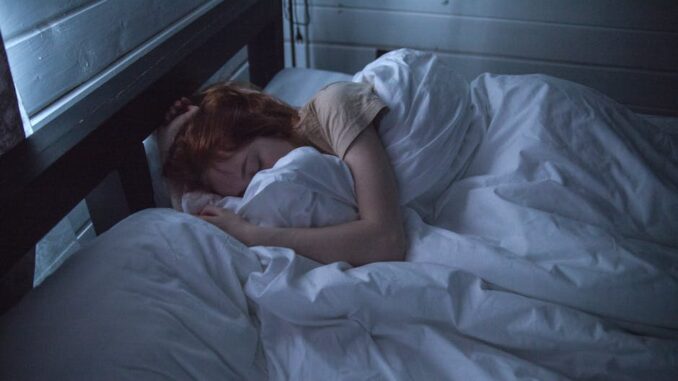
Summary
Researchers at Mount Sinai Hospital developed an AI algorithm that analyzes video recordings of sleep tests to diagnose REM sleep behavior disorder (RBD) with 92% accuracy. This breakthrough offers a more efficient and accurate way to diagnose RBD, a common precursor to Parkinson’s disease and dementia. Early detection of RBD could lead to earlier interventions for these neurological conditions.
** Main Story**
AI is rapidly transforming healthcare, and a recent breakthrough by the Mount Sinai Hospital research team highlights its potential to revolutionize sleep disorder diagnosis. The team developed an AI-powered algorithm that analyzes standard 2D video recordings from routine sleep studies to detect REM sleep behavior disorder (RBD) with remarkable accuracy. This innovative approach streamlines the diagnostic process and paves the way for personalized treatment plans and earlier intervention for neurological conditions like Parkinson’s disease and dementia.
The Challenge of Diagnosing RBD
RBD, a sleep disorder characterized by abnormal movements during REM sleep, affects over one million Americans and often precedes Parkinson’s disease or dementia by 10-15 years. Diagnosing RBD has been challenging due to several factors. Relying solely on patient-reported dream enactment episodes is unreliable, as many individuals with RBD experience subtle twitches that go unnoticed. Furthermore, symptoms of RBD can mimic other sleep disorders like sleep apnea, making accurate diagnosis difficult even with questionnaires. The gold standard for diagnosis has been in-lab sleep studies, but these are time-consuming, resource-intensive, and subject to human error.
Mount Sinai’s AI Solution
Recognizing the limitations of current diagnostic methods, the Mount Sinai team sought to automate the process using AI. Their algorithm analyzes video recordings taken during standard sleep tests, focusing on the frequency and patterns of body movements during REM sleep. Previous attempts at automated RBD detection relied on specialized 3D cameras, which added complexity and cost to sleep studies. The Mount Sinai algorithm, however, utilizes readily available 2D cameras typically found in sleep labs, making it a more practical and cost-effective solution.
How the Algorithm Works
The algorithm analyzes the video data, extracting key features of movement such as frequency, proportion of time spent moving during REM, magnitude, velocity, and immobility ratio. These features are then used to classify individuals as having RBD or not. The team trained and tested the algorithm on a large dataset of sleep study recordings from patients with and without RBD, including individuals with other sleep disorders.
Impressive Results and Implications
The results of the study were impressive. The AI algorithm achieved an accuracy rate of 91.9% in detecting RBD, surpassing previous methods and offering a significant improvement over subjective assessments and questionnaires. Importantly, the algorithm correctly identified RBD even in cases where movements were not readily apparent to human observers during the sleep test. This high level of accuracy has significant implications for clinical practice. By integrating the algorithm into sleep test analysis, clinicians can improve diagnostic efficiency, reduce missed diagnoses, and provide more timely and personalized treatment plans.
A New Era of Sleep Disorder Diagnosis
The Mount Sinai team’s work heralds a new era in sleep disorder diagnosis. The AI algorithm provides a streamlined, objective, and accurate method for detecting RBD. This early detection is crucial, as it allows for earlier intervention and potentially opens a window of opportunity for developing therapies that could delay or prevent the onset of Parkinson’s disease and dementia. Moreover, the algorithm’s reliance on standard 2D video recordings makes it readily implementable in existing sleep labs, paving the way for widespread adoption and improved patient care.
This AI-powered approach is not limited to RBD. It has the potential to be adapted and applied to other sleep disorders, further revolutionizing how sleep medicine is practiced and ultimately improving the lives of millions affected by these conditions. As AI continues to advance, we can expect even more sophisticated diagnostic and treatment tools to emerge, transforming healthcare and leading to better patient outcomes. This breakthrough in sleep disorder diagnosis is a testament to the power of AI to address unmet medical needs and improve the quality of life for patients worldwide.
The algorithm’s ability to leverage standard 2D video recordings is particularly exciting. Could this approach be adapted for home sleep monitoring, potentially broadening access to early RBD detection?
That’s a great point! The possibility of adapting the algorithm for home sleep monitoring is definitely something to consider. Broadening access could lead to earlier detection and intervention, potentially making a huge difference for many. The use of 2D video enhances accessibility and practicality.
Editor: MedTechNews.Uk
Thank you to our Sponsor Esdebe
92% accuracy? I bet the other 8% were just really dedicated method actors dreaming they *didn’t* have RBD. Wonder if the algorithm can tell the difference between a dream about being chased by zombies and actual nocturnal thrashing?
That’s a hilarious thought! It does bring up the important point about distinguishing between harmless dream enactment and true RBD-related movements. Further research could definitely explore refining the algorithm to account for more nuanced movement patterns and dream content cues. Thanks for the interesting perspective!
Editor: MedTechNews.Uk
Thank you to our Sponsor Esdebe
The algorithm’s ability to identify subtle movements undetectable by human observation is a significant advancement. This suggests potential for identifying other movement-related conditions beyond RBD through similar AI analysis of sleep patterns.
That’s an excellent point! Exploring applications for other movement-related conditions is definitely a promising avenue for future research. The sensitivity of the algorithm could potentially be leveraged to detect subtle indicators of various neurological disorders during sleep. This could have a huge impact on early diagnosis and treatment!
Editor: MedTechNews.Uk
Thank you to our Sponsor Esdebe
92% accuracy, eh? Does that mean my dreams of winning the lottery are actually just pre-Parkinson’s fidgeting caught on camera? Suddenly feeling less lucky, more… statistically likely to develop neurological issues.
That’s a funny and insightful take! While we can’t promise it’ll predict lottery wins, distinguishing between random movements and early RBD signs is exactly what makes the algorithm valuable. Hopefully, with further refinement, we can distinguish a genuine “winning the lottery” dream reaction from something more serious!
Editor: MedTechNews.Uk
Thank you to our Sponsor Esdebe
Given the high accuracy, could the algorithm be refined to quantify the severity of RBD, potentially aiding in tracking disease progression or response to therapeutic interventions?