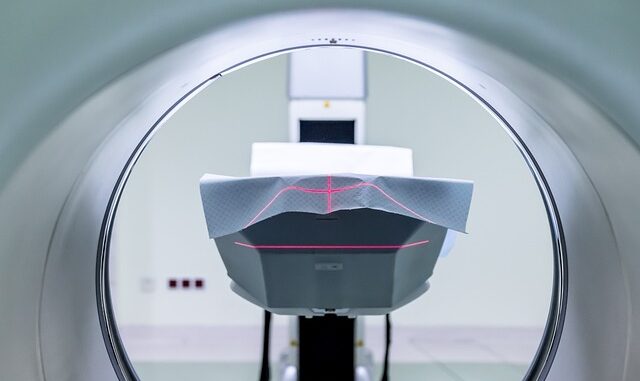
Advanced MRI Techniques for Quantitative Neurological Assessment: From Biomarker Discovery to Personalized Treatment Monitoring
Abstract
Magnetic Resonance Imaging (MRI) has revolutionized neurological diagnosis and treatment monitoring. Beyond conventional structural imaging, advanced MRI techniques offer quantitative insights into brain tissue microstructure, function, and metabolism. This review explores the evolving landscape of advanced MRI, covering diffusion-weighted imaging (DWI) and its variants, perfusion imaging, functional MRI (fMRI), magnetic resonance spectroscopy (MRS), and quantitative susceptibility mapping (QSM). We discuss the underlying principles, acquisition methods, and clinical applications of each technique, with a particular emphasis on their utility in identifying biomarkers for neurological diseases and tracking treatment response. We also address the challenges associated with data acquisition and analysis, including artifacts, standardization, and the need for robust processing pipelines. Finally, we explore the potential of combining multi-modal MRI data with artificial intelligence (AI) for personalized treatment strategies.
1. Introduction
The advent of Magnetic Resonance Imaging (MRI) has provided clinicians and researchers with an unprecedented non-invasive window into the human brain. Initially focused on structural imaging, MRI has evolved into a multifaceted tool capable of probing tissue microstructure, function, and metabolism. The increasing demand for objective and quantitative measures in clinical neuroscience has fueled the development and application of advanced MRI techniques. These techniques offer the potential to identify early biomarkers of disease, monitor treatment response, and ultimately personalize treatment strategies. This review aims to provide a comprehensive overview of advanced MRI techniques, focusing on their quantitative capabilities and their application in neurological assessment. We will discuss the theoretical underpinnings, acquisition and processing methods, and clinical utility of each technique, while also highlighting the challenges and future directions in this rapidly evolving field.
2. Diffusion-Weighted Imaging (DWI) and Advanced Diffusion Models
Diffusion-weighted imaging (DWI) is sensitive to the Brownian motion of water molecules, providing valuable information about tissue microstructure. In white matter, water diffusion is anisotropic due to the presence of myelin sheaths and axonal membranes, allowing for the assessment of white matter integrity. The most commonly used DWI metric is the apparent diffusion coefficient (ADC), which quantifies the magnitude of water diffusion. However, ADC is a simplified measure and does not capture the complexity of diffusion in heterogeneous tissues. Diffusion Tensor Imaging (DTI) is a more advanced technique that models water diffusion as a tensor, allowing for the calculation of fractional anisotropy (FA), a measure of white matter tract organization. DTI has been widely used to study white matter abnormalities in various neurological disorders, including multiple sclerosis (MS), stroke, and traumatic brain injury [1].
However, DTI has limitations in regions with complex fiber architecture, such as fiber crossings and fanning. More advanced diffusion models, such as diffusion kurtosis imaging (DKI) and neurite orientation dispersion and density imaging (NODDI), have been developed to address these limitations. DKI quantifies the non-Gaussianity of water diffusion, providing additional information about tissue microstructure. NODDI models brain tissue as a combination of neurites (axons and dendrites), extracellular space, and cerebrospinal fluid, allowing for the estimation of neurite density and orientation dispersion [2]. These advanced diffusion models have shown promise in detecting subtle microstructural changes in neurological diseases and may be more sensitive to treatment effects than DTI. The clinical applicability of advanced diffusion models is growing, but requires careful attention to acquisition parameters, processing methods, and potential confounds.
3. Perfusion Imaging
Perfusion imaging assesses cerebral blood flow (CBF) and blood volume, providing information about tissue viability and vascular function. Dynamic Susceptibility Contrast (DSC)-MRI is a commonly used technique that involves injecting a contrast agent and monitoring its passage through the brain vasculature. DSC-MRI allows for the calculation of CBF, cerebral blood volume (CBV), and mean transit time (MTT). Arterial Spin Labeling (ASL) is a non-contrast-enhanced technique that uses magnetically labeled arterial blood water as an endogenous tracer. ASL offers several advantages over DSC-MRI, including the absence of contrast agent administration and the ability to acquire repeated measurements. However, ASL has lower signal-to-noise ratio (SNR) than DSC-MRI.
Perfusion imaging is valuable in the diagnosis and management of stroke, brain tumors, and neurodegenerative diseases. In stroke, perfusion imaging can identify the ischemic penumbra, the region of potentially salvageable tissue surrounding the infarct core. In brain tumors, perfusion imaging can assess tumor angiogenesis and predict treatment response. In neurodegenerative diseases, perfusion imaging can detect regional CBF changes that may precede structural atrophy [3]. The reproducibility and accuracy of perfusion measurements are crucial for clinical applications. Standardization of acquisition protocols, advanced post-processing techniques, and careful consideration of physiological factors, such as arterial transit time, are essential for obtaining reliable and clinically relevant perfusion data.
4. Functional MRI (fMRI)
Functional MRI (fMRI) measures brain activity by detecting changes in blood oxygenation levels, a phenomenon known as the blood oxygenation level-dependent (BOLD) effect. During neuronal activity, there is an increase in local CBF, which leads to an increase in the ratio of oxygenated to deoxygenated hemoglobin. This change in blood oxygenation alters the local magnetic field, which can be detected by MRI. fMRI can be used to study a wide range of cognitive functions, including motor control, language processing, and memory. Task-based fMRI involves performing specific tasks during scanning, while resting-state fMRI (rs-fMRI) measures brain activity in the absence of any explicit task [4].
rs-fMRI has gained increasing attention in recent years due to its ability to assess intrinsic brain connectivity. rs-fMRI data can be used to identify networks of brain regions that are functionally connected, known as resting-state networks (RSNs). Alterations in RSN connectivity have been observed in various neurological and psychiatric disorders. fMRI is a powerful tool for studying brain function, but it is also susceptible to various artifacts, including head motion and physiological noise. Sophisticated pre-processing techniques are necessary to minimize these artifacts and ensure the reliability of fMRI results. Furthermore, the interpretation of fMRI data requires careful consideration of the underlying neural mechanisms and the limitations of the BOLD signal. The ongoing debate about the replicability of fMRI studies underscores the need for rigorous experimental design, statistical analysis, and data sharing practices.
5. Magnetic Resonance Spectroscopy (MRS)
Magnetic Resonance Spectroscopy (MRS) is a non-invasive technique that allows for the quantification of specific brain metabolites. MRS can detect various metabolites, including N-acetylaspartate (NAA), a marker of neuronal integrity; creatine (Cr), a marker of energy metabolism; choline (Cho), a marker of cell membrane turnover; and lactate (Lac), a marker of anaerobic metabolism. By measuring the concentrations of these metabolites, MRS can provide insights into brain metabolism and cellular function [5].
MRS is valuable in the diagnosis and management of various neurological disorders, including brain tumors, epilepsy, and metabolic disorders. In brain tumors, MRS can differentiate between tumor types and assess treatment response. In epilepsy, MRS can identify metabolic abnormalities in the epileptogenic zone. In metabolic disorders, MRS can detect specific metabolite abnormalities that are characteristic of the disease. The spectral resolution and SNR of MRS data are critical for accurate metabolite quantification. Advanced spectral fitting algorithms and optimal acquisition parameters are essential for obtaining reliable MRS results. Furthermore, the interpretation of MRS data requires careful consideration of the physiological factors that can influence metabolite concentrations.
6. Quantitative Susceptibility Mapping (QSM)
Quantitative Susceptibility Mapping (QSM) is a relatively new MRI technique that quantifies the magnetic susceptibility of brain tissue. Magnetic susceptibility is a physical property of matter that describes how easily it becomes magnetized in an applied magnetic field. QSM is sensitive to the presence of iron, myelin, and calcium, making it valuable in the study of neurodegenerative diseases, such as Parkinson’s disease and Alzheimer’s disease [6].
In Parkinson’s disease, QSM can detect increased iron levels in the substantia nigra, a brain region that is affected by the disease. In Alzheimer’s disease, QSM can detect increased iron levels in the hippocampus, a brain region that is involved in memory. QSM data can be used to create susceptibility maps, which provide a visual representation of the distribution of magnetic susceptibility in the brain. QSM requires sophisticated post-processing techniques to correct for artifacts and accurately quantify magnetic susceptibility. The development of robust and standardized QSM processing pipelines is essential for the widespread adoption of this technique in clinical practice. Moreover, the integration of QSM with other MRI modalities, such as DWI and fMRI, holds promise for providing a more comprehensive understanding of brain structure, function, and metabolism.
7. Multi-Modal MRI and Integration with Artificial Intelligence
Combining multiple MRI techniques can provide a more comprehensive understanding of brain structure, function, and metabolism than any single technique alone. For example, combining DWI and fMRI can provide insights into the relationship between white matter integrity and brain activity. Combining MRS and QSM can provide insights into the relationship between brain metabolism and iron levels. The integration of multi-modal MRI data requires sophisticated data fusion techniques [7].
Artificial intelligence (AI) is playing an increasingly important role in the analysis of multi-modal MRI data. AI algorithms can be used to automatically segment brain structures, classify diseases, and predict treatment response. AI can also be used to identify complex patterns in multi-modal MRI data that would be difficult or impossible to detect manually. For example, AI can be trained to predict the progression of Alzheimer’s disease based on a combination of structural MRI, functional MRI, and PET imaging data. The development of robust and reliable AI algorithms for MRI analysis is essential for the widespread adoption of these techniques in clinical practice. However, the use of AI in healthcare also raises ethical considerations, such as data privacy, algorithmic bias, and the potential for over-reliance on AI systems.
8. Challenges and Future Directions
Despite the significant advances in advanced MRI techniques, several challenges remain. These include:
- Artifacts: MRI data is susceptible to various artifacts, including head motion, physiological noise, and susceptibility artifacts. These artifacts can degrade image quality and affect the accuracy of quantitative measurements. Developing robust artifact correction techniques is essential for obtaining reliable MRI data. Specifically, motion correction algorithms, prospective motion correction techniques, and advanced shimming methods are crucial for mitigating the impact of these artifacts.
- Standardization: There is a lack of standardization in MRI acquisition protocols and post-processing techniques. This makes it difficult to compare results across different studies and clinical sites. Developing standardized protocols and techniques is essential for ensuring the reproducibility and comparability of MRI data. The development of phantoms that can be used to calibrate MRI scanners and standardize imaging parameters is also essential.
- Data analysis: The analysis of advanced MRI data can be complex and time-consuming. Developing automated and user-friendly data analysis tools is essential for making these techniques more accessible to clinicians and researchers.
- Clinical validation: Many advanced MRI techniques have not been fully validated in clinical trials. Conducting large-scale clinical trials is essential for demonstrating the clinical utility of these techniques and translating them into clinical practice. This includes demonstrating clinical efficacy, cost-effectiveness, and impact on patient outcomes.
The future of advanced MRI techniques is bright. With continued advances in hardware, software, and AI, these techniques will play an increasingly important role in the diagnosis, treatment, and monitoring of neurological diseases. The development of new contrast agents, higher field strength MRI scanners, and more sophisticated AI algorithms will further enhance the capabilities of advanced MRI. Furthermore, the integration of MRI with other imaging modalities, such as PET and CT, will provide a more comprehensive understanding of brain structure, function, and metabolism. Ultimately, the goal is to use advanced MRI to personalize treatment strategies and improve patient outcomes.
9. Conclusion
Advanced MRI techniques have transformed neurological assessment by providing quantitative measures of brain microstructure, function, and metabolism. Diffusion-weighted imaging, perfusion imaging, functional MRI, magnetic resonance spectroscopy, and quantitative susceptibility mapping offer complementary information about brain tissue properties and can be used to identify biomarkers for neurological diseases and monitor treatment response. The integration of multi-modal MRI data with artificial intelligence holds promise for personalized treatment strategies. Despite the challenges associated with data acquisition and analysis, the future of advanced MRI in neurology is promising, with continued advances in hardware, software, and AI driving further innovation and clinical translation.
References
[1] Alexander, A. L., Lee, J. E., Lazar, M., & Field, A. S. (2007). Diffusion tensor imaging of the brain. Nature Protocols, 2(12), 3227-3241.
[2] Zhang, H., Schneider, T., Wheeler-Kingshott, C. A., & Alexander, D. C. (2012). NODDI: practical in vivo neurite orientation dispersion and density imaging of the human brain. NeuroImage, 61(4), 1000-1016.
[3] Detre, J. A., Wang, J., Rao, H., & Wang, P. (2009). Arterial spin labeling MRI for clinical applications. Journal of Magnetic Resonance Imaging, 30(6), 1262-1275.
[4] Biswal, B. B., Yetkin, F. Z., Haughton, V. M., & Hyde, J. S. (1995). Functional connectivity in the motor cortex of resting human brain using echo-planar MRI. Magnetic Resonance in Medicine, 34(4), 537-541.
[5] Govindaraju, V., Young, K., Maudsley, A. A., & Sujatha, J. (2000). Proton NMR spectroscopy for in vivo metabolic characterization of human brain tumors. NMR in Biomedicine, 13(3), 129-149.
[6] Wang, Y., & Liu, T. (2015). Quantitative susceptibility mapping (QSM): decoding human brain in vivo magnetic property using MRI signal. Magnetic Resonance in Medicine, 73(1), 82-101.
[7] Calhoun, V. D., & Sui, J. (2016). Multimodal fusion of brain imaging data: a critical review and future directions. Human Brain Mapping, 37(11), 4233-4252.
The potential of AI to analyze multi-modal MRI data for early disease detection is exciting. How might we standardize data acquisition across different MRI platforms to ensure AI algorithms can be effectively trained and deployed for widespread clinical use?
That’s a great point about standardization! Harmonizing acquisition protocols across platforms is key. Perhaps developing open-source, validated phantoms and standardized pulse sequence repositories could help create more robust and generalizable AI models for widespread clinical use.
Editor: MedTechNews.Uk
Thank you to our Sponsor Esdebe