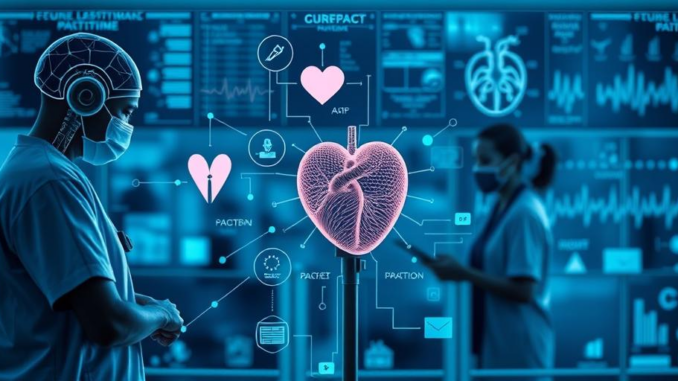
Summary
This article explores the groundbreaking use of AI in cardiology to predict bleeding and stroke risks for atrial fibrillation (AFib) patients. AI models analyze patient data to provide personalized risk assessments, outperforming traditional methods. This technology promises safer and more effective treatment strategies for AFib patients.
Healthcare data growth can be overwhelming scale effortlessly with TrueNAS by Esdebe.
** Main Story**
Okay, let’s talk about how AI is changing the game when it comes to predicting risk for patients with Atrial Fibrillation, or AFib. It’s a pretty big deal. AFib, as you know, is that common heart rhythm issue that can seriously bump up the risk of stroke. And managing that risk? It’s critical, especially for patients who are on DOACs—direct oral anticoagulants.
These drugs, while helpful, can also increase the risk of bleeding. So, traditionally, doctors have been using clinical guidelines to walk this tightrope, balancing the risk of stroke against the risk of bleeding. But here’s the thing: every patient is different; their individual profiles vary so widely that it’s like trying to fit a square peg in a round hole. That’s where AI comes in, and it’s not just a minor upgrade; it’s a whole new approach to AFib risk management.
How AI Steps Up in AFib Risk Prediction
Think of AI models as incredibly diligent students. They get fed massive amounts of patient data—health records, medical history, the works—and they learn to spot patterns that we, as humans, might miss. We’re talking about subtle indicators that could mean the difference between a good outcome and a bad one. These models dig into everything:
- Age, of course.
- Medication history—what they’re taking, what they’ve taken.
- Genetics—a big piece of the puzzle.
- Lifestyle—diet, exercise, smoking habits.
- And a whole host of other health indicators.
It’s a 360-degree view of the patient, and by factoring all of this in, AI can predict the likelihood of a stroke or a major bleeding event with surprising accuracy. I remember reading about a study last year, where an AI model outperformed traditional risk scores by, like, 20%. The end result? Cardiologists get more informed, more personalized treatment recommendations for each patient. It’s a win-win.
The Upsides of AI-Powered Risk Prediction
What’s so great about AI in AFib risk prediction? Let’s break it down:
- Personalized Treatment: This is huge. We’re not just giving everyone the same cookie-cutter approach anymore. AI allows us to tailor treatment specifically to each patient’s unique risk profile. And this is so crucial! Because a treatment that works for one person can actually harm another.
- Improved Accuracy: It’s not just a little better; AI models consistently outperform traditional risk scoring systems. That means fewer misclassifications and, ultimately, better decisions. For example, traditional methods might say a patient is low risk, but AI picks up on subtle factors and flags them for closer monitoring, something I’ve seen happen at my own hospital. It’s like having an extra pair of eyes that never get tired.
- Early Intervention: Time is critical, and with AI, we can identify high-risk individuals much earlier. This allows for timely intervention and potentially prevents adverse events from even happening.
- Continuous Learning: The beauty of AI is that it’s not static. These algorithms are constantly learning from new data, adapting, and refining their predictions over time. It’s like they’re getting smarter every day, which is something that you can’t really say about traditional risk scoring systems. This iterative nature is key to improving long-term patient care.
Real-World Advantages for Patients and Doctors
So, who benefits from all of this? Well, pretty much everyone:
- Enhanced Patient Safety: I think this is the most important. More accurate risk assessment means safer anticoagulation management and minimizes the risk of complications. Less complications are only beneficial to patients after all.
- Improved Patient Outcomes: Personalized treatment strategies lead to better overall outcomes for AFib patients. It’s not just about avoiding negative events; it’s about maximizing positive ones, like improving quality of life and reducing hospital readmissions.
- Streamlined Workflow: AI tools automate complex risk assessments, freeing up clinicians’ time and improving workflow efficiency. Now, your doctors are more happy. It’s less time crunching numbers and more time focusing on patient care.
- Empowered Decision-Making: Healthcare professionals can leverage AI insights to make more confident, data-driven decisions. You know it, i know it, making those confident decisions can be very helpful.
Roadblocks and Future Steps
It’s not all sunshine and roses, though. There are definitely challenges we need to address.
- Data Bias: AI models are only as good as the data they’re trained on. And if that data is biased, the AI will be biased, too. We need to make sure these models are trained on diverse and representative datasets to ensure equitable outcomes for all patients. Data needs to come from everyone in the population, if not, the algorithm may be biased, and thus unethical to use.
- Data Privacy: Protecting patient data is, of course, non-negotiable. Robust security measures are absolutely necessary to maintain confidentiality and comply with regulations. No patients should have their private medical records leaked.
- Regulatory Frameworks: We need clear regulatory guidelines to ensure the safe and responsible implementation of AI in healthcare. How do we validate these models? Who is responsible if something goes wrong? These are the questions that regulators are grappling with right now. Hopefully the regulatory side can keep up with the pace of innovation of the algorithm side.
Despite these challenges, the future of AI in cardiology is incredibly bright. Ongoing research and development promise to further enhance the accuracy and capabilities of these tools. AI isn’t just a nice-to-have; it’s becoming an essential component in delivering cutting-edge cardiovascular care. As of today, March 27, 2025, this information is current, but this field is evolving so rapidly that new developments will likely expand upon these capabilities in the very near future. It’s an exciting time to be in healthcare, don’t you think?
The article highlights AI’s ability to learn from diverse patient data. Considering the challenge of data bias, how can we proactively audit AI models in cardiology to ensure equitable risk predictions across different demographic groups?
That’s a crucial point about data bias! Proactive auditing is key. One promising approach is ‘adversarial debiasing,’ where we train AI to identify and mitigate bias during the model’s development. Continuous monitoring after deployment is essential, too, to catch any drift in performance across different demographic groups. Thanks for raising this important issue!
Editor: MedTechNews.Uk
Thank you to our Sponsor Esdebe