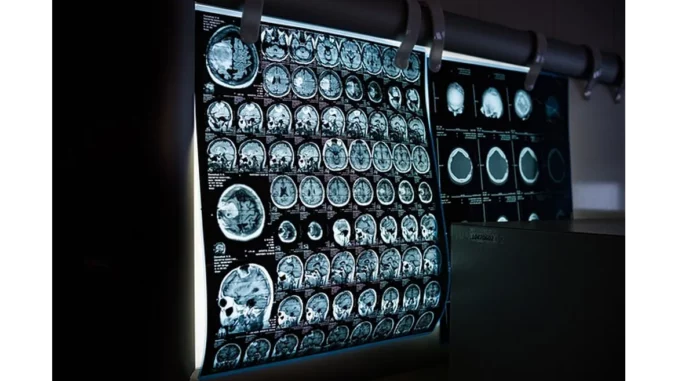
The emergence of artificial intelligence (AI) has transformed numerous sectors, with healthcare standing out as an area with immense potential. As AI technology advances, its role in medical diagnostics and decision-making has become a focal point of research and development. A noteworthy study by Ben-Gurion University of the Negev, published in Computers in Biology and Medicine, explores the capabilities of large language models (LLMs) such as ChatGPT-4 within the medical domain. The study reveals that although ChatGPT-4 surpasses other models, significant challenges remain unresolved.
AI models, particularly those utilising large language models like ChatGPT, have become increasingly integral to healthcare applications. These sophisticated models are employed in diverse roles, ranging from patient interaction through chatbots to predicting diseases, generating synthetic data for privacy protection, and assisting medical students with educational queries. The proficiency of AI in processing textual data and classifying information has shown promise across various scenarios. However, when it involves critical, life-saving clinical data, a more nuanced understanding of medical codes and concepts is imperative to ensure accuracy and reliability.
The study from Ben-Gurion University sought to evaluate how well LLMs comprehend the medical landscape and their capability to address pertinent questions. The research compared general-purpose models with those specifically fine-tuned for medical information. Researchers developed a rigorous evaluation method, generating over 800,000 closed questions and answers that span international medical concepts across three levels of complexity. These questions were crafted to test the models’ ability to interpret medical terminology and distinguish between various concepts such as diagnoses, procedures, and pharmaceuticals. The evaluation method incorporated existing clinical data standards, allowing for an assessment of clinical codes to differentiate medical concepts for tasks such as medical coding practice, summarisation, and automated billing.
The results of the study indicated that most models, even those trained on medical data, performed inadequately, often akin to random guessing. Notably, ChatGPT-4 emerged with an average accuracy of approximately 60%, outperforming its counterparts. Despite this relative success, the researchers noted that ChatGPT-4’s performance was still lacking for specific questions related to medical codes. The study’s findings suggest that while general-purpose models like ChatGPT-4 and Llama3-70B achieved superior results compared to clinical language models, their primary focus does not lie in medical applications. Notably, ChatGPT-4 showed an average improvement of 9-11% over Llama3-OpenBioLLM-70B, the clinical language model with the highest performance, indicating the potential for general-purpose models in healthcare settings.
The research underscores the crucial need for AI models that can accurately interpret medical codes and differentiate between medical concepts. Although ChatGPT-4 demonstrates potential, its limitations highlight the necessity for caution in its use for critical medical decision-making. The study establishes a benchmark for evaluating the quality of information related to medical codes, stressing the importance of ongoing improvements and broader evaluation of AI models in the healthcare sector.
The integration of AI into healthcare offers both significant opportunities and formidable challenges. As models like ChatGPT-4 continue to develop, their potential to advance medical diagnostics and decision-making processes is considerable. However, ensuring the precision and dependability of these models remains a paramount concern. This calls for sustained research and development, as well as collaboration among AI developers, healthcare professionals, and policymakers to address ethical considerations and optimise AI’s role in patient care.
Ultimately, while ChatGPT-4 outshines other models in medical AI comparisons, the path towards fully dependable AI-driven medical diagnostics is a work in progress. The insights from the Ben-Gurion University study provide valuable perspectives on the current state of AI in healthcare and chart a course for future advancements in this vital field. The findings highlight the importance of balancing innovation with caution, ensuring that AI’s integration into healthcare enhances patient outcomes while safeguarding against potential risks.
Be the first to comment