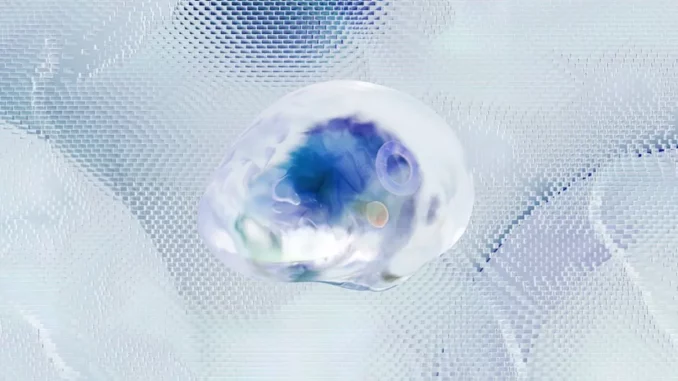
As I engaged in a conversation with Dr. Sarah Thompson, a passionate researcher at Kaunas University of Technology (KTU), her fervour for advancing mental health diagnostics was unmistakable. “Depression is one of the most prevalent mental health disorders, impacting as many as 280 million individuals globally,” she began, her voice a blend of resolve and optimism. “This is why our commitment to developing tools that can truly make a difference is so steadfast.”
Our dialogue revolved around KTU’s pioneering creation of an artificial intelligence (AI) model poised to revolutionise depression diagnosis. This groundbreaking model merges the analysis of speech and brain neural activity to deliver a more accurate and objective diagnosis. Dr. Thompson, who has played an integral role in the project’s development, elucidated the logic behind selecting these two data sources. “Traditional depression diagnostics heavily rely on subjective assessments, often limited to single data forms like self-reported questionnaires,” she observed. “By integrating speech and EEG data, our multimodal approach offers a more comprehensive view of an individual’s emotional state.”
The AI model, a result of collaborative efforts spearheaded by Professor Rytis Maskeliūnas and his team, boasts an impressive 97.53% accuracy in diagnosing depression. Dr. Thompson further explained the rationale behind the choice of speech as a data source. “Facial expressions, while informative, can sometimes be deceptive. Speech, however, captures subtle nuances in pace, intonation, and energy, often reflecting one’s mental state more accurately,” she stated. The meticulous process of data collection was designed to ensure both precision and privacy. EEG data, sourced from the Multimodal Open Dataset for Mental Disorder Analysis (MODMA), involved participants at rest with closed eyes to capture undisturbed brain activity. Concurrently, audio data was gathered through interactive sessions promoting natural language use, enabling the AI to discern underlying cognitive and emotional states.
A crucial feature of this model is its capacity to convert EEG and audio signals into spectrograms, which are then analysed by a modified DenseNet-121 deep-learning model. “This is the heart of the innovation,” Dr. Thompson remarked with a smile. “Spectrograms visually represent signal changes over time, allowing the AI to identify patterns indicative of depression.” Despite the promising achievements, Dr. Thompson recognised the forthcoming challenges. “The model’s ‘black box’ nature is a current limitation. Though it is accurate, it does not yet articulate its reasoning in a manner easily comprehensible to healthcare professionals,” she conceded. “This is where explainable AI (XAI) becomes essential. We are striving to make the AI’s decision-making process transparent, which will not only bolster trust in the system but also provide valuable insights for clinicians.”
Looking forward, Dr. Thompson expressed optimism about the potential for this AI model to not only expedite depression diagnosis but also facilitate remote access, thereby mitigating the reliance on subjective evaluations. Nonetheless, she cautioned that this vision requires extensive clinical trials and further enhancement of the programme. “A significant challenge is the scarcity of data,” she highlighted. “Individuals are often hesitant to share personal mental health information, which can hinder progress. We must devise strategies to encourage participation while guaranteeing privacy and security.”
As our discussion concluded, it was evident that Dr. Thompson and her team at KTU stand at the forefront of a potential revolution in mental health care. Their work shines as a beacon of hope for millions suffering from depression, heralding a future where diagnosis is not only swift and precise but also profoundly insightful. In this era of rapidly evolving technology, KTU’s research underscores the critical importance of harmonising AI with human-centric approaches. Through this integration, they are charting a course towards a more compassionate and effective mental health care system—one that acknowledges the intricate tapestry of human emotions and responds with both precision and empathy.
Be the first to comment