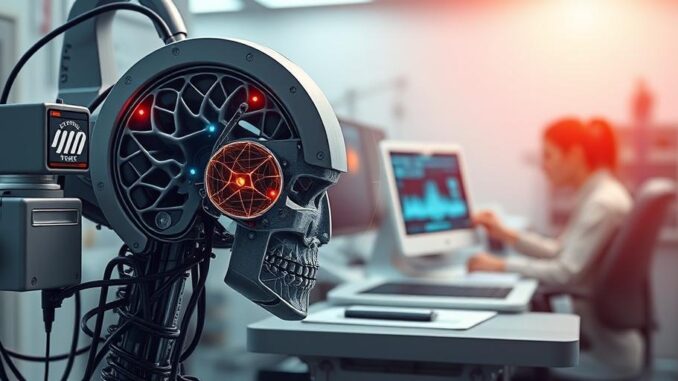
Summary
This article explores the groundbreaking use of machine learning in detecting neurodegenerative diseases like Alzheimer’s and Parkinson’s. We discuss the various machine learning techniques employed, the types of data used, and the potential for earlier diagnosis and personalized treatment. This technology offers hope for improving patient outcomes and revolutionizing how we approach these debilitating conditions.
** Main Story**
Neurodegenerative diseases, like Alzheimer’s and Parkinson’s, they’re a huge problem worldwide. Traditional diagnosis? Often it’s just waiting and watching for symptoms to get really bad, which limits what we can actually do for patients. But, machine learning (ML) is changing the game, offering the promise of catching these diseases earlier and with more accuracy, wouldn’t that be something?
The Sheer Power of Machine Learning
ML algorithms? They’re brilliant at spotting patterns and subtle changes in massive datasets, things a human might just miss. Think of it like this: it’s like trying to find a single typo in a 500-page book; a human might struggle, but a computer can find it in seconds. When it comes to neurodegenerative diseases, these algorithms can sift through:
-
Neuroimaging Data: We’re talking MRI and PET scans. These give us detailed looks at the brain. ML can find tiny changes in brain volume, shape, and even how different areas connect – signs that might point to early disease.
-
Motor and Language Data: Believe it or not, simple things like analyzing handwriting samples or speech patterns can reveal subtle motor and language problems that are typical of diseases like Parkinson’s. I remember reading a study once where they could predict Parkinson’s onset years in advance, just from analyzing how people drew a spiral. Crazy, right?
-
Genetic Information: ML can crunch genetic data to find biomarkers and risk factors. This allows for early risk assessment and, potentially, personalized treatment.
-
Clinical Assessments: ML can pull together data from cognitive tests and motor evaluations to boost diagnostic accuracy and track how a disease is progressing. It’s like having a super-powered research assistant.
Different Flavors of Machine Learning
There are a few different techniques at play here:
-
Supervised Learning: Algorithms like Support Vector Machines (SVMs) and Random Forests are trained using data that’s already labeled. For example, images from patients who have the disease versus those who don’t. Then, they can classify new data. Simple, but effective.
-
Deep Learning: Deep learning models, especially Convolutional Neural Networks (CNNs), are excellent at analyzing complicated data like medical images. They automatically pull out the key features that show early signs of disease.
-
Ensemble Methods: Combining multiple ML models? That can improve diagnostic accuracy and make the whole system more robust. It’s like getting a second, third, and fourth opinion, all at once.
Real-World Impact and What’s Next?
Researchers have already used ML to tell the difference between healthy folks and those with diseases like Alzheimer’s and Parkinson’s. Some models are even hitting accuracy levels of over 95% for early detection – that’s way better than traditional methods, which are really impressive. This opens the door for:
-
Early Diagnosis and Intervention: Catching diseases early means we can intervene sooner, potentially slowing down or even stopping the progression of the disease. Imagine the impact that would have on patients’ lives.
-
Personalized Treatment: ML can help us tailor treatments to each patient, based on their specific situation and how the disease is progressing. The one-size-fits-all approach is on the way out.
-
Drug Discovery and Development: ML can speed up the process of finding potential drug targets and predicting whether new therapies will work. This could drastically shorten the timeline for getting new treatments to patients.
Challenges? Of Course. And Ethical Considerations!
It’s not all sunshine and roses, though. There are challenges to overcome:
-
Data Differences: Data can vary a lot from one study to another, and that can make it tough to develop ML models that are both robust and generalizable. It’s important to have consistent data standards.
-
Understanding the “Why?”: Being able to see how an ML model makes its predictions is key to building trust and getting clinicians to actually use it. It’s like trusting a mechanic – you want to know why they’re recommending a certain repair.
-
Ethical Concerns: Data privacy, biases in algorithms, and the responsible use of AI in healthcare, it’s all crucial to consider. We need to make sure we’re using these tools ethically and fairly.
Looking Ahead
Research is constantly moving forward, including integrating data from different sources (neuroimaging, genetics, clinical data, etc.), real-time monitoring for dynamic assessments, and, perhaps most importantly, building stronger connections between researchers, clinicians, and tech developers. As a result, ML has real potential to change how we diagnose and manage neurodegenerative diseases, leading to better outcomes and a brighter outlook for those affected. It’s an exciting time to be working in this field. I think we’re only just scratching the surface, you know?
Beyond diagnostic accuracy, could machine learning also predict the rate of disease progression for individual patients, informing proactive care plans and resource allocation?
That’s a great point! Absolutely, predicting the rate of disease progression is a key area. Imagine the power of tailoring interventions based on predicted disease trajectory. This would allow for resource allocation and personalized care, optimizing patient outcomes and quality of life. Thanks for raising this important consideration!
Editor: MedTechNews.Uk
Thank you to our Sponsor Esdebe
The discussion of data differences highlights a crucial point. Ensuring data standardization and diversity across datasets will be essential for developing machine learning models that are both accurate and applicable to broader patient populations.
That’s so true! The variability in datasets can definitely impact the reliability of ML models. Exploring federated learning approaches, where models are trained across multiple datasets without direct data sharing, could be a valuable avenue to address this challenge and improve generalizability. What are your thoughts on this?
Editor: MedTechNews.Uk
Thank you to our Sponsor Esdebe