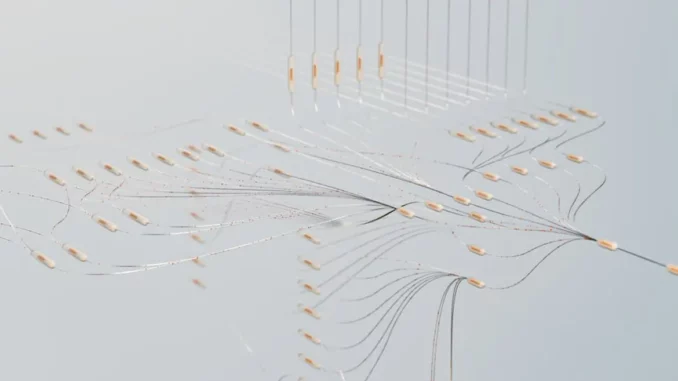
In the bustling ambience of a local café, I had the privilege of engaging in an enlightening discussion with Dr. Evelyn Carver, a distinguished independent researcher who has been meticulously examining the intersection of artificial intelligence with the health care sector. Our conversation centred around a transformative concept with vast potential for redefining patient care: Reinforcement Learning (RL).
Dr. Carver, known for her astute insights into AI trends, began our dialogue with palpable enthusiasm, referencing a series of recent studies she had been scrutinising. “Reinforcement Learning is far more than machines mastering games or surpassing human abilities,” she commented, her eyes alight with excitement. “It’s about machines evolving to make critical decisions—decisions that could very well save lives in the near future.”
The studies in question, conducted by researchers at Weill Cornell Medicine and Rockefeller University, explore the profound impact RL could have on health care. As Dr. Carver elaborated, RL is a sophisticated machine learning algorithm that learns through trial and error, akin to human learning processes. By assessing the results of various actions, it incrementally hones its decision-making abilities. “Envision a system capable of dynamically adjusting treatment plans based on a patient’s changing condition,” she explained. “This is the transformative potential RL offers.”
A particularly groundbreaking introduction from these studies is the concept of “Episodes of Care,” or EpiCare, which Dr. Carver described as a pivotal benchmark for testing RL in the medical field. “Benchmarks have been instrumental in advancing other AI domains such as image recognition and natural language processing,” she asserted, her tone earnest. “EpiCare could be the catalyst propelling RL forward in health care applications.”
While the prospects are promising, Dr. Carver was quick to highlight the hurdles that lie ahead. “The data demands for RL are formidable,” she noted. “In the studies, models were trained on tens of thousands of simulated treatment episodes. Implementing these models in real-world scenarios requires rigorous scrutiny.” Our discussion naturally shifted to the limitations of “off-policy evaluation” (OPE) methods, which utilise historical data to train RL models. The studies revealed deficiencies in these methods’ ability to accurately predict RL performance in medical contexts. “It underscores the complexity and subtlety of patient data,” Dr. Carver observed. “There is a pressing need for more sophisticated tools to evaluate and enhance RL algorithms.”
Throughout our conversation, I was struck by Dr. Carver’s balanced perspective. Despite recognising the challenges, she remained optimistic about the potential of RL. “Our objective,” she articulated, “is to develop RL systems robust enough to aid in creating personalised treatment strategies. Consider the impact on chronic or psychiatric care, where treatment is often an evolving journey.”
Beyond the realm of RL, Dr. Carver touched on innovations in other AI fields, such as adapting convolutional neural networks (CNNs) to work with graph-structured data. This research, she explained, holds significant promise for deciphering complex networks, such as those within the human brain. “Analysing data in its original graph form allows us to uncover patterns and relationships that were previously elusive,” she said, her enthusiasm evident.
The Quantized Graph Convolutional Networks (QuantNets) framework, developed in part by the same research team, aims to model these complex data structures. Dr. Carver elucidated how this could be applied to study EEG data in patients, offering insights into brain connectivity and its alterations during treatment. “This could revolutionise our understanding and treatment of conditions like depression or obsessive-compulsive disorder,” she added, highlighting the broad implications of this research.
As our conversation came to a close, Dr. Carver reflected on the path ahead for AI in health care. “We stand at a thrilling juncture,” she remarked. “Every new breakthrough, whether a novel algorithm or a new benchmark, brings us closer to more personalised and effective patient care. The journey is fraught with challenges, yet the potential rewards are extraordinary.”
As I departed the café, I found myself contemplating the future that Dr. Carver had vividly described—a future where AI not only assists but collaborates with medical professionals to optimise patient outcomes. In the ever-evolving landscape of health care, Reinforcement Learning emerges as a beacon of hope, heralding a future where personalised medicine transitions from aspiration to reality.
Be the first to comment