are used in a way that benefits patients and society as a whole.
6. Future Research Directions and Conclusion
The study of mortality continues to evolve, driven by advancements in technology, changing demographics, and emerging health challenges. Future research directions should focus on several key areas:
-
Improving the Accuracy and Reliability of Mortality Prediction Models: Further research is needed to develop more accurate and reliable mortality prediction models. This includes incorporating new data sources, such as genomic data and social determinants of health data, and developing more sophisticated statistical methods.
-
Understanding the Biological Mechanisms of Aging and Mortality: A deeper understanding of the biological mechanisms of aging and mortality is needed to develop interventions that can slow down the aging process and extend lifespan. This includes research on telomeres, oxidative stress, and other biological processes that contribute to aging.
-
Addressing Health Disparities in Mortality: Targeted interventions are needed to address health disparities in mortality. This includes addressing socioeconomic factors, improving access to healthcare, and promoting healthy behaviors in underserved populations.
-
Developing Personalized Interventions to Reduce Mortality Risk: Personalized interventions that are tailored to an individual’s specific risk factors and needs are needed to reduce mortality risk. This includes using genetic information, lifestyle factors, and other data to develop individualized prevention plans.
-
Exploring the Impact of Emerging Technologies on Mortality: Emerging technologies, such as artificial intelligence and gene editing, have the potential to significantly impact mortality. Research is needed to explore the ethical and societal implications of these technologies.
-
Expanding the Scope of Mortality Measurement: As highlighted earlier, moving beyond traditional metrics is crucial. Research should prioritize the refinement and validation of QALYs, DALYs, and other comprehensive measures that capture the holistic impact of health conditions.
In conclusion, mortality is a complex and multifaceted phenomenon that is influenced by a wide range of factors. Understanding these factors is essential for developing effective interventions to reduce mortality and improve population health. By embracing a multidisciplinary approach and prioritizing ethical considerations, we can strive to create a future where everyone has the opportunity to live a long and healthy life.
References
- Christakis, N. A. (2000). Death Foretold: Prophecy and Prognosis in Medical Care. University of Chicago Press.
- Crimmins, E. M. (2015). Lifespan and healthspan: Past, present, and future. The Gerontologist, 55(5), 750-761.
- Goldman, D., & Cutler, D. M. (2001). Socioeconomic gradients in health. Annual Review of Public Health, 22(1), 349-372.
- Hummer, R. A., & Hernandez, B. (2013). The effect of educational attainment on adult mortality in the United States. Population Bulletin, 68(1), 1-16.
- Murray, C. J. L., & Lopez, A. D. (1996). The global burden of disease: A comprehensive assessment of mortality and disability from diseases, injuries, and risk factors in 1990 and projected to 2020. Harvard University Press.
- Olshansky, S. J., Passaro, D. J., Hershow, R. C., Layden, J., Carnes, B. A., Brody, J., Hayflick, L., & Butler, R. N. (2005). A potential decline in life expectancy in the United States in the 21st century. New England Journal of Medicine, 352(11), 1138-1145.
- Sen, A. (1999). Development as freedom. Oxford University Press.
- World Health Organization. (2023). World Health Statistics 2023. Geneva: World Health Organization.
- Esteban-Gil, A., et al. (2023). Failure of machine learning to predict in-hospital mortality for low-risk patients with COVID-19 pneumonia. The Lancet Digital Health, 5(7), e410-e412.
The discussion on ethical considerations is critical, particularly concerning patient autonomy and data privacy when predicting mortality. How can we best balance the benefits of these predictions with the need to protect vulnerable individuals from potential bias or coercion in end-of-life decisions?
That’s a really important question! Ensuring patient autonomy is key. Perhaps incorporating diverse ethical perspectives in the development and deployment of these predictive models could help mitigate potential biases. Openly discussing the limitations of predictions with patients is also crucial to prevent coercion. I would be interested in hearing what others think about this.
Editor: MedTechNews.Uk
Thank you to our Sponsor Esdebe