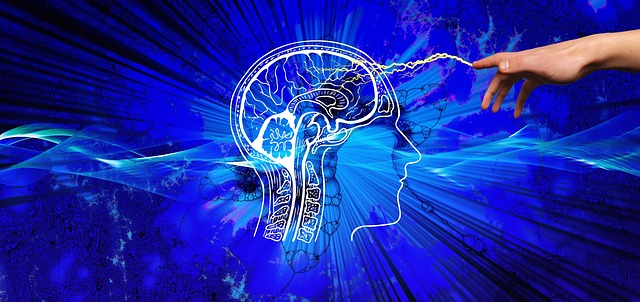
Abstract
Neuroimaging has revolutionized our understanding of the human brain, providing unprecedented insights into its structure, function, and dynamics. This report offers a comprehensive overview of advanced neuroimaging techniques, moving beyond traditional modalities like structural MRI and basic EEG. We delve into cutting-edge methods such as diffusion MRI (dMRI), functional near-infrared spectroscopy (fNIRS), magnetoencephalography (MEG), advanced electroencephalography (EEG) techniques including high-density EEG and source localization, positron emission tomography (PET) with novel radioligands, and the rapidly evolving field of multimodal neuroimaging. We discuss the underlying principles, strengths, limitations, and emerging applications of each technique. Furthermore, we explore the integration of artificial intelligence (AI) and machine learning (ML) to enhance neuroimaging data analysis, improve diagnostic accuracy, and predict treatment outcomes. Finally, we address the ethical considerations surrounding neuroimaging and highlight future research directions that hold promise for advancing our knowledge of the brain and improving patient care.
Many thanks to our sponsor Esdebe who helped us prepare this research report.
1. Introduction
Neuroimaging encompasses a diverse array of techniques that allow us to visualize and study the brain in vivo. These methods provide invaluable information about brain anatomy, physiology, and function, enabling researchers and clinicians to investigate neurological and psychiatric disorders, monitor treatment efficacy, and explore the neural basis of cognition. While structural MRI and basic EEG remain essential tools, advancements in neuroimaging technology have led to the development of more sophisticated and informative modalities. This report aims to provide a comprehensive overview of these advanced techniques, highlighting their potential to transform our understanding of the brain and improve patient outcomes. The report will be accessible to experts in the field and will attempt to provide a level of detail that exceeds that found in general review papers. The report will also highlight some key areas for future research.
Many thanks to our sponsor Esdebe who helped us prepare this research report.
2. Diffusion MRI (dMRI)
Diffusion MRI (dMRI) is a powerful non-invasive technique that probes the microstructural organization of brain tissue by measuring the diffusion of water molecules. Unlike conventional MRI, which provides information about macroscopic anatomy, dMRI is sensitive to the microscopic environment, including the orientation and integrity of white matter fibers. The most widely used dMRI technique is diffusion tensor imaging (DTI), which models water diffusion as a tensor and provides measures of fiber directionality and coherence, such as fractional anisotropy (FA) and mean diffusivity (MD). However, DTI has limitations in resolving complex fiber architectures, such as crossing fibers. More advanced dMRI techniques, such as diffusion spectrum imaging (DSI) and constrained spherical deconvolution (CSD), overcome these limitations by modeling the diffusion signal as a probability distribution function (PDF), allowing for the reconstruction of complex fiber pathways.
2.1 Applications of dMRI
dMRI has a wide range of applications in neuroscience and clinical practice. It is used to study white matter development and aging, investigate the effects of neurological disorders such as multiple sclerosis and stroke, and map the structural connectivity of the brain. DTI has been used to identify white matter abnormalities in patients with traumatic brain injury (TBI) and to track the recovery of white matter integrity following injury. DSI and CSD have been used to map the complex fiber pathways that connect different brain regions, providing insights into the structural basis of cognitive functions. In recent years, dMRI has also been used to guide neurosurgical planning, allowing surgeons to avoid critical white matter tracts during surgery.
2.2 Limitations of dMRI
Despite its many advantages, dMRI has some limitations. The acquisition and processing of dMRI data can be time-consuming and computationally intensive. The interpretation of dMRI results can be challenging, as the diffusion signal is affected by multiple factors, including fiber orientation, fiber density, and myelin content. Furthermore, dMRI is sensitive to motion artifacts, which can distort the diffusion signal and reduce the accuracy of the results. Future research should focus on developing more robust and efficient dMRI acquisition and analysis methods, as well as on developing better models of the relationship between diffusion signal and brain microstructure.
Many thanks to our sponsor Esdebe who helped us prepare this research report.
3. Functional Near-Infrared Spectroscopy (fNIRS)
Functional near-infrared spectroscopy (fNIRS) is a non-invasive optical neuroimaging technique that measures changes in cerebral blood flow by detecting changes in the absorption of near-infrared light. fNIRS uses light in the 650-950 nm range, which can penetrate the skull and brain tissue. Hemoglobin absorbs light in this range, and the amount of light absorbed depends on the concentration of oxygenated and deoxygenated hemoglobin. By measuring the changes in light absorption, fNIRS can provide information about brain activity. fNIRS is relatively inexpensive, portable, and safe, making it a useful tool for studying brain function in a variety of settings. It is particularly well-suited for studying brain activity in infants and children, as it does not require the participant to remain still, and can be used at the bedside.
3.1 Applications of fNIRS
fNIRS has been used to study a wide range of cognitive functions, including attention, memory, language, and social cognition. It has also been used to investigate the neural basis of neurological and psychiatric disorders, such as autism spectrum disorder (ASD) and schizophrenia. fNIRS has shown promise as a tool for brain-computer interfaces (BCIs), allowing individuals to control external devices using their brain activity. One of the major applications of fNIRS is in mapping cortical activity during language tasks. Researchers have been able to identify brain regions involved in language processing, such as Broca’s and Wernicke’s areas, and to study how these regions are affected by language disorders. Another emerging area is the application of fNIRS in the development of BCIs for communication and motor control. fNIRS-based BCIs have been used to allow individuals with paralysis to control a computer cursor or a robotic arm.
3.2 Limitations of fNIRS
fNIRS has some limitations. The spatial resolution of fNIRS is lower than that of other neuroimaging techniques, such as fMRI. fNIRS is also sensitive to artifacts from scalp blood flow and movement. Furthermore, fNIRS can only measure activity in the superficial layers of the cortex. Advancements in fNIRS technology, such as high-density fNIRS and diffuse optical tomography (DOT), are improving the spatial resolution and depth sensitivity of fNIRS. Future research should focus on developing better methods for reducing artifacts and on integrating fNIRS with other neuroimaging techniques to obtain a more complete picture of brain function.
Many thanks to our sponsor Esdebe who helped us prepare this research report.
4. Magnetoencephalography (MEG)
Magnetoencephalography (MEG) is a non-invasive neuroimaging technique that measures the magnetic fields produced by electrical currents in the brain. MEG has excellent temporal resolution, allowing it to capture the rapid dynamics of brain activity. MEG is also relatively insensitive to artifacts from muscle activity and eye movements, making it a useful tool for studying brain function in awake, behaving subjects. MEG systems typically consist of an array of superconducting quantum interference devices (SQUIDs) that are housed in a magnetically shielded room. The SQUIDs detect the tiny magnetic fields produced by neuronal activity in the brain. The data acquired from the MEG system is then used to reconstruct the location and timing of the underlying neural activity. MEG is unique in being able to directly measure neural activity, whereas methods such as fMRI are measuring blood flow which is only an indirect measure of neural activity.
4.1 Applications of MEG
MEG has a wide range of applications in neuroscience and clinical practice. It is used to study the neural basis of cognitive functions, such as perception, attention, and memory. MEG is also used to identify epileptic foci in patients with epilepsy and to guide neurosurgical planning. MEG has been used to investigate the neural mechanisms underlying various neurological and psychiatric disorders, such as schizophrenia, autism, and Alzheimer’s disease. One of the key applications of MEG is in presurgical planning for epilepsy surgery. MEG can be used to localize the epileptogenic zone, which is the area of the brain that generates seizures. This information can help surgeons to remove the epileptogenic zone while sparing healthy brain tissue. MEG is also used to study the effects of drugs on brain activity, which can help to develop new treatments for neurological and psychiatric disorders.
4.2 Limitations of MEG
MEG has some limitations. MEG systems are expensive and require specialized facilities. The spatial resolution of MEG is lower than that of fMRI. The interpretation of MEG data can be challenging, as the magnetic fields measured by MEG are affected by multiple factors, including the location and orientation of the neural sources and the conductivity of the head tissues. Future research should focus on developing more affordable and portable MEG systems, as well as on developing better methods for source localization and data analysis. Developing algorithms that can better account for variations in head shape and tissue conductivity is crucial for improving the accuracy of MEG source localization.
Many thanks to our sponsor Esdebe who helped us prepare this research report.
5. Advanced EEG Techniques
Electroencephalography (EEG) is a non-invasive neuroimaging technique that measures the electrical activity of the brain using electrodes placed on the scalp. EEG is relatively inexpensive, portable, and easy to use, making it a widely used tool for studying brain function in both clinical and research settings. While conventional EEG provides valuable information about brain activity, advanced EEG techniques, such as high-density EEG and source localization, can provide more detailed and accurate information about the underlying neural processes. High-density EEG uses a larger number of electrodes than conventional EEG, which improves the spatial resolution of the EEG signal. Source localization techniques use mathematical algorithms to estimate the location of the neural sources that generate the EEG signal. These techniques are particularly valuable for studying cognitive processes that involve multiple brain regions.
5.1 High-Density EEG
High-density EEG (hdEEG) uses a larger number of electrodes (typically 64 or more) compared to conventional EEG (typically 19 or 32 electrodes). This increased electrode density improves the spatial sampling of the scalp EEG signal, which can lead to more accurate source localization and better characterization of brain activity. hdEEG has been used to study a wide range of cognitive functions, including attention, memory, and language. It has also been used to investigate the neural basis of neurological and psychiatric disorders, such as epilepsy, schizophrenia, and autism. The improved spatial resolution of hdEEG allows researchers to differentiate between activity originating from closely located brain regions. This is particularly useful in studying cognitive processes that involve multiple brain areas working in concert.
5.2 EEG Source Localization
EEG source localization is a technique that uses mathematical algorithms to estimate the location of the neural sources that generate the EEG signal. Source localization algorithms typically use a head model, which describes the electrical properties of the head tissues, to calculate the forward solution, which predicts the EEG signal that would be generated by a given source location. The inverse problem is then solved to estimate the source location that best explains the observed EEG signal. There are various source localization algorithms, including dipole fitting, minimum norm estimation, and beamforming. The choice of algorithm depends on the specific application and the assumptions that are made about the underlying neural sources. EEG source localization is a complex and computationally intensive process, but it can provide valuable information about the neural generators of EEG activity.
5.3 Applications of Advanced EEG Techniques
Advanced EEG techniques have a wide range of applications in neuroscience and clinical practice. They are used to study the neural basis of cognitive functions, such as attention, memory, and language. They are also used to identify epileptic foci in patients with epilepsy and to guide neurosurgical planning. Advanced EEG techniques have been used to investigate the neural mechanisms underlying various neurological and psychiatric disorders, such as schizophrenia, autism, and Alzheimer’s disease. In cognitive neuroscience, hdEEG and source localization are used to investigate the neural correlates of various cognitive processes, such as working memory, decision-making, and language comprehension. In clinical neurology, these techniques are used to identify the source of epileptic seizures and to guide surgical resection of the epileptogenic zone. They are also used to monitor brain activity during anesthesia and to assess the severity of traumatic brain injury.
5.4 Limitations of Advanced EEG Techniques
Despite their many advantages, advanced EEG techniques have some limitations. The spatial resolution of EEG is still lower than that of fMRI. The accuracy of source localization depends on the accuracy of the head model and the assumptions that are made about the underlying neural sources. Furthermore, EEG is sensitive to artifacts from muscle activity and eye movements. Future research should focus on developing better head models and source localization algorithms, as well as on developing better methods for reducing artifacts. This includes incorporating individual head anatomy from MRI scans into the head model, as well as developing adaptive filtering techniques to remove artifacts from muscle activity and eye movements.
Many thanks to our sponsor Esdebe who helped us prepare this research report.
6. Positron Emission Tomography (PET) with Novel Radioligands
Positron emission tomography (PET) is a neuroimaging technique that uses radioactive tracers to measure various aspects of brain function, such as glucose metabolism, blood flow, and neurotransmitter activity. Traditional PET studies have used radioligands to measure glucose metabolism (FDG-PET) and blood flow (H2O-PET). However, the development of novel radioligands has expanded the range of applications of PET, allowing researchers and clinicians to study a wider range of brain processes. These novel radioligands can target specific neurotransmitter receptors, enzymes, and other proteins in the brain.
6.1 Applications of PET with Novel Radioligands
PET with novel radioligands has a wide range of applications in neuroscience and clinical practice. It is used to study the role of neurotransmitters in various neurological and psychiatric disorders, such as Parkinson’s disease, Alzheimer’s disease, and schizophrenia. PET is also used to monitor the effects of drugs on brain neurotransmitter systems. For example, radioligands that bind to dopamine receptors can be used to study the effects of drugs that affect dopamine neurotransmission, such as antipsychotics and stimulants. Radioligands that bind to amyloid plaques and tau tangles can be used to detect and monitor the progression of Alzheimer’s disease. The use of PET imaging to detect early signs of neurodegenerative diseases is a rapidly growing area of research. PET imaging is also being used to guide the development of new drugs for these diseases.
6.2 Limitations of PET
PET has some limitations. PET imaging requires the use of radioactive materials, which poses a potential risk to patients and staff. The spatial resolution of PET is lower than that of MRI. The availability of novel radioligands is limited. Furthermore, PET imaging is relatively expensive. Future research should focus on developing new and safer radioligands, as well as on improving the spatial resolution of PET. Developing new radioligands that target specific proteins involved in neurodegenerative diseases is a high priority. Efforts are also being made to develop hybrid PET/MRI scanners, which would combine the high spatial resolution of MRI with the functional information provided by PET.
Many thanks to our sponsor Esdebe who helped us prepare this research report.
7. Multimodal Neuroimaging
Multimodal neuroimaging involves the simultaneous or sequential acquisition of data from two or more neuroimaging modalities. This approach can provide a more comprehensive picture of brain function than can be obtained from any single modality. By combining the strengths of different modalities, multimodal neuroimaging can overcome the limitations of individual techniques and provide more accurate and informative results. For example, combining EEG and fMRI can provide both high temporal resolution and high spatial resolution. Combining structural MRI and diffusion MRI can provide information about both brain anatomy and white matter connectivity. The integration of data from multiple modalities requires sophisticated data analysis techniques, but the potential benefits are substantial.
7.1 Examples of Multimodal Neuroimaging
There are many examples of multimodal neuroimaging studies. EEG-fMRI is a commonly used combination that allows researchers to study the relationship between electrical brain activity and hemodynamic responses. MEG-MRI is another popular combination that provides both high temporal resolution and high spatial resolution. PET-MRI is used to study the relationship between brain metabolism and brain structure. Near-infrared spectroscopy (NIRS)-EEG is a combination of non-invasive techniques used in cognitive studies, providing information on cortical hemodynamics and electrophysiological activity. Multimodal approaches are particularly valuable for studying complex cognitive processes and neurological disorders that involve multiple brain regions and systems. For example, EEG-fMRI has been used to study the neural mechanisms underlying epileptic seizures. MEG-MRI has been used to study the neural basis of schizophrenia. PET-MRI has been used to study the effects of aging on brain metabolism and structure.
7.2 Challenges of Multimodal Neuroimaging
Multimodal neuroimaging presents several challenges. The acquisition and processing of data from multiple modalities can be complex and time-consuming. The integration of data from different modalities requires sophisticated data analysis techniques. Furthermore, the interpretation of multimodal data can be challenging, as the different modalities may provide conflicting information. Future research should focus on developing more efficient data acquisition and analysis methods, as well as on developing better models for integrating data from different modalities. Developing standardized protocols for multimodal neuroimaging studies is crucial for ensuring the reproducibility of results. This includes establishing guidelines for data acquisition, preprocessing, and analysis, as well as developing common data formats and software tools.
Many thanks to our sponsor Esdebe who helped us prepare this research report.
8. Artificial Intelligence (AI) and Machine Learning (ML) in Neuroimaging
The application of artificial intelligence (AI) and machine learning (ML) techniques is transforming the field of neuroimaging. AI/ML algorithms can be used to analyze large neuroimaging datasets, identify subtle patterns that may be missed by human observers, and make predictions about brain function and behavior. These techniques have the potential to improve diagnostic accuracy, predict treatment outcomes, and personalize treatment strategies. AI/ML algorithms are being used to automate many of the tedious and time-consuming tasks involved in neuroimaging data analysis, such as image segmentation and registration. They are also being used to develop new methods for image reconstruction and artifact removal. The use of AI/ML in neuroimaging is a rapidly growing area of research, and it is expected to have a significant impact on the future of the field.
8.1 Applications of AI/ML in Neuroimaging
AI/ML algorithms have a wide range of applications in neuroimaging. They are used to classify patients with neurological and psychiatric disorders, predict the progression of disease, and identify biomarkers that can be used to monitor treatment response. AI/ML algorithms are also being used to decode brain activity from neuroimaging data, allowing researchers to study the neural basis of cognition and behavior. In clinical practice, AI/ML algorithms are being used to assist radiologists in the diagnosis of brain tumors and other neurological conditions. They are also being used to predict which patients are most likely to benefit from specific treatments. AI/ML algorithms are also being used to develop personalized treatment plans for patients with neurological disorders. For example, AI/ML algorithms can be used to predict the optimal dose of medication for a given patient, based on their individual brain characteristics.
8.2 Challenges of AI/ML in Neuroimaging
AI/ML in neuroimaging faces several challenges. One major challenge is the need for large, high-quality datasets to train the algorithms. Neuroimaging data can be expensive and time-consuming to acquire, and it is often difficult to obtain large datasets that are representative of the population of interest. Another challenge is the interpretability of AI/ML models. Many AI/ML algorithms are “black boxes,” meaning that it is difficult to understand how they make their predictions. This lack of interpretability can make it difficult to trust the results of AI/ML analyses. Future research should focus on developing more interpretable AI/ML algorithms, as well as on developing methods for validating AI/ML models. Developing methods for transferring AI/ML models trained on one dataset to another dataset is also an important goal. This would allow researchers to leverage existing datasets to train AI/ML models for new applications.
Many thanks to our sponsor Esdebe who helped us prepare this research report.
9. Ethical Considerations
The increasing sophistication and power of neuroimaging technologies raise a number of ethical considerations. Neuroimaging data can reveal sensitive information about an individual’s brain structure, function, and potential predispositions to neurological and psychiatric disorders. This information could be used to discriminate against individuals or to make predictions about their behavior that are not accurate. It is important to ensure that neuroimaging data is used responsibly and ethically. Data privacy and security are paramount. Strict protocols must be in place to protect the confidentiality of neuroimaging data and to prevent unauthorized access. Informed consent is essential. Participants in neuroimaging studies must be fully informed about the potential risks and benefits of the research and must have the right to withdraw from the study at any time. Researchers must also be aware of the potential for incidental findings, such as brain tumors, and must have a plan in place for addressing these findings.
Many thanks to our sponsor Esdebe who helped us prepare this research report.
10. Future Directions
The field of neuroimaging is rapidly evolving, and there are many exciting directions for future research. One major area of focus is the development of new and more powerful neuroimaging techniques. This includes developing higher-resolution imaging techniques, as well as developing techniques that can measure brain activity with greater temporal precision. Another important area of focus is the development of new methods for analyzing neuroimaging data. This includes developing AI/ML algorithms that can automatically extract meaningful information from neuroimaging data, as well as developing methods for integrating data from multiple neuroimaging modalities. Future research should also focus on using neuroimaging to study the neural basis of complex cognitive processes and neurological disorders. This includes using neuroimaging to study the effects of aging on the brain, as well as using neuroimaging to develop new treatments for neurological and psychiatric disorders. Personalized medicine, guided by neuroimaging biomarkers, holds great promise for improving patient outcomes. By tailoring treatment strategies to individual brain characteristics, we can maximize the effectiveness of interventions and minimize the risk of side effects.
Many thanks to our sponsor Esdebe who helped us prepare this research report.
11. Conclusion
Neuroimaging has become an indispensable tool for studying the human brain, providing valuable insights into its structure, function, and dynamics. Advanced neuroimaging techniques, such as dMRI, fNIRS, MEG, advanced EEG, and PET with novel radioligands, have significantly expanded our ability to investigate the brain in vivo. The integration of AI/ML is further revolutionizing the field, enhancing data analysis, improving diagnostic accuracy, and predicting treatment outcomes. While ethical considerations remain paramount, the potential benefits of neuroimaging for advancing our understanding of the brain and improving patient care are immense. Continued research and development in neuroimaging technologies and methodologies will undoubtedly lead to even greater breakthroughs in the years to come.
Many thanks to our sponsor Esdebe who helped us prepare this research report.
References
- Bandettini, P. A. (2009). What’s new in neuroimaging methods? Annals of the New York Academy of Sciences, 1156, 260-293.
- Buxton, R. B. (2002). Introduction to functional magnetic resonance imaging: principles and techniques. Cambridge University Press.
- Cabeza, R., & Nyberg, L. (2000). Imaging cognition: an empirical review of PET and fMRI studies of working memory, perception, and attention. Journal of Cognitive Neuroscience, 12(1), 1-47.
- Cohen, M. X. (2017). Analyzing neural time series data: theory and practice. MIT press.
- Friston, K. J. (2009). Modalities, models, and inferences in imaging neuroscience. Neuron, 62(4), 584-595.
- Glover, G. H. (2011). Overview of functional magnetic resonance imaging. Neurosurgery clinics of North America, 22(2), 133-139.
- Hämäläinen, M., Hari, R., Ilmoniemi, R. J., Knuutila, J., & Lounasmaa, O. V. (1993). Magnetoencephalography—theory, instrumentation, and applications to noninvasive studies of the working human brain. Reviews of Modern Physics, 65(2), 413.
- Logothetis, N. K. (2008). What we can do and what we cannot do with fMRI. Nature, 453(7197), 869-878.
- Poldrack, R. A., Mumford, J. A., & Nichols, T. E. (2011). Handbook of functional MRI data analysis. Cambridge University Press.
- Raichle, M. E. (2010). Two views of brain function. Trends in cognitive sciences, 14(4), 180-190.
- Wandell, B. A. (2011). Diffusion MRI. Annual review of neuroscience, 34, 49-64.
The discussion on ethical considerations is crucial. As neuroimaging becomes more sophisticated, how do we ensure equitable access to these technologies and protect against potential misuse of the data, especially in vulnerable populations?
Thank you for highlighting the critical ethical considerations! Equitable access is definitely a challenge. Perhaps a tiered system of resource allocation, coupled with stringent data protection policies and community involvement, could help to address this. What are your thoughts on these potential solutions?
Editor: MedTechNews.Uk
Thank you to our Sponsor Esdebe
This report highlights the remarkable potential of multimodal neuroimaging. Combining techniques such as MEG-MRI and NIRS-EEG can offer a more comprehensive understanding of brain function. Further standardization in data acquisition and analysis would significantly advance the field.