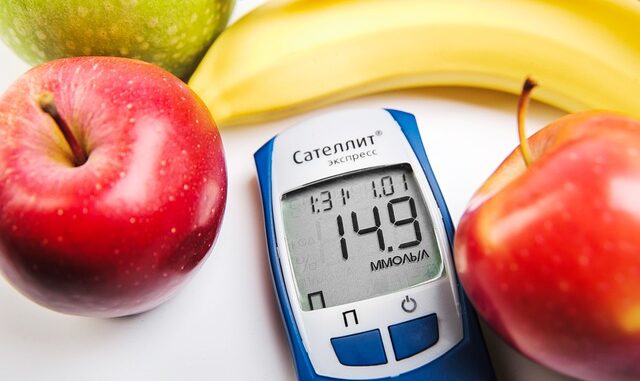
Abstract
Artificial pancreas (AP) systems, also known as automated insulin delivery (AID) systems, represent a significant advancement in the management of type 1 diabetes (T1D) and, increasingly, type 2 diabetes (T2D). These systems integrate continuous glucose monitoring (CGM) with insulin pumps, utilizing sophisticated algorithms to automatically adjust insulin delivery based on real-time glucose levels. This report provides a comprehensive overview of AP systems, encompassing their historical development, diverse configurations (including hybrid closed-loop, fully closed-loop, and bihormonal systems), underlying control algorithms, and user experience considerations. We delve into the complexities of advanced control strategies such as model predictive control (MPC), fuzzy logic, and artificial intelligence (AI)-driven approaches, examining their strengths and limitations in the context of glycemic variability and individualized patient needs. Furthermore, we explore the clinical outcomes associated with AP system use, including improvements in glycemic control, reductions in hypoglycemia, and enhanced quality of life. Finally, we discuss the challenges and future directions of AP technology, focusing on integration with other technologies (e.g., novel sensors, glucagon delivery), personalization strategies, and the potential for preventative applications in at-risk populations.
Many thanks to our sponsor Esdebe who helped us prepare this research report.
1. Introduction
Type 1 diabetes (T1D) is an autoimmune disease characterized by the destruction of insulin-producing beta cells in the pancreas, leading to absolute insulin deficiency. Individuals with T1D require exogenous insulin administration to regulate blood glucose levels and prevent life-threatening complications such as diabetic ketoacidosis (DKA) and severe hypoglycemia. While conventional insulin therapy (multiple daily injections or continuous subcutaneous insulin infusion [CSII]) can be effective, it often requires significant patient effort and vigilance in monitoring blood glucose and adjusting insulin doses. This reliance on patient adherence and accurate bolus calculations can lead to substantial glycemic variability, increasing the risk of both acute and chronic complications.
Artificial pancreas (AP) systems have emerged as a transformative technology aimed at automating insulin delivery and minimizing the burden of diabetes management. AP systems, also referred to as automated insulin delivery (AID) systems, consist of three primary components: a continuous glucose monitor (CGM) that measures glucose levels in real-time, an insulin pump that delivers insulin subcutaneously, and a control algorithm that automatically adjusts insulin delivery based on CGM data. The goal of an AP system is to mimic the function of a healthy pancreas by maintaining glucose levels within a target range, thereby reducing the risk of hyperglycemia and hypoglycemia. This paradigm shift moves from open-loop to closed-loop glucose control, offering the potential for improved glycemic outcomes and enhanced quality of life for individuals with diabetes.
This report provides a comprehensive overview of AP systems, covering their historical evolution, technological advancements, clinical benefits, and future directions. We will explore the different types of AP systems, discuss the control algorithms that govern insulin delivery, examine the user experience and adherence considerations, and assess the clinical outcomes associated with AP system use. Furthermore, we will delve into the challenges and future opportunities in this rapidly evolving field, including the integration of new technologies, the development of personalized control strategies, and the potential for AP systems to prevent or delay the onset of diabetes complications.
Many thanks to our sponsor Esdebe who helped us prepare this research report.
2. Evolution of Artificial Pancreas Systems
The development of AP systems has been a long and iterative process, spanning several decades of research and technological innovation. Early attempts at closed-loop insulin delivery date back to the 1970s, with the introduction of intravenous glucose sensors and insulin infusion systems for use in hospital settings. These early systems were bulky, complex, and primarily intended for short-term glucose control during surgery or critical illness. However, they laid the foundation for the development of more portable and user-friendly AP systems.
The first generation of subcutaneous AP systems emerged in the late 1990s and early 2000s. These systems consisted of a CGM and an insulin pump connected to a computer, which implemented a control algorithm to adjust insulin delivery. However, these systems were still relatively cumbersome and required frequent calibration of the CGM. Furthermore, the control algorithms were often limited in their ability to handle the complexities of glucose dynamics, leading to suboptimal glycemic control.
A significant breakthrough came with the development of hybrid closed-loop (HCL) systems. HCL systems automate basal insulin delivery but require the user to manually bolus for meals. This approach simplifies the control problem and reduces the risk of hypoglycemia while still providing substantial improvements in glycemic control compared to conventional insulin therapy. Several HCL systems have been approved by regulatory agencies and are now commercially available.
The next generation of AP systems aims to achieve fully closed-loop control, eliminating the need for manual bolus administration. These systems utilize advanced control algorithms that can automatically adjust both basal and bolus insulin delivery based on real-time glucose levels and predicted glucose trends. While fully closed-loop systems are still under development, preliminary clinical studies have shown promising results.
More recently, research has focused on the development of bihormonal AP systems, which incorporate both insulin and glucagon delivery. Glucagon is a hormone that raises blood glucose levels, and its inclusion in an AP system allows for more aggressive correction of hypoglycemia. Bihormonal systems have the potential to further improve glycemic control and reduce the risk of hypoglycemia, particularly in individuals with impaired awareness of hypoglycemia.
Many thanks to our sponsor Esdebe who helped us prepare this research report.
3. Types of Artificial Pancreas Systems
AP systems can be broadly classified into several categories based on their level of automation, control algorithm, and hormonal components. The following are the main types of AP systems:
3.1 Hybrid Closed-Loop (HCL) Systems:
HCL systems are the most widely available type of AP system. They automate basal insulin delivery based on CGM data but require the user to manually bolus for meals. The control algorithm in an HCL system typically adjusts basal insulin delivery every few minutes to maintain glucose levels within a target range. HCL systems offer a balance between automation and user control, providing significant improvements in glycemic control while still allowing the user to manage mealtime insulin delivery. An example is Medtronic’s MiniMed 780G system.
3.2 Fully Closed-Loop (FCL) Systems:
FCL systems aim to automate both basal and bolus insulin delivery. These systems utilize advanced control algorithms that can predict glucose trends and adjust insulin delivery accordingly. FCL systems are more complex than HCL systems but have the potential to further reduce the burden of diabetes management and improve glycemic outcomes. Several FCL systems are currently under development, and some have shown promising results in clinical trials. These systems often utilize advanced machine learning techniques to predict glucose excursions and personalize insulin delivery. An example is Tidepool Loop.
3.3 Bihormonal Systems:
Bihormonal AP systems incorporate both insulin and glucagon delivery. The addition of glucagon allows for more aggressive correction of hypoglycemia and can potentially improve glycemic control and reduce the risk of hypoglycemia. Bihormonal systems are particularly beneficial for individuals with impaired awareness of hypoglycemia. The development of stable and user-friendly glucagon formulations has been a key factor in the advancement of bihormonal AP systems.
3.4 Threshold Suspend Systems:
These are simpler systems that suspend insulin delivery when glucose levels fall below a pre-set threshold. While not a full closed-loop system, they can significantly reduce the frequency and severity of hypoglycemic episodes. These systems are often a stepping stone for patients to transition to more complex AP systems.
3.5 Advanced Hybrid Closed-Loop (AHCL) Systems:
These systems represent an evolution of HCL technology, incorporating features such as automated correction boluses and meal detection algorithms to further reduce user burden and improve glycemic control. They often learn the user’s patterns and adjust insulin delivery accordingly, making them more personalized and effective.
Many thanks to our sponsor Esdebe who helped us prepare this research report.
4. Components of Artificial Pancreas Systems
AP systems consist of several key components that work together to automate insulin delivery. These components include:
4.1 Continuous Glucose Monitor (CGM):
The CGM is a small sensor that is inserted under the skin and measures glucose levels in the interstitial fluid. CGMs provide real-time glucose readings every few minutes, allowing the AP system to track glucose trends and adjust insulin delivery accordingly. The accuracy and reliability of the CGM are critical for the effective functioning of the AP system. Recent advancements in CGM technology have led to improved accuracy, reduced lag time, and longer sensor life. Examples include Dexcom G7 and Abbott FreeStyle Libre 3.
4.2 Insulin Pump:
The insulin pump is a small, battery-powered device that delivers insulin subcutaneously. Insulin pumps can deliver both basal and bolus insulin, and they can be programmed to deliver different insulin rates at different times of the day. The insulin pump is controlled by the AP system’s control algorithm, which adjusts insulin delivery based on CGM data. Insulin pumps are becoming increasingly sophisticated, with features such as remote control, Bluetooth connectivity, and integration with smartphone apps.
4.3 Control Algorithm:
The control algorithm is the brain of the AP system. It receives data from the CGM, processes the data, and calculates the appropriate insulin delivery rate. The control algorithm is responsible for maintaining glucose levels within a target range and preventing hyperglycemia and hypoglycemia. Control algorithms can be based on a variety of mathematical and computational techniques, including proportional-integral-derivative (PID) control, model predictive control (MPC), fuzzy logic, and artificial intelligence (AI). The choice of control algorithm depends on the specific requirements of the AP system and the characteristics of the individual user. The algorithm must be robust, reliable, and adaptable to changing conditions. MPC algorithms are favored for their ability to predict future glucose levels and adjust insulin delivery proactively, but they require accurate models of glucose dynamics.
4.4 Communication System:
The communication system allows the CGM, insulin pump, and control algorithm to communicate with each other. This communication can be achieved through wired or wireless connections. Wireless communication is becoming increasingly common, as it allows for greater flexibility and convenience. The communication system must be reliable and secure to ensure the proper functioning of the AP system.
4.5 User Interface:
The user interface is the means by which the user interacts with the AP system. The user interface can be a dedicated device, such as a smartphone or tablet, or it can be integrated into the insulin pump or CGM. The user interface allows the user to view glucose data, adjust settings, and receive alerts. The user interface should be intuitive, user-friendly, and accessible to individuals with varying levels of technical expertise.
Many thanks to our sponsor Esdebe who helped us prepare this research report.
5. Control Algorithms for Artificial Pancreas Systems
The control algorithm is a critical component of an AP system, responsible for determining the appropriate insulin delivery rate based on real-time glucose data and predicted glucose trends. Several types of control algorithms have been developed and implemented in AP systems, each with its own strengths and limitations. These algorithms can be broadly classified into the following categories:
5.1 Proportional-Integral-Derivative (PID) Control:
PID control is a classical control algorithm that is widely used in industrial applications. PID controllers adjust insulin delivery based on three parameters: the proportional gain (Kp), the integral gain (Ki), and the derivative gain (Kd). The proportional gain determines the immediate response to changes in glucose levels, the integral gain corrects for long-term errors, and the derivative gain anticipates future changes in glucose levels. PID controllers are relatively simple to implement but may not be optimal for the complex dynamics of glucose metabolism. They often require careful tuning to achieve satisfactory performance.
5.2 Model Predictive Control (MPC):
MPC is an advanced control algorithm that uses a mathematical model of the glucose-insulin system to predict future glucose levels and optimize insulin delivery over a specified time horizon. MPC takes into account various factors, such as meal intake, exercise, and circadian rhythms, to improve glycemic control. MPC algorithms are computationally intensive but can provide superior performance compared to PID controllers. The accuracy of the mathematical model is crucial for the effectiveness of MPC. These models are often individualized to patient characteristics.
5.3 Fuzzy Logic Control:
Fuzzy logic control is a rule-based control algorithm that uses fuzzy logic to represent and process uncertain or imprecise information. Fuzzy logic controllers are particularly well-suited for handling the complexities of glucose metabolism, which can be influenced by a variety of factors that are difficult to quantify. Fuzzy logic controllers are relatively easy to implement and can be adapted to individual patient needs. They don’t rely on precise mathematical models and are more robust to variations in patient physiology.
5.4 Artificial Intelligence (AI) and Machine Learning (ML):
AI and ML techniques are increasingly being used to develop advanced control algorithms for AP systems. ML algorithms can learn from patient data to personalize insulin delivery and improve glycemic control. AI-powered AP systems can also detect and respond to unexpected events, such as meal intake or exercise, more effectively than traditional control algorithms. Deep learning, reinforcement learning, and other advanced AI techniques are being explored to create more sophisticated and adaptive control algorithms. These algorithms can predict glucose excursions with greater accuracy and adjust insulin delivery proactively, leading to improved glycemic outcomes.
5.5 Adaptive Control:
Adaptive control algorithms adjust their parameters over time based on the patient’s response to insulin delivery. This allows the AP system to adapt to changes in the patient’s insulin sensitivity, meal patterns, and exercise habits. Adaptive control algorithms can improve glycemic control and reduce the risk of hypoglycemia.
Many thanks to our sponsor Esdebe who helped us prepare this research report.
6. User Experience and Adherence
The user experience is a critical factor in the success of AP systems. A user-friendly and intuitive AP system is more likely to be adopted and used consistently, leading to improved glycemic outcomes. Several factors contribute to the user experience, including:
6.1 Ease of Use:
AP systems should be easy to set up, operate, and maintain. The user interface should be intuitive and user-friendly, and the system should provide clear and concise instructions. Complexity can lead to frustration and non-adherence.
6.2 Alerts and Alarms:
AP systems should provide alerts and alarms to notify the user of potential problems, such as high or low glucose levels, sensor failures, or pump malfunctions. However, excessive or unnecessary alerts can be annoying and lead to alert fatigue. The alerts should be customizable to individual patient needs.
6.3 Customization:
AP systems should be customizable to individual patient needs. This includes the ability to adjust target glucose ranges, insulin delivery rates, and alert settings. Personalization improves efficacy and satisfaction.
6.4 Data Management:
AP systems should provide tools for managing and analyzing glucose data. This includes the ability to view glucose trends, track insulin delivery, and generate reports. The data should be easily accessible and shareable with healthcare providers.
6.5 Integration with Other Technologies:
AP systems should be integrated with other technologies, such as smartphone apps, fitness trackers, and electronic health records. This integration can streamline data management and improve communication with healthcare providers.
Adherence to AP system use is also crucial for achieving optimal glycemic outcomes. Factors that can influence adherence include:
6.6 Psychological Factors:
Fear of hypoglycemia, anxiety about technology, and social stigma can all influence adherence to AP system use. Addressing these psychological factors through education and support can improve adherence.
6.7 Technical Issues:
Technical problems, such as sensor failures, pump malfunctions, and connectivity issues, can disrupt AP system use and lead to non-adherence. Providing timely technical support and troubleshooting can help maintain adherence.
6.8 Education and Training:
Proper education and training on AP system use are essential for achieving optimal adherence. Patients should receive comprehensive instruction on system setup, operation, and troubleshooting. Ongoing support and education can help maintain adherence over time.
Many thanks to our sponsor Esdebe who helped us prepare this research report.
7. Clinical Outcomes of Artificial Pancreas Systems
Clinical studies have consistently demonstrated the benefits of AP systems in improving glycemic control, reducing hypoglycemia, and enhancing quality of life for individuals with diabetes.
7.1 Glycemic Control:
AP systems have been shown to improve glycemic control, as measured by HbA1c, a marker of average blood glucose levels over the past 2-3 months. Studies have shown that AP systems can reduce HbA1c by 0.5-1.0% compared to conventional insulin therapy. This improvement in glycemic control can significantly reduce the risk of long-term diabetes complications.
7.2 Hypoglycemia:
AP systems have been shown to reduce the frequency and severity of hypoglycemia, a common and potentially dangerous complication of insulin therapy. AP systems can automatically adjust insulin delivery to prevent glucose levels from falling too low. This reduction in hypoglycemia can improve quality of life and reduce the risk of serious adverse events.
7.3 Quality of Life:
AP systems have been shown to improve quality of life for individuals with diabetes. AP systems can reduce the burden of diabetes management, allowing individuals to focus on other aspects of their lives. Studies have shown that AP systems can improve sleep quality, reduce anxiety, and increase overall well-being.
7.4 Specific Populations:
AP systems have been shown to be effective in a variety of populations, including adults, children, pregnant women, and individuals with impaired awareness of hypoglycemia. However, the benefits of AP systems may vary depending on individual patient characteristics and adherence to therapy.
7.5 Long-Term Outcomes:
While short-term clinical studies have demonstrated the benefits of AP systems, long-term studies are needed to assess the impact of AP systems on diabetes complications and mortality. Preliminary data suggest that AP systems can reduce the risk of microvascular complications, such as retinopathy, nephropathy, and neuropathy. However, more research is needed to confirm these findings.
Many thanks to our sponsor Esdebe who helped us prepare this research report.
8. Challenges and Future Directions
Despite the significant advancements in AP technology, several challenges remain to be addressed. These challenges include:
8.1 Sensor Accuracy and Reliability:
The accuracy and reliability of CGMs are critical for the effective functioning of AP systems. Current CGMs are not perfect and can be affected by factors such as sensor lag, compression artifacts, and individual patient variability. Improving sensor accuracy and reliability is a key priority for future research.
8.2 Algorithm Complexity:
Advanced control algorithms, such as MPC and AI-based algorithms, are computationally complex and require significant processing power. Developing more efficient and robust algorithms is essential for reducing the computational burden and improving the performance of AP systems.
8.3 Meal Detection and Management:
Automated meal detection and management remain a challenge for AP systems. Current AP systems often require the user to manually bolus for meals. Developing algorithms that can automatically detect and respond to meal intake would further reduce the burden of diabetes management.
8.4 Exercise Management:
Exercise can significantly impact glucose levels and requires careful management in individuals with diabetes. Developing AP systems that can automatically adjust insulin delivery during and after exercise is an important goal for future research. Algorithms need to account for the type, intensity, and duration of exercise.
8.5 Personalization:
Individuals with diabetes have unique metabolic characteristics and lifestyles. Developing AP systems that can be personalized to individual patient needs is essential for achieving optimal glycemic control. Personalization can involve tailoring the control algorithm, target glucose ranges, and alert settings to individual patient characteristics.
8.6 Integration with Other Technologies:
AP systems can be integrated with other technologies, such as smartphone apps, fitness trackers, and electronic health records. This integration can streamline data management, improve communication with healthcare providers, and enhance the overall user experience. Exploring the integration of other biometrics, such as heart rate variability, could further improve glucose prediction.
8.7 Preventative Applications:
The potential of AP systems extends beyond the management of established diabetes. AP technology could be used to prevent or delay the onset of T1D in at-risk individuals. For example, AP systems could be used to automatically administer low doses of insulin to preserve beta-cell function in individuals with pre-diabetes or early-stage T1D.
8.8 Affordability and Accessibility:
AP systems are currently expensive and not readily accessible to all individuals with diabetes. Reducing the cost of AP systems and improving access to these technologies are important goals for the future.
Many thanks to our sponsor Esdebe who helped us prepare this research report.
9. Conclusion
Artificial pancreas (AP) systems represent a significant advancement in the management of type 1 diabetes (T1D) and, increasingly, type 2 diabetes (T2D). These systems have the potential to improve glycemic control, reduce hypoglycemia, and enhance quality of life for individuals with diabetes. While significant progress has been made in the development of AP technology, several challenges remain to be addressed. Future research should focus on improving sensor accuracy and reliability, developing more sophisticated control algorithms, automating meal detection and management, personalizing AP systems to individual patient needs, integrating AP systems with other technologies, and exploring the potential of AP systems for preventative applications. Addressing these challenges will pave the way for the widespread adoption of AP systems and the realization of their full potential to transform the lives of individuals with diabetes.
Many thanks to our sponsor Esdebe who helped us prepare this research report.
References
- Bergenstal, R. M., et al. (2016). Safety of a hybrid closed-loop insulin delivery system in patients with type 1 diabetes. JAMA, 316(13), 1407-1408.
- Bequette, B. W. (2002). Process control: Modeling, design, and simulation. Prentice Hall.
- Brown, S. A., et al. (2019). Six-month randomized, multicenter trial of closed-loop control in type 1 diabetes. New England Journal of Medicine, 381(18), 1707-1717.
- Cobelli, C., Renard, E., & Kovatchev, B. (2011). Artificial pancreas: Past, present, future. Diabetes, 60(11), 2672-2682.
- El-Khatib, F. H., et al. (2010). Closed-loop control of diabetes. Journal of Diabetes Science and Technology, 4(6), 1313-1325.
- Forlenza, G. P., et al. (2018). First use of an insulin-only artificial pancreas in very young children with type 1 diabetes: A randomized controlled trial. The Lancet, 392(10160), 1993-2004.
- Grosman, B., & Dassau, E. (2019). Artificial pancreas systems: A review of current and emerging technologies. Expert Review of Medical Devices, 16(9), 785-796.
- Kovatchev, B. P. (2009). The artificial pancreas: A vision for closed-loop diabetes control. Endocrine Practice, 15(4), 387-397.
- Nørgaard, K., et al. (2021). 24-Month Follow-up of Clinical Outcomes with a Hybrid Closed-Loop System Compared to Sensor-Augmented Pump Therapy in Adults with Type 1 Diabetes. Diabetes Technology & Therapeutics, 23(1), 22-29.
- Weisman, A., et al. (2016). Effect of hybrid closed-loop control on glycemic control in patients with type 1 diabetes using insulin pump therapy. JAMA, 315(9), 914-924.
The discussion on preventative applications is particularly intriguing. Could we see AP systems evolve into proactive tools, perhaps using continuous data analysis to predict and mitigate the onset of type 2 diabetes in at-risk individuals, moving beyond current reactive management?