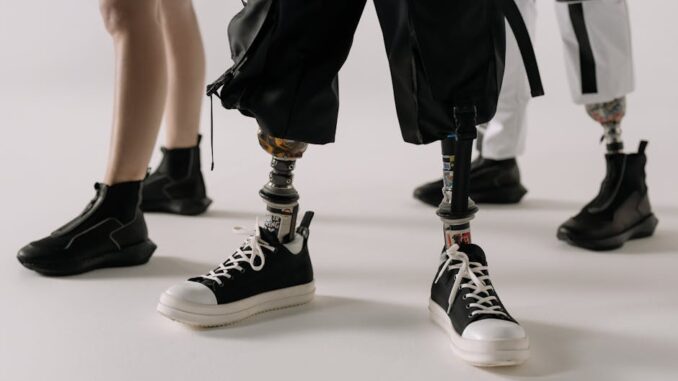
Abstract
Additive manufacturing (AM), also known as 3D printing, has revolutionized manufacturing across numerous industries, offering unprecedented design freedom, rapid prototyping capabilities, and the potential for mass customization. However, the widespread adoption of AM is hindered by challenges related to process control, material properties, and, critically, quality assurance. This research report provides a comprehensive overview of the current state of additive manufacturing, exploring key AM processes, materials, and applications. It delves into the inherent challenges associated with achieving consistent part quality, including issues related to porosity, residual stress, and dimensional accuracy. Existing non-destructive testing (NDT) methods employed in AM, such as ultrasonic testing, X-ray radiography, and computed tomography (CT), are critically examined, highlighting their strengths and limitations. The report then investigates the potential of advanced NDT techniques, including laser-driven X-ray CT and in-situ monitoring systems, to overcome these limitations and enhance quality control in AM. Finally, it explores future directions in AM, focusing on emerging materials, process optimization strategies, and the integration of artificial intelligence (AI) and machine learning (ML) to further improve process control, predict part performance, and ultimately drive the broader adoption of AM technologies. This report aims to provide a valuable resource for experts in the field, fostering a deeper understanding of the current landscape and future opportunities in additive manufacturing.
Many thanks to our sponsor Esdebe who helped us prepare this research report.
1. Introduction
Additive manufacturing (AM) represents a paradigm shift in manufacturing, moving away from subtractive (e.g., machining) and formative (e.g., casting) processes to a layer-by-layer material deposition approach [1]. This fundamental change unlocks unprecedented design freedom, enabling the creation of complex geometries and intricate internal features that are often impossible to produce using conventional manufacturing techniques. AM offers significant advantages, including reduced material waste, rapid prototyping capabilities, and the potential for mass customization. Consequently, AM has found applications in a diverse range of industries, including aerospace, automotive, biomedical, consumer goods, and energy [2].
Despite its potential, the widespread adoption of AM is constrained by several challenges. One of the most critical challenges is ensuring consistent part quality and reliability. Unlike traditional manufacturing processes, AM processes are often highly sensitive to variations in process parameters, material properties, and environmental conditions. These variations can lead to defects such as porosity, residual stress, dimensional inaccuracies, and variations in mechanical properties, which can significantly compromise the performance and integrity of AM-fabricated parts [3]. Addressing these challenges requires a comprehensive approach that encompasses process optimization, material development, and advanced quality control techniques.
This report aims to provide a comprehensive overview of the current state of additive manufacturing, focusing on the challenges associated with quality control and the potential of advanced non-destructive testing (NDT) methods to overcome these challenges. It will explore key AM processes, materials, and applications, critically examine existing NDT methods, and investigate the potential of emerging NDT techniques, such as laser-driven X-ray CT, to enhance quality control in AM. The report will also discuss future directions in AM, including emerging materials, process optimization strategies, and the integration of artificial intelligence (AI) and machine learning (ML) to further improve process control and predict part performance.
Many thanks to our sponsor Esdebe who helped us prepare this research report.
2. Additive Manufacturing Processes and Materials
AM encompasses a diverse range of processes, each with its own strengths, limitations, and suitability for specific applications. These processes can be broadly categorized based on the material deposition method employed [4]:
- Powder Bed Fusion (PBF): PBF processes utilize a powder bed as the feedstock material and employ a heat source, such as a laser (Selective Laser Melting/SLM, Selective Laser Sintering/SLS) or an electron beam (Electron Beam Melting/EBM), to selectively melt or sinter the powder particles together, layer by layer. PBF processes are capable of producing parts with high density and complex geometries and are widely used for metals, polymers, and ceramics [5].
- Directed Energy Deposition (DED): DED processes involve the simultaneous deposition of material and energy, typically in the form of a laser or electron beam, to melt or fuse the material as it is deposited. DED processes are often used for repairing existing parts, adding features to existing components, and creating large-scale parts. Common DED processes include Laser Engineered Net Shaping (LENS) and Wire Arc Additive Manufacturing (WAAM) [6].
- Material Extrusion: Material extrusion processes, such as Fused Deposition Modeling (FDM), involve extruding a thermoplastic filament through a heated nozzle and depositing it layer by layer to create a part. FDM is a relatively low-cost and versatile AM process widely used for prototyping and producing functional parts from polymers [7].
- Vat Photopolymerization: Vat photopolymerization processes, such as Stereolithography (SLA) and Digital Light Processing (DLP), utilize a liquid photopolymer resin that is selectively cured by exposure to a light source, such as a laser or projector. Vat photopolymerization processes are capable of producing parts with high resolution and smooth surfaces and are commonly used for producing prototypes and tooling [8].
- Material Jetting: Material jetting processes involve depositing droplets of liquid material, such as polymers or waxes, onto a build platform and curing or solidifying them to create a part. Material jetting processes can be used to produce multi-material parts with varying properties and colors [9].
- Binder Jetting: Binder jetting processes involve depositing a liquid binder onto a powder bed to selectively bind the powder particles together. After each layer is deposited, the build platform is lowered, and a new layer of powder is spread across the surface. Once the build is complete, the unbound powder is removed, and the parts are typically infiltrated with a secondary material to improve their strength and density [10].
The materials used in AM span a broad range, including metals, polymers, ceramics, and composites. The choice of material depends on the specific application requirements, such as strength, stiffness, temperature resistance, and biocompatibility [11]. Common AM materials include:
- Metals: Stainless steel, titanium alloys, aluminum alloys, nickel alloys, cobalt-chrome alloys, and precious metals.
- Polymers: Acrylonitrile Butadiene Styrene (ABS), Polycarbonate (PC), Polylactic Acid (PLA), Nylon (PA), Polyetheretherketone (PEEK), and Polyetherimide (PEI).
- Ceramics: Alumina, zirconia, silicon carbide, and silicon nitride.
- Composites: Polymer matrix composites reinforced with carbon fibers, glass fibers, or ceramic particles.
The selection of the appropriate AM process and material is crucial for achieving the desired part properties and performance. Careful consideration must be given to factors such as material compatibility, process parameters, and post-processing requirements.
Many thanks to our sponsor Esdebe who helped us prepare this research report.
3. Challenges in Additive Manufacturing Quality Control
Achieving consistent part quality remains a significant challenge in AM due to the complexity of the processes and the numerous factors that can influence the final product. Several key challenges must be addressed to ensure the widespread adoption of AM for critical applications [12]:
- Porosity: Porosity, or the presence of voids within the material, is a common defect in AM-fabricated parts, particularly in metal AM. Porosity can significantly reduce the mechanical properties, fatigue resistance, and corrosion resistance of the part. Causes of porosity include insufficient melting or sintering, gas entrapment, and lack of fusion between layers [13].
- Residual Stress: The rapid heating and cooling cycles inherent in many AM processes can induce significant residual stresses within the part. These residual stresses can lead to distortion, cracking, and premature failure. Residual stress management is crucial for ensuring the dimensional accuracy and structural integrity of AM-fabricated parts [14].
- Dimensional Accuracy: Achieving precise dimensional control is essential for many applications. However, AM processes can be prone to dimensional inaccuracies due to factors such as thermal expansion and contraction, shrinkage, and process variability. Accurate process calibration and optimization are necessary to minimize dimensional deviations [15].
- Surface Finish: The layer-by-layer nature of AM processes can result in a rough surface finish, which may be unacceptable for certain applications. Post-processing techniques, such as machining, polishing, or coating, may be required to improve the surface finish [16].
- Material Properties: The mechanical properties of AM-fabricated parts can vary significantly depending on the process parameters, material properties, and post-processing treatments. It is crucial to carefully control the process parameters to achieve the desired material properties [17].
- Process Variability: AM processes are often highly sensitive to variations in process parameters, material properties, and environmental conditions. This process variability can lead to inconsistent part quality and reliability. Robust process control and monitoring are essential for minimizing the impact of process variability [18].
- Lack of Standardization: The lack of standardized testing methods, materials, and process parameters makes it difficult to compare the performance of different AM processes and materials. Standardization efforts are needed to facilitate the widespread adoption of AM [19].
Overcoming these challenges requires a multi-faceted approach that encompasses process optimization, material development, advanced quality control techniques, and standardization efforts.
Many thanks to our sponsor Esdebe who helped us prepare this research report.
4. Existing Non-Destructive Testing (NDT) Methods in Additive Manufacturing
Non-destructive testing (NDT) methods play a crucial role in ensuring the quality and reliability of AM-fabricated parts. NDT methods allow for the detection of defects and the assessment of material properties without damaging or destroying the part [20]. Several NDT methods are commonly used in AM:
- Visual Inspection: Visual inspection is the simplest and most widely used NDT method. It involves visually examining the part for surface defects, such as cracks, porosity, and dimensional inaccuracies. Visual inspection can be enhanced using magnifying glasses, microscopes, or automated optical inspection systems [21].
- Dye Penetrant Inspection (DPI): DPI is a widely used NDT method for detecting surface-breaking defects in non-porous materials. The process involves applying a dye penetrant to the surface of the part, allowing it to penetrate into any surface cracks or pores, removing the excess penetrant, and then applying a developer that draws the penetrant back to the surface, revealing the location of the defects [22].
- Ultrasonic Testing (UT): UT uses high-frequency sound waves to detect internal defects and measure material thickness. Sound waves are transmitted into the part, and the reflected or transmitted waves are analyzed to identify defects. UT is sensitive to variations in material density and can be used to detect porosity, cracks, and inclusions [23].
- X-ray Radiography (RT): RT uses X-rays to create an image of the internal structure of the part. X-rays are transmitted through the part, and the amount of radiation that is absorbed depends on the density and thickness of the material. The resulting image reveals variations in density and can be used to detect porosity, cracks, and inclusions [24].
- Computed Tomography (CT): CT uses X-rays to create a three-dimensional image of the internal structure of the part. CT involves acquiring a series of X-ray images from different angles and then reconstructing the images using computer algorithms to create a 3D representation of the part. CT provides detailed information about the size, shape, and location of internal defects and can be used to assess the dimensional accuracy of the part [25].
- Thermography: Thermography uses infrared cameras to detect temperature variations on the surface of the part. These temperature variations can be indicative of internal defects, such as cracks or delaminations. Thermography can be used to assess the thermal conductivity and heat distribution within the part [26].
While these NDT methods are valuable for quality control in AM, they also have limitations. Visual inspection and DPI are limited to detecting surface defects. UT can be challenging to apply to parts with complex geometries. RT and CT can be time-consuming and expensive, and their resolution may be limited for detecting small defects. Furthermore, conventional X-ray CT systems often struggle with dense metallic parts due to X-ray attenuation, leading to long scan times and reduced image quality. Therefore, there is a need for advanced NDT techniques that can overcome these limitations and provide more comprehensive and efficient quality control in AM.
Many thanks to our sponsor Esdebe who helped us prepare this research report.
5. Advanced Non-Destructive Testing Techniques: Laser-Driven X-ray CT and In-Situ Monitoring
To address the limitations of existing NDT methods in AM, research is focused on developing advanced techniques that can provide more comprehensive and efficient quality control. Two promising approaches are laser-driven X-ray CT and in-situ monitoring [27].
-
Laser-Driven X-ray CT: Laser-driven X-ray sources offer several advantages over conventional X-ray tubes, including higher flux, smaller source size, and tunable energy. The higher flux allows for faster scan times and improved signal-to-noise ratio, while the smaller source size enables higher spatial resolution. The tunable energy allows for optimizing the X-ray energy for specific materials and defect types. Laser-driven X-ray CT has the potential to significantly improve the quality and efficiency of CT inspection for AM-fabricated parts, particularly for dense metallic components [28]. The higher energy X-rays are better able to penetrate dense materials, leading to clearer images and more accurate detection of internal flaws.
-
In-Situ Monitoring: In-situ monitoring involves integrating sensors and data acquisition systems directly into the AM process to monitor process parameters and material properties in real-time. In-situ monitoring can provide valuable information about the process conditions, such as temperature, melt pool size, and layer thickness, which can be used to detect and correct process deviations before they lead to defects. Common in-situ monitoring techniques include optical coherence tomography (OCT), acoustic emission monitoring, and thermal imaging [29]. The combination of in-situ monitoring with machine learning algorithms can enable closed-loop process control, where the process parameters are automatically adjusted based on the real-time monitoring data to maintain consistent part quality. This closed-loop control offers significant potential for improving the reliability and repeatability of AM processes.
-
Computed Tomography Metrology (CTM): CTM provides a non-destructive method for dimensional measurement and defect analysis of additively manufactured parts. It leverages the 3D data acquired through CT scanning to perform precise measurements, assess geometric tolerances, and identify deviations from the nominal design. [30] This technique is particularly valuable for complex geometries and internal features that are difficult to access with traditional metrology methods.
The integration of advanced NDT techniques, such as laser-driven X-ray CT and in-situ monitoring, has the potential to revolutionize quality control in AM, enabling the production of high-quality, reliable parts for critical applications.
Many thanks to our sponsor Esdebe who helped us prepare this research report.
6. The Role of Artificial Intelligence and Machine Learning
Artificial intelligence (AI) and machine learning (ML) are increasingly being integrated into AM processes to improve process control, predict part performance, and enhance quality assurance [31]. ML algorithms can be trained on large datasets of process parameters, material properties, and NDT data to identify correlations between process variables and part quality. This information can then be used to optimize process parameters, predict the likelihood of defects, and automate quality control procedures.
AI and ML can be applied to various aspects of AM, including:
- Process Optimization: ML algorithms can be used to optimize process parameters, such as laser power, scan speed, and layer thickness, to achieve the desired material properties and minimize defects [32].
- Defect Prediction: ML algorithms can be trained on NDT data to predict the likelihood of defects based on process parameters and material properties. This allows for proactive identification and correction of potential problems [33].
- Quality Control: AI-powered image analysis can be used to automate the inspection of AM-fabricated parts, detecting defects and dimensional inaccuracies with high accuracy and speed [34].
- Material Development: ML algorithms can be used to accelerate the discovery and development of new AM materials by predicting the properties of materials based on their composition and microstructure [35].
- In-situ Process Monitoring Analysis: Combining in-situ monitoring data with ML algorithms can provide real-time feedback on process stability and part quality, enabling adaptive process control and defect mitigation [36].
The integration of AI and ML into AM has the potential to significantly improve process control, reduce manufacturing costs, and enhance the reliability of AM-fabricated parts. However, the successful implementation of AI and ML in AM requires large datasets of high-quality data, robust algorithms, and skilled personnel.
Many thanks to our sponsor Esdebe who helped us prepare this research report.
7. Future Directions in Additive Manufacturing
The future of additive manufacturing is bright, with ongoing research and development efforts focused on addressing the remaining challenges and expanding the capabilities of AM technologies. Some key future directions in AM include:
- Emerging Materials: Research is focused on developing new AM materials with improved properties, such as higher strength, higher temperature resistance, and enhanced biocompatibility. Examples include high-entropy alloys, advanced ceramics, and bio-based polymers [37].
- Multi-Material Additive Manufacturing: The ability to produce parts with multiple materials and functionally graded properties is a key area of development. Multi-material AM enables the creation of parts with tailored properties and performance characteristics [38].
- Large-Scale Additive Manufacturing: Efforts are underway to scale up AM processes for producing large-scale parts, such as aircraft components and automotive body panels. This requires the development of new AM processes, materials, and equipment [39].
- Hybrid Manufacturing: Combining AM with traditional manufacturing processes, such as machining and casting, can leverage the strengths of both approaches to create parts with complex geometries and high precision [40].
- Sustainable Additive Manufacturing: Research is focused on developing more sustainable AM processes that reduce material waste, energy consumption, and environmental impact. This includes the use of recycled materials, energy-efficient processes, and closed-loop material management [41].
- Digital Twins and Predictive Modeling: Creating digital twins of AM processes and parts allows for simulating and predicting the performance of parts before they are manufactured. This can significantly reduce the time and cost associated with process development and quality control [42].
These future directions hold the promise of transforming manufacturing and enabling the creation of innovative products with enhanced performance, functionality, and sustainability.
Many thanks to our sponsor Esdebe who helped us prepare this research report.
8. Conclusion
Additive manufacturing has emerged as a transformative technology with the potential to revolutionize manufacturing across various industries. However, ensuring consistent part quality and reliability remains a critical challenge. This report has provided a comprehensive overview of the current state of additive manufacturing, exploring key AM processes, materials, and applications. It has critically examined existing NDT methods and investigated the potential of advanced NDT techniques, such as laser-driven X-ray CT and in-situ monitoring, to enhance quality control in AM. The report has also discussed the role of AI and ML in improving process control, predicting part performance, and enhancing quality assurance. By addressing the remaining challenges and continuing to innovate in materials, processes, and quality control techniques, additive manufacturing can realize its full potential and become a mainstream manufacturing technology.
Many thanks to our sponsor Esdebe who helped us prepare this research report.
References
[1] Gibson, I., Rosen, D. W., & Stucker, B. (2014). Additive manufacturing technologies: 3D printing, rapid prototyping, and direct manufacturing. Springer.
[2] Bourell, D., Rosen, D., Leu, M., & Seepersad, C. (2009). Roadmap for additive manufacturing: Identifying the future of freeform processing. University of Texas at Austin, Technical Report.
[3] DebRoy, T., Wei, H. L., Zuback, J. S., Cordova, J. M., Koduri, S., Wu, W., … & David, S. A. (2018). Additive manufacturing of metallic components–process, structure and properties. Progress in Materials Science, 92, 112-224.
[4] Wohlers, T. T., Campbell, R. I., Diegel, O., Kowen, J., & Shet, D. (2016). Wohlers Report 2016: 3D printing and additive manufacturing state of the industry. Wohlers Associates.
[5] Frazier, W. E. (2014). Metal additive manufacturing: A review. Journal of Materials Engineering and Performance, 23(6), 1917-1928.
[6] Carroll, B. E., Palmer, T. A., & Beese, A. M. (2015). Anisotropic mechanical behavior of Ti–6Al–4V structures fabricated with directed energy deposition additive manufacturing. Acta Materialia, 87, 309-320.
[7] Turner, B. N., Strong, R., & Gold, S. A. (2014). A review of melt extrusion additive manufacturing processes: I. Process design. Rapid Prototyping Journal, 20(3), 192-204.
[8] Melchels, F. P., Domingos, M. A., Stappers, L., Pereira, F. K., & Bartolo, P. J. (2012). Additive manufacturing of tissues and tissue scaffolds. Progress in Polymer Science, 37(8), 1079-1104.
[9] Guillemot, F., Souquet, A., Catros, S., Guillotin, B., & Bareille, R. (2010). Physics of laser-assisted bioprinting for bone tissue engineering. European Cells and Materials, 20, 284-293.
[10] Bai, X., Li, C., Rydholm, M., Liu, D., & Shen, J. (2016). Binder jetting of ceramics: A review. Journal of the European Ceramic Society, 36(1), 1-19.
[11] Attaran, M. (2016). An overview of powder-based additive manufacturing (AM) or 3D printing of metallic components. Additive Manufacturing, 11, 1-23.
[12] Ngo, T. D., Kashani, A., Imbalzano, G., Nguyen, K. T. Q., & Hui, D. (2018). Additive manufacturing (3D printing): A review of materials, methods, applications and challenges. Composites Part B: Engineering, 143, 172-196.
[13] King, W. E., Anderson, A. T., Ferencz, R. M., Hodge, N. E., Spadaccini, C. M., Weisberg, R. H., … & Rubenchik, A. M. (2015). Laser powder bed fusion additive manufacturing of pre-alloyed AlSi10Mg: Process optimization, microstructure and properties. Acta Materialia, 85, 252-262.
[14] Mukherjee, T., Manvatkar, V., De, A., & DebRoy, T. (2017). Mitigation strategies for residual stress and distortion during additive manufacturing. Annual Review of Materials Research, 47, 1-31.
[15] Song, Y. A., Tan, P. S., Bi, G., & Seah, L. K. (2017). Improving dimensional accuracy of SLM parts by minimizing thermal distortion. Journal of Materials Processing Technology, 241, 1-12.
[16] Kumar, S., Krolczyk, G. M., Chattopadhyaya, S., Kacalak, R., Zagorski, K., & Yadav, A. (2021). Strategies for surface roughness improvement of additively manufactured components. Materials, 14(17), 4801.
[17] Herzog, D., Seyda, V., Wycisk, E., & Emmelmann, C. (2016). Additive manufacturing of metals. Acta Materialia, 117, 371-392.
[18] Caulfield, B., McHugh, P. E., Lohfeld, J., & McLoone, S. (2016). Process monitoring of metal powder bed fusion additive manufacturing. Additive Manufacturing, 12, 1-17.
[19] Wegner, K., & Witt, G. (2015). Standardization in additive manufacturing: Status and research directions. Journal of Manufacturing Technology Management, 26(5), 739-757.
[20] Hellier, C. J. (2001). Handbook of nondestructive evaluation. McGraw-Hill.
[21] Almond, D., & Ruiz, A. (1993). Non-destructive testing. Chapman & Hall.
[22] Halmshaw, R. (1991). Non-destructive testing. Edward Arnold.
[23] Krautkrämer, J., & Krautkrämer, H. (2013). Ultrasonic testing: Inspection of welds. Springer Science & Business Media.
[24] Cullity, B. D., & Stock, S. R. (2001). Elements of X-ray diffraction. Prentice Hall.
[25] Stock, S. R. (2008). Computed tomography: Methodology, industrial applications and image analysis. CRC press.
[26] Maldague, X. P. (2001). Theory and practice of infrared technology for nondestructive testing. John Wiley & Sons.
[27] Thompson, A., & Miller, M. (2015). Developments in non-destructive testing. CRC Press.
[28] Barty, A., Liang, M., Marathe, S., Quiney, H. M., Nugent, K. A., & Paganin, D. (2008). Coherent diffraction microscopy with soft X-rays. Nature Photonics, 2(8), 472-476.
[29] Everton, S. K., Hirsch, M., Stravroulakis, P., Leandrou, E., & Taylor, S. (2016). Review of in-situ process monitoring and control for metal powder bed fusion. Materials & Design, 95, 431-445.
[30] Carmignato, S., Dewulf, W., Leach, R. K. (Eds.). (2018). Industrial X-Ray Computed Tomography. Springer.
[31] Agrawal, P., Zhao, C., Raina, A., Gao, R. X., & Lin, Z. (2020). Artificial intelligence in additive manufacturing: A survey. Additive Manufacturing, 36, 101530.
[32] Imani, A., Moradi, M., & Safarzadeh, H. (2021). Machine learning in additive manufacturing: A review. Journal of Intelligent Manufacturing, 32(3), 619-647.
[33] Scime, L., & Beese, A. M. (2019). Using machine learning to predict mechanical properties of additively manufactured materials. Materials & Design, 183, 108145.
[34] Baturynska, I., Holik, L., & Krawczyk, M. (2020). Artificial intelligence in the quality control of additive manufacturing processes. Procedia CIRP, 91, 600-605.
[35] Tahseen, A., Khan, A. M., Ullah, A., & Qureshi, I. M. (2021). Machine learning for material design and discovery. Applied Sciences, 11(12), 5408.
[36] Tapia, G., Elwany, A., & Sangid, M. D. (2016). Prediction of porosity in additively manufactured metallic parts using machine learning techniques. Journal of Manufacturing Science and Engineering, 138(6), 061007.
[37] Miracle, D. B., & Donaldson, S. L. (Eds.). (2001). Intermetallic compounds: synthesis, microstructure and properties. John Wiley & Sons.
[38] Zocca, A., Colombo, P., Gomes, C. M., & Günster, J. (2015). Additive manufacturing of ceramics: Issues, potentialities, and future trends. Journal of the American Ceramic Society, 98(7), 1983-2001.
[39] Rosen, D. W. (2007). Topologically structured material design for rapid manufactured products. Computer-Aided Design, 39(12), 997-1007.
[40] Mourtzis, D., Vlachou, E., & Milas, N. (2018). Hybrid manufacturing: A brief discussion of the recent advances and future directions. Procedia CIRP, 69, 411-416.
[41] Hopkinson, N., Dickens, P. M., & Hague, R. J. M. (2006). Rapid manufacturing: an industrial revolution for digital manufacturing. John Wiley & Sons.
[42] Grieves, M. (2014). Digital twin: Manufacturing excellence through virtual factory replication. In Complex systems engineering: Theory and practice (pp. 103-118).
Considering the crucial role of in-situ monitoring, how do you envision the integration of standardized sensor technologies and data analytics platforms across different AM processes to facilitate real-time feedback and ensure consistent quality control across varied manufacturing environments?
That’s a great point! Standardizing sensor tech and data platforms is key. I think a modular approach, allowing for adaptable integration with different AM processes, is vital. This would facilitate easier data sharing and analysis, leading to more consistent quality control across diverse manufacturing settings. What are your thoughts on the initial steps needed for this standardization?
Editor: MedTechNews.Uk
Thank you to our Sponsor Esdebe
The report highlights the critical need for advanced NDT techniques like laser-driven X-ray CT. How can we ensure these sophisticated methods are accessible and cost-effective for smaller manufacturing firms to facilitate broader adoption and quality improvements across the industry?
Great question! Perhaps a tiered service model could work, with larger firms investing in in-house laser-driven X-ray CT, while smaller firms access it through shared facilities or specialized service providers. This would distribute costs and ensure broader access to advanced NDT. What incentives or collaborative initiatives could encourage this shared resource approach?
Editor: MedTechNews.Uk
Thank you to our Sponsor Esdebe
The discussion of AI and ML integration for process optimization is particularly compelling. Exploring the potential of generative design algorithms to create novel geometries tailored for AM, considering material properties and process constraints, could further enhance design freedom and part performance.
I’m glad you found the AI/ML section compelling! Generative design is a fascinating area. Do you think integrating real-time feedback from in-situ monitoring could further refine these algorithms, leading to even more optimized designs and faster iteration cycles for AM parts?
Editor: MedTechNews.Uk
Thank you to our Sponsor Esdebe
The report highlights AI/ML’s role in optimizing process parameters. What level of explainability is currently achievable with these algorithms, and how might increased transparency impact adoption in industries with stringent certification requirements?
That’s an important point about explainability! Currently, some AI/ML models offer limited transparency. Increasing explainability through techniques like SHAP values or LIME is crucial, especially in regulated industries, where understanding the “why” behind decisions is essential for certification. It’s a trade-off between accuracy and interpretability that needs careful consideration. Do you have any thoughts on how to best communicate AI/ML decisions to regulators?
Editor: MedTechNews.Uk
Thank you to our Sponsor Esdebe