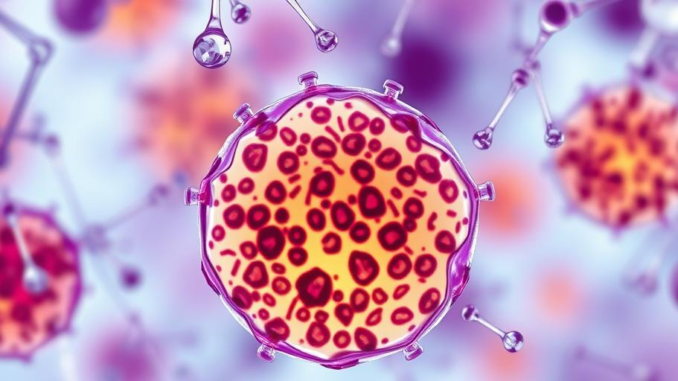
Advancements and Challenges in Biopsy Techniques: A Comprehensive Review with a Focus on AI Integration
Abstract
Biopsy, the extraction and microscopic examination of tissue samples, remains a cornerstone of modern medical diagnostics, particularly in oncology and the management of inflammatory and infectious diseases. While the principle is straightforward, the practice involves a diverse array of techniques, each with its own strengths, limitations, and associated risks. This review provides a comprehensive overview of contemporary biopsy techniques, ranging from traditional open surgical biopsies to minimally invasive approaches guided by advanced imaging modalities. It explores the success rates, complications, and evolving advancements in these techniques, with a specific focus on the burgeoning role of artificial intelligence (AI) in enhancing accuracy, efficiency, and patient outcomes. The discussion also addresses the inherent challenges in biopsy procedures, including sampling error, diagnostic discordance, and the need for standardized protocols, and proposes future directions for research and development in the field.
1. Introduction
Biopsy procedures are indispensable tools in the diagnostic armamentarium of modern medicine. They provide crucial information for distinguishing benign from malignant lesions, characterizing the stage and grade of tumors, identifying infectious agents, and assessing the extent of organ damage in inflammatory conditions. The evolution of biopsy techniques has been driven by the desire to minimize patient morbidity, improve diagnostic accuracy, and streamline the diagnostic workflow. From the early days of open surgical biopsies, the field has progressed to embrace minimally invasive approaches, image-guided interventions, and now, the integration of AI technologies.
The selection of the appropriate biopsy technique depends on a multitude of factors, including the location and size of the target lesion, the patient’s overall health status, the expertise of the medical team, and the availability of specialized equipment and imaging modalities. Each technique presents its own set of advantages and disadvantages, and the decision-making process often involves a careful balance of potential benefits and risks. This review aims to provide a comprehensive overview of these factors, highlighting the current state of the art in biopsy techniques and exploring the potential of AI to further improve their efficacy and safety.
2. Overview of Biopsy Techniques
Biopsy techniques can be broadly classified into several categories, including:
-
Excisional Biopsy: Involves the complete removal of a lesion. This technique is often used for superficial skin lesions, lymph nodes, and other easily accessible masses. It provides the most comprehensive histological information but is also the most invasive.
-
Incisional Biopsy: Involves the removal of a portion of a lesion. This technique is used when complete removal is not feasible or desirable, such as in large tumors or lesions in critical locations. The risk of sampling error is higher compared to excisional biopsy.
-
Core Needle Biopsy (CNB): Utilizes a large-bore needle to extract a cylindrical core of tissue. CNB is frequently employed for sampling deep-seated lesions in organs such as the liver, lung, and kidney. It provides sufficient tissue for histological analysis and avoids the need for open surgery.
-
Fine-Needle Aspiration (FNA): Involves the use of a small-gauge needle to aspirate cells and fluid from a lesion. FNA is a minimally invasive technique that is often used for sampling thyroid nodules, lymph nodes, and cysts. While less invasive, the tissue sample is limited, and accurate diagnosis requires skilled cytopathologic interpretation.
-
Endoscopic Biopsy: Performed during endoscopic procedures, such as colonoscopy, bronchoscopy, and upper endoscopy. Endoscopic biopsies allow for direct visualization of the target tissue and enable the sampling of lesions in the gastrointestinal tract, respiratory system, and other internal organs. This is particularly useful when combined with narrow-band imaging (NBI) or chromoendoscopy which can highlight suspicious areas for biopsy.
-
Image-Guided Biopsy: Utilizes imaging modalities such as ultrasound, computed tomography (CT), and magnetic resonance imaging (MRI) to guide the biopsy needle to the target lesion. Image guidance improves the accuracy of biopsy procedures, especially for small or deep-seated lesions. Real-time imaging further increases accuracy.
-
Liquid Biopsy: An emerging technique that involves analyzing circulating tumor cells (CTCs), circulating tumor DNA (ctDNA), or other biomarkers in blood or other bodily fluids. Liquid biopsies offer the potential for non-invasive cancer detection, monitoring, and prognostication, but their clinical utility is still being investigated. This is particularly useful for monitoring response to treatment and detecting minimal residual disease.
3. Success Rates and Risks Associated with Biopsy Procedures
While biopsy procedures are generally safe and effective, they are not without risks. The success rates and risks associated with each technique vary depending on the location and size of the target lesion, the patient’s health status, and the experience of the medical team.
Common complications of biopsy procedures include bleeding, infection, pain, and pneumothorax (in lung biopsies). In rare cases, more serious complications such as nerve damage, organ perforation, and tumor seeding can occur. Careful patient selection, meticulous technique, and appropriate post-procedure monitoring can help to minimize these risks.
The diagnostic accuracy of biopsy procedures is also influenced by several factors, including the size and quality of the tissue sample, the presence of sampling error, and the experience of the pathologist. Sampling error, which occurs when the biopsy sample does not accurately represent the entire lesion, is a major source of false-negative results. Techniques such as image-guided biopsy and multiple biopsies can help to reduce the risk of sampling error.
4. Advancements in Minimally Invasive and Robotic-Assisted Biopsy
Minimally invasive biopsy techniques have revolutionized the field of diagnostics by reducing patient morbidity, shortening recovery times, and improving cosmetic outcomes. Laparoscopic, thoracoscopic, and robotic-assisted biopsy procedures allow for the sampling of deep-seated lesions with minimal disruption to surrounding tissues. These techniques offer several advantages over traditional open surgical biopsies, including smaller incisions, less pain, and a lower risk of complications.
Robotic-assisted biopsy is a particularly promising area of development. Robotic systems provide surgeons with enhanced dexterity, precision, and visualization, enabling them to perform complex biopsy procedures with greater accuracy and control. Robotic platforms, such as the da Vinci Surgical System, have been used to perform biopsies of the lung, liver, pancreas, and other organs. Studies have shown that robotic-assisted biopsy can improve diagnostic yield, reduce the need for repeat biopsies, and shorten hospital stays.
5. The Role of Imaging in Guiding Biopsies
Imaging plays a critical role in guiding biopsy procedures, particularly for small or deep-seated lesions that are difficult to access without imaging guidance. Ultrasound, CT, and MRI are the most commonly used imaging modalities for guiding biopsies. These techniques provide real-time visualization of the target lesion and surrounding structures, allowing the physician to accurately guide the biopsy needle to the desired location.
-
Ultrasound-guided biopsy: Is a cost-effective and readily available technique that is often used for sampling superficial lesions in the breast, thyroid, and lymph nodes. Ultrasound provides real-time visualization of the needle and allows for dynamic adjustments during the procedure. However, ultrasound has limited penetration and may not be suitable for sampling deep-seated lesions.
-
CT-guided biopsy: Provides excellent anatomical detail and is particularly useful for sampling lesions in the lung, liver, and abdomen. CT guidance allows for precise needle placement and can be used to sample lesions that are difficult to visualize with other imaging modalities. However, CT involves exposure to ionizing radiation and may not be suitable for patients with contraindications to CT scanning.
-
MRI-guided biopsy: Offers superior soft tissue contrast and is particularly useful for sampling lesions in the brain, breast, and musculoskeletal system. MRI guidance allows for accurate needle placement and can be used to sample lesions that are not well-visualized with other imaging modalities. However, MRI is more expensive and time-consuming than ultrasound or CT, and it may not be available in all settings.
The integration of advanced imaging techniques, such as contrast-enhanced ultrasound, diffusion-weighted MRI, and positron emission tomography (PET)/CT, can further improve the accuracy of image-guided biopsies by providing additional information about the biological characteristics of the target lesion.
6. The Impact of Early Diagnosis on Patient Outcomes
Early diagnosis is crucial for improving patient outcomes in many diseases, particularly cancer. Biopsy procedures play a vital role in enabling early diagnosis by providing definitive evidence of disease and allowing for timely initiation of treatment. Studies have shown that early diagnosis of cancer can lead to improved survival rates, reduced morbidity, and lower healthcare costs.
For example, in lung cancer, early detection through screening programs and prompt biopsy of suspicious lesions can significantly improve survival rates. Similarly, in breast cancer, early diagnosis through mammography screening and biopsy of suspicious breast masses can lead to less aggressive treatments and better outcomes.
However, it is important to recognize that early diagnosis is not always beneficial. In some cases, early detection may lead to overdiagnosis and overtreatment, which can result in unnecessary anxiety, morbidity, and healthcare costs. Therefore, it is essential to carefully weigh the potential benefits and risks of early diagnosis in each individual case.
7. The Role of Artificial Intelligence in Biopsy Procedures
The integration of artificial intelligence (AI) is poised to transform biopsy procedures in several ways, including:
-
Image Analysis: AI algorithms can be trained to analyze medical images (CT, MRI, ultrasound) to identify suspicious lesions, segment tumors, and guide biopsy needles to the optimal location. AI-powered image analysis tools can improve the accuracy and efficiency of image-guided biopsies, reducing the risk of sampling error and improving diagnostic yield.
-
Real-time Guidance: AI algorithms can be used to provide real-time guidance during biopsy procedures, helping physicians to navigate complex anatomy, avoid critical structures, and optimize needle placement. AI-powered guidance systems can be particularly useful for robotic-assisted biopsies, where precise needle control is essential.
-
Pathology Analysis: AI algorithms can be trained to analyze histological images of biopsy samples to identify cancer cells, grade tumors, and predict prognosis. AI-powered pathology analysis tools can improve the accuracy and speed of pathological diagnosis, reducing the burden on pathologists and improving patient care. This is particularly important given the current shortage of pathologists in many countries.
-
Risk Prediction: AI algorithms can be used to predict the risk of complications associated with biopsy procedures, allowing physicians to tailor the procedure to the individual patient and minimize potential risks. AI-powered risk prediction models can be trained on large datasets of patient information, including demographic data, medical history, and imaging findings.
-
Personalized Medicine: AI can integrate data from various sources (imaging, genomics, clinical history) to predict which patients are most likely to benefit from biopsy. This is crucial to avoid unnecessary invasive procedures.
However, the integration of AI into biopsy procedures also presents several challenges. These include the need for large, high-quality datasets to train AI algorithms, the risk of bias in AI models, and the need for regulatory oversight to ensure the safety and effectiveness of AI-powered biopsy tools. Furthermore, the ethical implications of AI-assisted diagnostics, particularly regarding patient autonomy and data privacy, must be carefully considered.
8. Challenges and Future Directions
Despite the significant advancements in biopsy techniques, several challenges remain.
-
Sampling Error: As mentioned earlier, sampling error is a major source of false-negative results in biopsy procedures. Future research should focus on developing strategies to reduce the risk of sampling error, such as improved image guidance, multiple biopsies, and the use of advanced imaging techniques to identify the most representative area of the lesion.
-
Diagnostic Discordance: Discrepancies between biopsy results and final diagnoses can occur due to a variety of factors, including sampling error, interobserver variability among pathologists, and the presence of heterogeneous disease within the lesion. Future research should focus on developing standardized diagnostic criteria and improving communication between radiologists, surgeons, and pathologists.
-
Standardization of Protocols: There is a need for standardized protocols for biopsy procedures, including patient preparation, technique selection, image guidance, and post-procedure monitoring. Standardized protocols can help to improve the consistency and reliability of biopsy procedures and reduce the risk of complications.
-
Cost-Effectiveness: The cost of biopsy procedures can be a significant barrier to access, particularly in developing countries. Future research should focus on developing cost-effective biopsy techniques that can be widely implemented without compromising diagnostic accuracy or patient safety.
-
Integration of Multi-omics Data: The integration of genomic, proteomic, and metabolomic data with imaging and clinical data has the potential to provide a more comprehensive understanding of disease and improve diagnostic accuracy. Future research should focus on developing methods for integrating multi-omics data into biopsy procedures.
The future of biopsy procedures is likely to be characterized by further advancements in minimally invasive techniques, the integration of AI, and the development of personalized approaches to diagnosis and treatment. By addressing the challenges outlined above, we can continue to improve the safety, efficacy, and accessibility of biopsy procedures and ultimately improve patient outcomes.
9. Conclusion
Biopsy techniques are essential diagnostic tools that have evolved significantly over time. From traditional surgical approaches to minimally invasive and image-guided procedures, the field continues to advance. The integration of AI holds immense promise for enhancing accuracy, efficiency, and personalization in biopsy practices. However, challenges such as sampling error, diagnostic discordance, and the need for standardized protocols must be addressed to optimize the clinical utility of these techniques. Continued research and development in this area are crucial to improving patient outcomes and advancing the field of diagnostic medicine.
References
- [1] Jemal, A., et al. “Global cancer statistics.” CA: a cancer journal for clinicians 61.2 (2011): 69-90.
- [2] Kumar, V., Abbas, A. K., & Aster, J. C. (2017). Robbins and Cotran pathologic basis of disease. Philadelphia, PA: Elsevier.
- [3] Wahidi, M. M., et al. “American College of Chest Physicians consensus statement on the role of transbronchial needle aspiration in the diagnosis and staging of patients with suspected lung cancer.” Chest 129.6 (2006): 1749-1762.
- [4] Seigel C, Gatsonis C, Ransohoff DF, et al. Screening for Lung Cancer: Systematic Evidence Review to Update the 2004 U.S. Preventive Services Task Force Recommendation. Rockville (MD): Agency for Healthcare Research and Quality (US); 2013 Feb. Report No.: 11-05156-EF-1.
- [5] Esteva, A., Kuprel, B., Novoa, R. A., Ko, J., Swani, S. M., Blau, H. M., … & Threlfall, C. J. (2017). Dermatologist-level classification of skin cancer with deep neural networks. Nature, 542(7639), 115-118.
- [6] Coudray, N., Ocampo, P. S., Sakellaropoulos, T., Narula, N., Yadav, S. S., Gusev, A., … & Tsirigos, A. (2018). Classification and mutation prediction from non–small cell lung cancer histopathology using deep learning. Nature medicine, 24(10), 1559-1567.
- [7] Liu, H., Balagurunathan, Y., Garcia, A. L., Grimm, L. J., Christe, A., Currie, G., … & Gillies, R. J. (2017). Radiomic features for decoding tumor phenotype by unsupervised machine learning. Scientific reports, 7(1), 44261.
- [8] Hosny, A., Parmar, C., Quackenbush, J., Schwartz, L. H., & Aerts, H. J. W. L. (2016). Radiomics: principles and applications. European radiology, 26(11), 3524-3545.
- [9] Paschke, R., Hegedüs, L., Alexander, E., Valderrabano, P., Baloch, Z. W., Braun, D., … & Dralle, H. (2017). 2017 European Thyroid Association guidelines for the management of benign thyroid nodules. European thyroid journal, 6(3), 125-151.
- [10] Ke, G., Meng, Q., Finley, T., Wang, T., Chen, W., Ma, W., … & Liu, T. Y. (2017). Lightgbm: A highly efficient gradient boosting decision tree. In Advances in neural information processing systems (pp. 3146-3154).
- [11] Yu, K. H., Beam, A. L., & Kohane, I. S. (2018). Artificial intelligence in healthcare. Nature biomedical engineering, 2(10), 719-731.
- [12] Beaulieu-Jones, B. K., Moore, J. H., Williams, S. A., Havulinna, A. S., & Anttila, V. (2024). Ethical considerations of predictive algorithms in healthcare. BMC medical ethics, 25(1), 28.
- [13] van Griethuysen, J. J. M., Fedorov, A., Parmar, C., Hosny, A., Aucoin, N., Narayan, V., … & Aerts, H. J. W. L. (2017). Computational radiomics system to decode the radiographic phenotype. Cancer research, 77(21), e104-e107.
- [14] Tang, L. Y., Wu, D., & Peng, Y. (2019). Artificial intelligence in the diagnosis and treatment of lung cancer. Translational lung cancer research, 8(S1), S16.
- [15] National Lung Screening Trial Research Team. Reduced lung-cancer mortality with low-dose computed tomographic screening. N Engl J Med. 2011 Aug 4;365(5):395-409.
The discussion of AI integration in pathology analysis is particularly compelling, given the current shortage of pathologists. How might AI-driven tools assist in triaging cases to ensure the most critical samples are prioritized for immediate review?
That’s a great point! AI could definitely help triage cases. Imagine AI tools flagging high-risk features in digital pathology slides, allowing pathologists to focus on the most urgent cases first. It could really improve workflow efficiency and ensure timely diagnosis for those who need it most. What are your thoughts on the biggest hurdle to implementing this?
Editor: MedTechNews.Uk
Thank you to our Sponsor Esdebe
The overview of biopsy techniques is comprehensive. Considering liquid biopsies, how do you see the integration of AI improving the analysis of circulating biomarkers to personalize treatment strategies, and what validation studies are needed to ensure clinical reliability?
Thanks for your insightful question! AI’s role in analyzing circulating biomarkers from liquid biopsies is exciting. AI can identify subtle patterns indicative of treatment response or resistance, enabling real-time adjustments to therapy. Robust, multi-center clinical trials are essential to validate the accuracy and clinical utility of these AI-driven tools, ensuring consistent and reliable results across diverse patient populations. What are the potential regulatory pathways for AI-driven diagnostics?
Editor: MedTechNews.Uk
Thank you to our Sponsor Esdebe
The point about AI integrating multi-omics data to determine biopsy necessity is interesting. How do you see the cost-effectiveness of integrating these complex datasets impacting accessibility, particularly in resource-limited settings, and what strategies can mitigate these challenges?