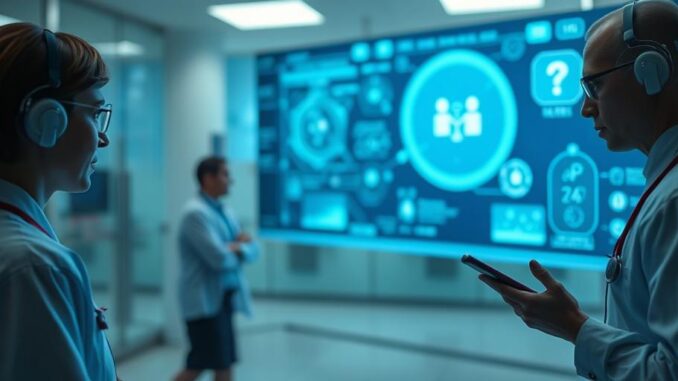
Summary
This article explores the rise of voice AI agents in healthcare, focusing on how developers are tackling the crucial issues of trust and explainability. It examines the innovative approaches used to build reliable and transparent AI systems for patient engagement and care coordination. The article also discusses the broader implications of explainable AI in medicine, highlighting its potential to revolutionize healthcare delivery and improve patient outcomes.
Start with a free consultation to discover how TrueNAS can transform your healthcare data management.
** Main Story**
AI Agents: Trust & Explainability in Healthcare
Artificial intelligence (AI) is rapidly transforming healthcare, and voice AI agents are emerging as a powerful tool for patient engagement and care coordination. However, the successful integration of AI in healthcare hinges on addressing two critical challenges: trust and explainability. This article delves into the innovative strategies developers are employing to build reliable and transparent AI systems that patients and healthcare providers can confidently embrace.
Building Trust in Voice AI
Trust is paramount in any healthcare interaction, and AI agents are no exception. Patients need assurance that the information and guidance they receive from these digital assistants are accurate, reliable, and aligned with established medical practices. Developers are tackling this challenge through several key approaches:
- Hallucination-Free AI: One major concern with traditional AI models is their tendency to “hallucinate,” or generate fabricated information. To mitigate this risk, companies like Infinitus are developing “hallucination-free” voice AI agents. These agents operate within a defined “Discrete Action Space,” limiting their responses to a pre-vetted set of clinically validated phrases, ensuring accuracy and compliance with regulatory standards.
- Real-Time Information Validation: HIPAA-compliant AI agents can validate information in real-time by cross-referencing patient data, payer plans, treatment areas, and other relevant sources. This ensures that the information provided to patients is consistent and up-to-date.
- Autonomous Self-Evaluation: To further enhance trust and accountability, some AI agents are equipped with self-monitoring capabilities. They continuously analyze their own responses for anomalies, contradictions, and gaps, alerting human overseers when necessary. This autonomous self-evaluation provides an additional layer of quality control and helps build confidence in the system’s reliability.
- Transparency and Clear Communication: Building trust also involves being upfront about the AI agent’s nature. These agents clearly identify themselves as digital assistants and communicate in a clear, empathetic manner, fostering a sense of transparency and authenticity.
The Importance of Explainability in Medical AI
Explainability is another crucial aspect of building trust in AI healthcare applications. It refers to the ability of an AI system to provide understandable explanations for its decisions and recommendations. This is particularly important in medicine, where both patients and clinicians need to understand the reasoning behind an AI’s output to make informed decisions. Explainable AI offers several key advantages:
- Supporting Shared Decision-Making: Explainable AI empowers patients and clinicians to engage in collaborative discussions about treatment options. By understanding the factors driving an AI’s recommendations, patients can actively participate in their healthcare decisions, leading to greater satisfaction and adherence to treatment plans.
- Enhancing Clinician Confidence: When clinicians understand how an AI model arrives at its conclusions, they are more likely to trust its output and integrate it into their workflows. This can lead to more efficient and effective care delivery.
- Detecting and Mitigating Bias: Explainable AI can help identify potential biases in the data used to train AI models, ensuring fairness and equity in healthcare delivery.
The Future of AI in Healthcare
The advancements in voice AI and explainable AI hold immense promise for the future of healthcare. These technologies have the potential to:
- Improve Patient Outcomes: By providing personalized, accessible, and timely information, AI agents can empower patients to take a more active role in managing their health, leading to better outcomes.
- Enhance Care Coordination: AI agents can streamline communication between patients, providers, and payers, facilitating smoother care transitions and reducing administrative burdens.
- Accelerate Medical Research: Explainable AI can help researchers gain a deeper understanding of disease mechanisms and accelerate the development of new treatments.
As AI continues to evolve, it is crucial that developers prioritize trust and explainability. By building transparent, accountable, and human-centered AI systems, we can unlock the full potential of this transformative technology to revolutionize healthcare and improve lives.
Given the emphasis on “hallucination-free” AI, how might we ensure that these pre-vetted phrases evolve to reflect advancements in medical knowledge without compromising their initial validation?
That’s a great point! Striking that balance between up-to-date knowledge and validated accuracy is key. Perhaps a tiered validation system, where updates undergo rigorous review before wider release, could be a solution. Continuous monitoring of real-world performance would also be crucial to flag any discrepancies or areas needing refinement.
Editor: MedTechNews.Uk
Thank you to our Sponsor Esdebe
Given the reliance on pre-vetted phrases, how can AI agents adapt to nuanced patient queries or unexpected scenarios that fall outside the defined “Discrete Action Space” while maintaining accuracy?
That’s a crucial question! One approach could involve a carefully designed escalation protocol. When an AI agent encounters a query outside its pre-vetted responses, it could seamlessly transfer the patient to a human expert, ensuring nuanced needs are met without sacrificing accuracy. This combines AI efficiency with human understanding.
Editor: MedTechNews.Uk
Thank you to our Sponsor Esdebe
The emphasis on “hallucination-free” AI is vital for building trust. Expanding on the Discrete Action Space concept, how might these AI agents be designed to recognize and flag novel or ambiguous patient inputs that require human intervention, ensuring continuous learning and refinement of the system?
That’s an important challenge! One approach could involve equipping agents with confidence scoring for inputs. Low confidence triggers a flag for human review, allowing for iterative refinement of the Discrete Action Space with new, validated phrases. This blends safety with continuous learning.
Editor: MedTechNews.Uk
Thank you to our Sponsor Esdebe
Hallucination-free AI, you say? Sounds a bit like my attempts at cooking after a long day. Sticking to pre-vetted recipes is definitely safer. Maybe these agents could share their ‘safe’ recipes…for, you know, optimal patient nourishment.