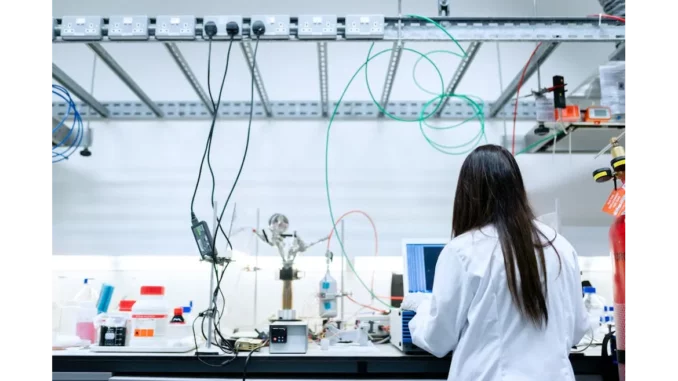
In the ever-evolving landscape of cancer research, the intersection of artificial intelligence (AI) and medical diagnostics heralds a transformative era. A prime exemplar of this is SEQUOIA, an innovative AI tool that forecasts cancer gene activity from biopsy images. This technological advancement is poised to refine the precision of cancer diagnostics, potentially revolutionising patient care and outcomes.
SEQUOIA, which stands for slide-based expression quantification using linearized attention, is an AI model that predicts the expression patterns of over 15,000 genes from stained biopsy images. It was developed using an extensive dataset comprising 7,584 cancer biopsies from 16 distinct cancer types. These samples were prepared using the standard hematoxylin and eosin staining, a well-established method in pathology for visualising cancerous cells. This visual data was augmented with transcriptomic information, providing a comprehensive view of the genes actively engaged by the cancer cells. The AI model exhibited extraordinary accuracy, demonstrating a correlation exceeding 80% between AI-predicted and actual gene activity data for certain types of cancer. This correlation improves with the inclusion of more samples from a given cancer type, underscoring the model’s adaptability and potential for further enhancement.
In a clinical context, SEQUOIA’s capacity to predict gene signatures, rather than individual gene expressions, offers significant advantages. Clinicians frequently depend on gene signatures—comprising clusters of hundreds of genes—to make well-informed decisions regarding treatment and prognosis. These signatures can signal processes such as inflammation or cell proliferation, which are vital in comprehending cancer progression. A particularly compelling application of SEQUOIA is in the realm of breast cancer. The model accurately predicted the expression of genes involved in commercial genomic tests, such as the FDA-approved MammaPrint test, which evaluates the risk of cancer recurrence. By relying solely on stained biopsy images, SEQUOIA suggests a future where expensive gene expression tests might be supplanted by AI-driven analysis, markedly reducing costs and enhancing accessibility.
Another noteworthy aspect of SEQUOIA is its novel approach to visualising genetic variations within tumours. It provides a visual representation of genetic findings as maps of tumour biopsies, enabling researchers and clinicians to comprehend how genetic variations differ across various tumour regions. This spatial understanding could pave the way for more targeted treatment strategies, addressing the heterogeneity commonly observed within tumours.
Despite its promise, SEQUOIA is not yet ready for immediate clinical application, as it necessitates further testing through clinical trials and regulatory endorsements. However, its potential applications extend beyond breast cancer. Lead researcher Gevaert envisions its utility across all cancer types, offering insights into any available gene signature. The development of SEQUOIA was bolstered by a collaboration of academic and industry partners, including Roche Diagnostics, and was funded by grants from multiple international organisations. This underscores the collective effort indispensable in translating such innovative tools from concept to clinical reality.
As SEQUOIA advances through clinical trials and navigates regulatory pathways, it holds the promise of reshaping cancer diagnostics, harnessing AI to predict gene activity from biopsy images. By potentially replacing costly gene expression tests with AI analysis, SEQUOIA could significantly enhance the accessibility and affordability of precision medicine. Thus, SEQUOIA represents a significant leap forward in cancer care, illustrating the profound impact of integrating AI into medical diagnostics and reinforcing the promise of a future where precision medicine is within reach for all.
Be the first to comment