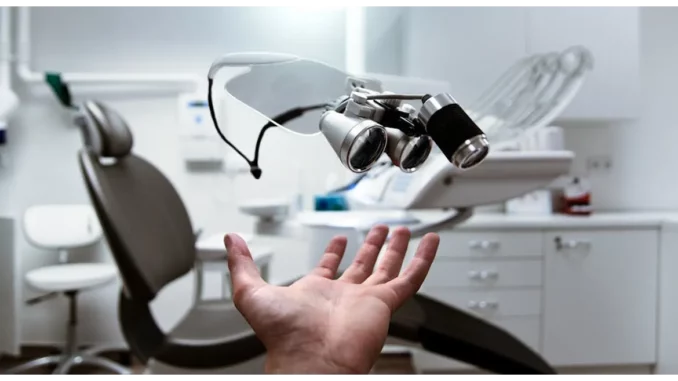
The introduction of large language models (LLMs), such as ChatGPT, into the healthcare sector heralds a new era of possibilities driven by advancements in artificial intelligence (AI). With the potential to transform various facets of medical practice—ranging from diagnostics to patient communication—LLMs promise to enhance clinical efficiency and effectiveness. However, alongside these potential benefits lies a spectrum of challenges that necessitate careful navigation. This exploration delves into the complex issues surrounding the use of LLMs in healthcare, advocating for a balanced approach that considers both their promising capabilities and their inherent limitations.
LLMs have captured the healthcare industry’s attention due to their ability to process vast amounts of text and generate human-like language, making them invaluable for managing medical documentation and streamlining clinical workflows. Their adeptness at mimicking human communication patterns allows for more natural interactions, potentially improving how healthcare is delivered. For instance, LLMs can aid in compiling patient notes, support clinical decision-making, and serve as educational tools for patients and medical professionals alike. Nevertheless, the deployment of these models requires a nuanced understanding of their capabilities and constraints.
A critical limitation of LLMs is their lack of genuine understanding of meaning or context. These models generate text based on patterns observed in extensive datasets, which can sometimes result in plausible but incorrect information. This shortcoming is particularly concerning in the medical field, where accuracy is paramount. The phenomenon known as “AI hallucinations”—where LLMs produce misleading or erroneous outputs—highlights the risks of relying too heavily on these models without human oversight. As a result, medical professionals must exercise caution and ensure that AI-generated information undergoes rigorous validation by human experts.
The ethical implications of LLM use in healthcare are profound, particularly concerning the risks of over-reliance and bias. These models are not infallible, and an over-dependence on their outputs, absent thorough vetting, could have serious repercussions. Furthermore, LLMs can perpetuate biases present in their training data, raising concerns about equity and fairness in healthcare delivery. To counteract these risks, several strategies can be implemented. Layered LLM architectures, for example, employ multiple models to cross-validate outputs and identify inaccuracies. While promising, this approach introduces additional complexity and the potential for error propagation. Integrating LLMs with trusted databases and verification systems is another strategy, ensuring AI-generated content is cross-referenced with credible sources.
Explainable AI (XAI) techniques offer a pathway to enhance transparency in AI decision-making, enabling users to grasp how LLMs generate their outputs. However, XAI does not address the core limitation of LLMs: their reliance on statistical patterns rather than genuine reasoning. Regulatory frameworks, such as the European Union’s AI Regulation and the US AI Bill of Rights Blueprint, have established crucial standards for transparency, safety, and accountability. Adapting LLMs to meet these standards is vital to ensuring their responsible use in healthcare. Moreover, some experts advocate exploring alternative AI paradigms, such as neurosymbolic AI, which combines neural networks with logical reasoning to address LLM limitations. Though promising, neurosymbolic AI also faces challenges in scalability and interpretability. Continuous research and innovation are required to develop AI systems that can effectively support healthcare delivery while maintaining ethical and safety standards.
As the healthcare industry stands on the cusp of an AI-driven transformation, the integration of LLMs presents both opportunities and challenges. These models have the potential to revolutionise medical practice by boosting efficiency and aiding decision-making processes. Yet, their limitations call for a cautious and critical approach. By prioritising transparency, accountability, and ethical considerations, stakeholders can work towards leveraging AI’s benefits while mitigating potential risks to patients. As AI technology continues to evolve, ongoing dialogue and collaboration among developers, healthcare professionals, and policymakers will be crucial in ensuring the responsible and beneficial use of LLMs in medicine. It is this collaborative spirit and balanced perspective that will ultimately guide the successful integration of AI into healthcare, safeguarding patient welfare while embracing innovation.
Be the first to comment