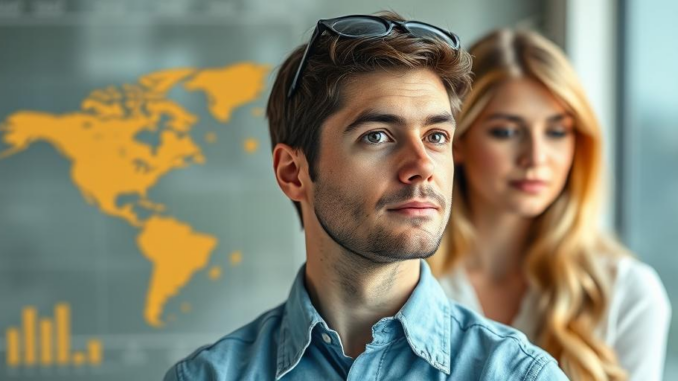
Abstract
Accurate and equitable premium pricing is the cornerstone of a sustainable insurance market. However, the confluence of demographic shifts, technological advancements, evolving regulatory landscapes, and inherent uncertainties in predicting future risks pose significant challenges to actuarial science. This research report provides a comprehensive analysis of the complexities involved in pricing insurance premiums across diverse sectors, examining traditional actuarial methodologies alongside emerging innovations. We delve into the intricacies of risk assessment, the impact of behavioral economics, the integration of big data and machine learning, and the ethical considerations that shape premium determination. Furthermore, we explore the implications of regulatory interventions and market dynamics on premium affordability and accessibility, ultimately proposing avenues for fostering more resilient and equitable insurance systems.
Many thanks to our sponsor Esdebe who helped us prepare this research report.
1. Introduction: The Critical Role of Premium Pricing
Insurance operates on the principle of pooling risk, where individuals or entities contribute premiums to a collective fund that compensates those who experience unforeseen losses. The premium, therefore, acts as the price of this risk transfer mechanism. The accuracy and fairness of premium pricing are paramount for the viability of both insurance companies and policyholders. Underpricing can lead to financial instability for insurers, potentially resulting in insolvency and leaving policyholders unprotected. Conversely, overpricing can deter individuals from purchasing insurance, creating adverse selection problems and undermining the insurance market’s effectiveness in mitigating societal risks. Beyond its financial implications, premium pricing also carries ethical considerations, particularly regarding access to essential insurance coverage for vulnerable populations. Therefore, a robust and transparent framework for premium determination is crucial for fostering trust and ensuring equitable access to risk management tools.
Many thanks to our sponsor Esdebe who helped us prepare this research report.
2. Foundational Actuarial Principles and Traditional Methodologies
The core of premium pricing lies in actuarial science, which utilizes mathematical and statistical methods to assess and manage financial risks. Traditional actuarial methodologies rely heavily on historical data, probability distributions, and statistical modeling to forecast future claim costs. The process typically involves several key steps:
- Data Collection and Analysis: Actuaries gather extensive data on past claims, policyholder characteristics, and environmental factors relevant to the risk being insured. This data is then analyzed to identify patterns and trends that can inform future predictions.
- Risk Classification: Policyholders are grouped into risk classes based on characteristics that are statistically correlated with the likelihood of claims. This allows insurers to charge premiums that reflect the relative risk associated with each group.
- Probability Modeling: Actuaries employ statistical models, such as Poisson, binomial, and normal distributions, to estimate the probability of future claims. These models are calibrated using historical data and adjusted for expected changes in the risk environment.
- Expense Loading: Insurers add a loading factor to the pure premium (the expected cost of claims) to cover administrative expenses, marketing costs, and profit margins. This loading factor is typically expressed as a percentage of the pure premium.
- Discounting for Investment Income: Insurers may discount future claim payments to reflect the investment income they expect to earn on premium funds before claims are paid.
While these traditional methodologies have proven effective in many contexts, they also face limitations. One key challenge is the reliance on historical data, which may not accurately reflect future risks, particularly in rapidly changing environments. Moreover, traditional models often assume that individuals are rational actors who make informed decisions, an assumption that behavioral economics has shown to be frequently violated.
Many thanks to our sponsor Esdebe who helped us prepare this research report.
3. The Influence of Behavioral Economics on Insurance Demand and Risk Assessment
Behavioral economics has revolutionized our understanding of human decision-making, revealing systematic biases and cognitive limitations that influence individual behavior. These insights have profound implications for insurance pricing and risk management. Several key behavioral biases are particularly relevant:
- Loss Aversion: Individuals tend to feel the pain of a loss more strongly than the pleasure of an equivalent gain. This can lead to underinsurance, as people may be reluctant to pay premiums for a potential future loss, even if the expected value of the coverage exceeds the cost.
- Availability Heuristic: Individuals tend to overestimate the probability of events that are easily recalled or vividly imagined. This can lead to overinsurance for certain types of risks, such as those that have recently been publicized in the media.
- Framing Effects: The way in which information is presented can significantly influence decision-making. For example, framing insurance as a protection against loss rather than a cost can increase demand.
- Present Bias: Individuals tend to place a disproportionate emphasis on immediate gratification, leading them to discount future benefits and costs. This can result in procrastination in purchasing insurance or engaging in preventive behaviors.
Recognizing these behavioral biases, insurers are increasingly incorporating behavioral insights into their pricing and marketing strategies. For example, they may use framing techniques to highlight the benefits of insurance, offer incentives for preventive behaviors, or design default options that encourage greater coverage. Furthermore, actuaries are exploring ways to incorporate behavioral factors into risk assessment models to better predict claim probabilities.
Many thanks to our sponsor Esdebe who helped us prepare this research report.
4. Leveraging Big Data and Machine Learning for Enhanced Premium Prediction
The proliferation of data and the advancement of machine learning (ML) techniques have opened up new possibilities for improving premium pricing accuracy. Big data refers to the vast volumes of structured and unstructured data generated by various sources, including insurance claims, policyholder records, wearable devices, social media, and internet of things (IoT) sensors. ML algorithms can analyze this data to identify complex patterns and relationships that are not readily apparent using traditional statistical methods. Several applications of big data and ML in premium pricing include:
- Improved Risk Segmentation: ML algorithms can identify more granular risk segments than traditional methods, allowing insurers to charge premiums that are more closely aligned with individual risk profiles. For instance, telematics data from cars can be used to assess driving behavior and adjust auto insurance premiums accordingly.
- Predictive Modeling of Claim Costs: ML models can be trained to predict future claim costs based on a wide range of variables, including policyholder characteristics, medical history, lifestyle factors, and environmental conditions. This can help insurers to better anticipate future liabilities and set premiums accordingly.
- Fraud Detection: ML algorithms can identify suspicious claims patterns and flag potential fraud cases for further investigation. This can help insurers to reduce losses from fraudulent claims and keep premiums lower for honest policyholders.
- Personalized Pricing: ML can enable insurers to offer personalized premiums based on individual risk profiles and preferences. This can increase customer satisfaction and loyalty, as policyholders feel that they are receiving a fair price for their coverage.
While the potential benefits of big data and ML in premium pricing are significant, there are also important challenges to address. Data privacy and security are paramount concerns, as insurers must ensure that they are collecting and using data in a responsible and ethical manner. Furthermore, ML models can be complex and opaque, making it difficult to understand the factors that are driving premium predictions. This can raise concerns about fairness and transparency.
Many thanks to our sponsor Esdebe who helped us prepare this research report.
5. Regulatory Frameworks and Their Impact on Premium Affordability and Accessibility
Government regulations play a crucial role in shaping insurance markets and influencing premium pricing. The primary goals of insurance regulation are to protect consumers, ensure the solvency of insurers, and promote fair competition. Regulations can impact premium pricing in several ways:
- Rate Regulation: Some jurisdictions impose direct rate regulation, which limits the amount that insurers can charge for premiums. This is often done to protect consumers from excessive pricing, particularly in essential insurance markets such as health insurance.
- Underwriting Restrictions: Regulations may restrict insurers’ ability to discriminate against certain groups of individuals based on factors such as age, gender, or pre-existing medical conditions. This can increase premium costs for some groups while lowering them for others.
- Mandated Coverage: Regulations may require insurers to offer certain types of coverage, such as mental health benefits or coverage for specific medical conditions. This can increase premium costs for all policyholders.
- Capital Requirements: Regulations require insurers to maintain a certain level of capital reserves to ensure their solvency. This can increase the cost of doing business for insurers, which may be passed on to consumers in the form of higher premiums.
Regulatory interventions can have both positive and negative effects on premium affordability and accessibility. While rate regulation can protect consumers from excessive pricing, it can also discourage insurers from offering coverage in certain markets, leading to reduced competition and limited consumer choice. Similarly, underwriting restrictions can promote fairness and equity, but they can also increase premium costs for certain groups.
The design of effective insurance regulation requires careful consideration of the trade-offs between consumer protection, insurer solvency, and market efficiency. Regulators must strike a balance between ensuring that insurance is affordable and accessible while also allowing insurers to earn a reasonable profit and remain financially sound.
Many thanks to our sponsor Esdebe who helped us prepare this research report.
6. Ethical Considerations in Premium Pricing
Premium pricing decisions are not solely based on technical calculations; they also involve significant ethical considerations. The principle of fairness is central to ethical premium pricing, but its interpretation can be complex. Several ethical dilemmas arise in this context:
- Adverse Selection and Cream Skimming: Insurers have an incentive to attract low-risk individuals while avoiding high-risk individuals. This can lead to adverse selection, where the insurance pool becomes dominated by high-risk individuals, driving up premiums for everyone. Cream skimming, the practice of selectively insuring low-risk individuals, is also ethically problematic, as it undermines the principle of risk sharing.
- Data Privacy and Discrimination: The use of big data and ML in premium pricing raises concerns about data privacy and the potential for discrimination. Insurers must ensure that they are collecting and using data in a responsible and ethical manner, and that they are not unfairly discriminating against individuals based on sensitive characteristics such as race, religion, or socioeconomic status.
- Transparency and Explainability: Policyholders have a right to understand how their premiums are determined. Insurers should be transparent about their pricing methodologies and provide clear explanations for any changes in premiums. The use of complex ML models can make it difficult to explain premium predictions, raising concerns about transparency and accountability.
- Access to Essential Coverage: Insurance plays a vital role in protecting individuals from financial hardship and promoting social well-being. Insurers have an ethical obligation to ensure that essential insurance coverage is accessible to all members of society, regardless of their risk profile or socioeconomic status.
Addressing these ethical challenges requires a multi-faceted approach. Insurers must adopt ethical codes of conduct, invest in data privacy and security measures, and promote transparency and explainability in their pricing practices. Regulators must establish clear guidelines for data usage and prevent discriminatory pricing practices. Society as a whole must engage in a dialogue about the role of insurance in promoting fairness and equity.
Many thanks to our sponsor Esdebe who helped us prepare this research report.
7. Emerging Trends and Future Directions in Premium Pricing
Several emerging trends are shaping the future of premium pricing:
- Personalized and Dynamic Pricing: Advances in technology are enabling insurers to offer more personalized and dynamic pricing based on individual risk profiles and real-time behavior. This can increase customer satisfaction and loyalty, but it also raises concerns about data privacy and the potential for unfair discrimination.
- Blockchain Technology: Blockchain technology can be used to improve transparency and efficiency in insurance transactions. It can also enable new forms of insurance, such as peer-to-peer insurance, which can lower premiums by eliminating intermediaries.
- Parametric Insurance: Parametric insurance provides coverage for specific events, such as earthquakes or floods, based on pre-defined parameters. This can simplify claims processing and reduce administrative costs, leading to lower premiums.
- Cyber Insurance: The growing threat of cyberattacks is driving demand for cyber insurance, which protects businesses from financial losses resulting from data breaches and other cyber incidents. Premium pricing for cyber insurance is particularly challenging due to the rapidly evolving nature of cyber threats.
- Climate Change: Climate change is increasing the frequency and severity of extreme weather events, posing significant challenges for the insurance industry. Insurers must adapt their pricing models to account for these increased risks, and they must also play a role in promoting climate resilience.
These emerging trends will require actuaries and regulators to adapt their skills and frameworks to ensure that insurance remains a sustainable and equitable risk management tool in the face of evolving challenges. Continuous research and innovation are essential for developing pricing models that are accurate, transparent, and ethical.
Many thanks to our sponsor Esdebe who helped us prepare this research report.
8. Conclusion
Accurate and equitable premium pricing is a complex and multifaceted challenge that requires a deep understanding of actuarial science, behavioral economics, data analytics, regulatory frameworks, and ethical considerations. While traditional actuarial methodologies provide a solid foundation for premium determination, they must be complemented by emerging innovations such as big data, machine learning, and behavioral insights. Regulatory interventions play a crucial role in shaping insurance markets and ensuring that insurance remains affordable and accessible. Ultimately, the goal of premium pricing should be to create a sustainable and equitable insurance system that protects individuals from financial hardship and promotes social well-being. This requires a collaborative effort among insurers, regulators, and policymakers to address the challenges and opportunities presented by a rapidly changing world.
Many thanks to our sponsor Esdebe who helped us prepare this research report.
References
- Arrow, K. J. (1963). Uncertainty and the Welfare Economics of Medical Care. The American Economic Review, 53(5), 941–973.
- Crocker, K. J., & Snow, A. (1985). The Efficiency Effects of Categorical Discrimination in Insurance Markets. The Journal of Political Economy, 93(2), 321–344.
- Eling, M., & Wirfs, J. H. (2016). What do we know about pricing in P&C insurance? Journal of Risk and Insurance, 83(4), 893-922.
- Freedman, D. A. (2009). Statistical models: Theory and practice. Cambridge University Press.
- Gollier, C. (2001). The Economics of Risk and Time. MIT Press.
- Kunreuther, H. (1996). Disaster insurance protection: Public policy lessons. John Wiley & Sons.
- Lemaire, J. (2013). Non-life insurance: Mathematics and statistics. Springer Science & Business Media.
- OECD (2018), FinTech and Insurance: Opportunities and Risks. OECD Publishing, Paris, DOI: https://doi.org/10.1787/9789264308149-en.
- Satz, D. (2010). Why Some Things Should Not Be For Sale: The Moral Limits of Markets. Oxford University Press.
- Tversky, A., & Kahneman, D. (1974). Judgment under uncertainty: Heuristics and biases. Science, 185(4157), 1124-1131.
The discussion of ethical considerations, especially regarding data privacy in premium pricing, is crucial. How can insurers effectively balance personalized pricing through data analytics with the need to protect sensitive customer information and avoid potential discrimination?
That’s a great point! The balance is definitely tricky. Perhaps a focus on anonymized data sets coupled with rigorous algorithmic audits could help insurers innovate with data while safeguarding individual privacy and preventing unintended biases. What are your thoughts on regulatory sandboxes for experimenting with these new pricing models?
Editor: MedTechNews.Uk
Thank you to our Sponsor Esdebe
So, personalized pricing, eh? I’m sure my data broker is thrilled. Wonder if insurers factor in my penchant for online shopping into my life insurance premiums. Or maybe my caffeine addiction impacts my health coverage costs. Inquiring minds (and wallets) want to know!
That’s a thought-provoking question! The potential for online shopping habits or caffeine consumption to influence premiums highlights the growing role of data in insurance. Exploring how insurers balance data usage with consumer privacy and ethical considerations is definitely crucial as personalized pricing evolves. It’s a fascinating and complex area to watch!
Editor: MedTechNews.Uk
Thank you to our Sponsor Esdebe