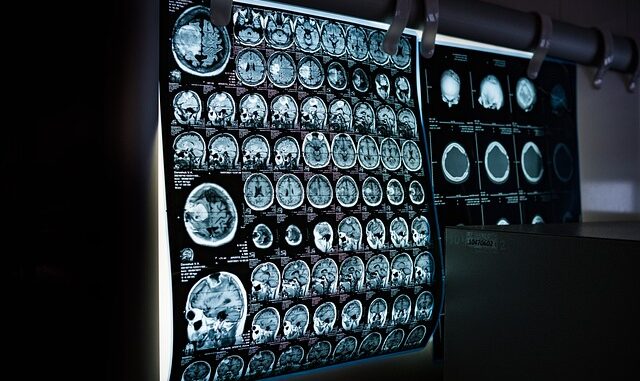
Abstract
Medical imaging has revolutionized healthcare, enabling non-invasive visualization and characterization of internal structures and processes. This review provides a comprehensive overview of advancements and challenges across various medical imaging modalities, including X-ray, computed tomography (CT), magnetic resonance imaging (MRI), ultrasound (US), and nuclear medicine techniques like positron emission tomography (PET) and single-photon emission computed tomography (SPECT). We delve into the principles of each modality, highlighting their strengths and limitations in different clinical applications. Recent innovations such as advanced image reconstruction algorithms, multi-parametric imaging, artificial intelligence (AI)-driven image analysis, and molecular imaging probes are discussed. Furthermore, we address the ongoing challenges related to radiation exposure, image quality, cost-effectiveness, and the need for standardized protocols and data sharing. Finally, we explore the potential of emerging imaging technologies and their role in precision medicine and personalized healthcare.
Many thanks to our sponsor Esdebe who helped us prepare this research report.
1. Introduction
Medical imaging has fundamentally transformed the practice of medicine, providing clinicians with invaluable tools for diagnosis, treatment planning, and monitoring disease progression. From the initial discovery of X-rays by Wilhelm Conrad Röntgen in 1895 to the development of sophisticated modalities like MRI and PET, medical imaging has continuously evolved, driven by technological advancements and a growing understanding of human physiology. This report aims to provide a detailed overview of the current state-of-the-art in medical imaging, covering both established techniques and emerging innovations. We will explore the underlying principles, clinical applications, strengths, and limitations of various imaging modalities, as well as the challenges and future directions in this rapidly evolving field.
The importance of medical imaging extends beyond simply visualizing anatomical structures. Modern imaging techniques are increasingly capable of providing functional and molecular information, allowing for earlier and more accurate diagnosis of diseases. The integration of advanced image processing algorithms and AI is further enhancing the capabilities of medical imaging, enabling quantitative analysis, automated detection of abnormalities, and personalized treatment planning. However, the increasing complexity and cost of these technologies also present significant challenges, particularly in terms of accessibility, standardization, and the need for well-trained personnel to interpret and utilize the vast amount of data generated.
Many thanks to our sponsor Esdebe who helped us prepare this research report.
2. Overview of Major Medical Imaging Modalities
2.1 X-ray Radiography
X-ray radiography is the oldest and most widely used medical imaging modality. It utilizes ionizing radiation to produce images of the body’s internal structures based on differential absorption of X-rays by different tissues. Dense tissues like bone absorb more X-rays and appear white on the image, while air-filled spaces appear black. X-ray radiography is commonly used for detecting fractures, pneumonia, and other abnormalities in the chest and skeletal system. However, its limited soft tissue contrast and the use of ionizing radiation are significant drawbacks.
2.2 Computed Tomography (CT)
CT is an advanced X-ray imaging technique that provides cross-sectional images of the body. It involves rotating an X-ray tube around the patient and acquiring multiple projections, which are then reconstructed using sophisticated algorithms to create a 3D image. CT offers superior soft tissue contrast compared to conventional X-ray radiography and is particularly useful for imaging the brain, chest, abdomen, and pelvis. Multi-detector CT scanners can acquire images rapidly, allowing for dynamic studies and angiography. However, CT also involves higher radiation doses compared to X-ray radiography, which is a concern, especially for pediatric patients. Newer techniques, such as iterative reconstruction algorithms, are being developed to reduce radiation dose without compromising image quality. Dual-energy CT can also provide additional information about tissue composition.
2.3 Magnetic Resonance Imaging (MRI)
MRI is a non-ionizing imaging technique that utilizes strong magnetic fields and radiofrequency pulses to generate images of the body. It is based on the principle of nuclear magnetic resonance (NMR), where atomic nuclei in a strong magnetic field absorb and emit radiofrequency energy. Different tissues have different NMR properties, which allow for excellent soft tissue contrast. MRI is particularly well-suited for imaging the brain, spinal cord, joints, and soft tissues. Different MRI sequences, such as T1-weighted, T2-weighted, and diffusion-weighted imaging (DWI), provide complementary information about tissue characteristics. Functional MRI (fMRI) can measure brain activity by detecting changes in blood flow. However, MRI is more expensive than CT and has longer scan times. Patients with metallic implants or pacemakers may not be able to undergo MRI. The spatial resolution of MRI can be limited by the signal-to-noise ratio, and artifacts can be problematic. Further improvements in gradient coils and radiofrequency technology are continually being made to increase the speed and resolution of MRI.
2.4 Ultrasound (US)
Ultrasound is a real-time imaging technique that uses high-frequency sound waves to create images of the body. A transducer emits sound waves that are reflected back from different tissues. The time it takes for the echoes to return and their amplitude are used to create an image. Ultrasound is commonly used for imaging the abdomen, pelvis, heart, and blood vessels. It is also widely used for prenatal imaging. Ultrasound is relatively inexpensive, portable, and does not involve ionizing radiation. However, image quality can be affected by the presence of air or bone, and the operator’s skill is crucial for obtaining diagnostic images. Contrast-enhanced ultrasound (CEUS) can improve image quality and provide information about blood flow. Emerging techniques like elastography can assess tissue stiffness, which can be useful for diagnosing liver fibrosis and other conditions. High-intensity focused ultrasound (HIFU) is also being developed for therapeutic applications, such as tumor ablation.
2.5 Nuclear Medicine (PET and SPECT)
Nuclear medicine techniques, such as PET and SPECT, use radioactive tracers to image physiological processes in the body. These tracers are injected into the patient and accumulate in specific organs or tissues. PET uses positron-emitting tracers, while SPECT uses gamma-emitting tracers. The radiation emitted by the tracers is detected by specialized cameras, which are used to create images of tracer distribution. PET is particularly useful for imaging cancer, heart disease, and neurological disorders. SPECT is commonly used for imaging the thyroid, bone, and heart. Nuclear medicine techniques provide functional and molecular information that is not available with other imaging modalities. However, they involve the use of ionizing radiation and have relatively low spatial resolution. The development of new and more specific radiotracers is a major area of research. Combined PET/CT and SPECT/CT scanners provide both anatomical and functional information.
Many thanks to our sponsor Esdebe who helped us prepare this research report.
3. Recent Advancements in Medical Imaging
3.1 Advanced Image Reconstruction Algorithms
Image reconstruction algorithms play a crucial role in converting raw data acquired by imaging scanners into diagnostic images. Traditional reconstruction algorithms, such as filtered back-projection, can be computationally efficient but may produce images with artifacts or noise. Advanced reconstruction algorithms, such as iterative reconstruction and model-based reconstruction, can significantly improve image quality by reducing noise, artifacts, and radiation dose. These algorithms use statistical models of the imaging process to iteratively refine the image until it matches the acquired data. Deep learning techniques are also being used to develop even more advanced image reconstruction algorithms that can further improve image quality and reduce reconstruction time.
3.2 Multi-Parametric Imaging
Multi-parametric imaging involves acquiring multiple images with different parameters to provide complementary information about tissue characteristics. For example, multi-parametric MRI can include T1-weighted, T2-weighted, diffusion-weighted, and perfusion-weighted imaging. By combining information from these different sequences, clinicians can obtain a more comprehensive understanding of the disease process. Multi-parametric imaging is particularly useful for characterizing tumors, assessing treatment response, and differentiating between different types of tissue. Radiomics is a field that involves extracting quantitative features from multi-parametric images to develop predictive models for diagnosis, prognosis, and treatment response.
3.3 Artificial Intelligence (AI)-Driven Image Analysis
AI, particularly deep learning, is revolutionizing medical image analysis. Deep learning algorithms can be trained to automatically detect and classify abnormalities in medical images, segment organs and tissues, and predict disease outcomes. AI-powered tools can assist radiologists in interpreting images, reducing the workload and improving diagnostic accuracy. AI can also be used to personalize treatment planning by predicting how a patient will respond to different therapies based on their imaging data. However, the development and validation of AI algorithms for medical imaging require large datasets and careful attention to bias and generalizability. Regulatory approval and ethical considerations are also important factors in the widespread adoption of AI in clinical practice. The accuracy and reliability of AI algorithms are critical for ensuring patient safety.
3.4 Molecular Imaging Probes
Molecular imaging probes are molecules that are designed to bind to specific targets in the body and can be detected by imaging modalities. These probes can be used to image specific biomarkers associated with disease, such as receptors, enzymes, and proteins. Molecular imaging probes are particularly useful for detecting early stages of disease, monitoring treatment response, and personalizing therapy. For example, PET tracers that target the prostate-specific membrane antigen (PSMA) are being used to image prostate cancer. The development of new and more specific molecular imaging probes is a major area of research. The use of nanotechnology to deliver imaging probes to specific targets is also being explored.
Many thanks to our sponsor Esdebe who helped us prepare this research report.
4. Challenges in Medical Imaging
4.1 Radiation Exposure
The use of ionizing radiation in X-ray, CT, and nuclear medicine poses a risk of radiation-induced cancer. Although the risk from a single imaging examination is generally low, the cumulative effect of multiple examinations can be significant. Efforts are being made to reduce radiation dose in medical imaging by optimizing imaging protocols, using advanced reconstruction algorithms, and developing alternative imaging techniques that do not involve ionizing radiation. The “As Low As Reasonably Achievable” (ALARA) principle is a guiding principle in radiation protection. Education and training of healthcare professionals and patients are also important for minimizing radiation exposure.
4.2 Image Quality
Image quality is crucial for accurate diagnosis and treatment planning. Factors that can affect image quality include noise, artifacts, resolution, and contrast. Noise can obscure fine details and make it difficult to detect abnormalities. Artifacts can mimic pathology and lead to misdiagnosis. Low resolution can limit the ability to visualize small structures. Poor contrast can make it difficult to differentiate between different tissues. Efforts are being made to improve image quality by optimizing imaging protocols, using advanced reconstruction algorithms, and developing new imaging technologies with higher sensitivity and resolution. Standardized imaging protocols and quality control procedures are also important for ensuring consistent image quality.
4.3 Cost-Effectiveness
The cost of medical imaging can be a significant barrier to access, particularly in developing countries. Advanced imaging modalities like MRI and PET are more expensive than conventional techniques like X-ray and ultrasound. Cost-effectiveness analyses are needed to determine the optimal use of medical imaging in different clinical scenarios. Efforts are being made to reduce the cost of medical imaging by developing cheaper imaging technologies, optimizing imaging protocols, and promoting the use of appropriate imaging guidelines. Sharing of imaging data and infrastructure can also help to reduce costs.
4.4 Standardization and Data Sharing
The lack of standardization in medical imaging protocols and data formats can hinder research and collaboration. Different institutions may use different imaging protocols, which can make it difficult to compare results across studies. The lack of standardized data formats can make it difficult to share imaging data between institutions. Efforts are being made to promote standardization in medical imaging by developing common data elements (CDEs) and imaging biomarkers. The development of open-source software and data repositories can also facilitate data sharing and collaboration.
Many thanks to our sponsor Esdebe who helped us prepare this research report.
5. Emerging Imaging Technologies
5.1 Photoacoustic Imaging (PAI)
Photoacoustic imaging (PAI) is a hybrid imaging technique that combines the high contrast of optical imaging with the deep penetration of ultrasound imaging. PAI uses pulsed laser light to generate ultrasound waves from tissues. The amplitude of the ultrasound waves is proportional to the optical absorption of the tissue. PAI can be used to image blood vessels, tumors, and other tissues with high optical absorption. It has potential applications in cancer diagnosis, cardiovascular imaging, and dermatology. The technique is relatively new, but is rapidly advancing with the introduction of handheld photoacoustic scanners.
5.2 Magnetic Particle Imaging (MPI)
Magnetic particle imaging (MPI) is a novel imaging technique that uses superparamagnetic iron oxide nanoparticles (SPIOs) as tracers. MPI detects the magnetic signal from the SPIOs in a strong magnetic field. It offers high sensitivity and specificity for imaging blood vessels, tumors, and other tissues. MPI is particularly well-suited for imaging blood flow and perfusion. It is also being explored for therapeutic applications, such as drug delivery and hyperthermia. MPI does not involve ionizing radiation, so it has potential advantages over X-ray and nuclear medicine techniques.
5.3 Terahertz Imaging
Terahertz imaging uses electromagnetic radiation in the terahertz frequency range (0.1-10 THz) to create images of the body. Terahertz radiation is non-ionizing and can penetrate many materials, including clothing, paper, and plastic. Terahertz imaging has potential applications in dermatology, dentistry, and security screening. It can also be used to detect cancer and other diseases. However, terahertz imaging is still a relatively new technology, and further research is needed to optimize its performance and expand its applications.
Many thanks to our sponsor Esdebe who helped us prepare this research report.
6. Impact on Precision Medicine and Personalized Healthcare
Medical imaging plays a crucial role in precision medicine and personalized healthcare by providing detailed information about individual patients’ anatomy, physiology, and molecular characteristics. Imaging can be used to identify patients who are likely to benefit from specific treatments, predict treatment response, and monitor disease progression. AI-powered image analysis can further enhance the capabilities of medical imaging in personalized healthcare by providing quantitative assessments of disease burden, predicting prognosis, and tailoring treatment plans to individual patients. The integration of medical imaging data with other clinical and genomic data can provide a more comprehensive understanding of the patient’s condition and guide personalized treatment decisions.
Many thanks to our sponsor Esdebe who helped us prepare this research report.
7. Conclusion
Medical imaging has made tremendous progress in recent decades, providing clinicians with powerful tools for diagnosis, treatment planning, and monitoring disease progression. Advances in image reconstruction algorithms, multi-parametric imaging, AI-driven image analysis, and molecular imaging probes are further enhancing the capabilities of medical imaging. However, challenges related to radiation exposure, image quality, cost-effectiveness, and standardization remain. Emerging imaging technologies like photoacoustic imaging, magnetic particle imaging, and terahertz imaging hold promise for future applications. The integration of medical imaging with other clinical and genomic data is essential for realizing the full potential of precision medicine and personalized healthcare. Continued research and development are needed to overcome the existing challenges and unlock the full potential of medical imaging to improve patient outcomes.
Many thanks to our sponsor Esdebe who helped us prepare this research report.
References
- Amunts, K., Lepage, Y., Borgeat, L., Mohlberg, H., Dickscheid, T., Rousseau, M. É., … & Zilles, K. (2013). BigBrain: an ultrahigh-resolution 3D human brain model. Science, 340(6139), 1472-1475.
- Brady, A. P., Crowe, P. M., Saeed, M. A. B., & Kavanagh, P. V. (2012). Computed tomography and radiation dose: what do radiologists need to know?. Clinical radiology, 67(2), 113-124.
- Esteva, A., Kuprel, B., Novoa, R. A., Ko, J., Swani, S. M., Blau, H. M., … & Threlfall, C. J. (2017). Dermatologist-level classification of skin cancer with deep neural networks. Nature, 542(7639), 115-118.
- Hamarneh, G., Jafari, M. H., Haghighi, M. S., & Rohling, R. (2020). AI in Medical Imaging. IEEE Pulse, 11(3), 22-26.
- Hricak, H., Gatsonis, C., Chiorean, E. G., Weatherall, J., Macari, M., Carter, D., … & Sandler, H. (2011). Prostate-specific antigen-detected prostate cancer: is early detection an independent risk factor for treatment failure and death?. Journal of the National Cancer Institute, 103(11), 892-899.
- Jaffe, T. A., & ожиданно, Б. С. (2019). Medical imaging at a crossroads: A narrative review. Journal of the American College of Radiology, 16(2), 205-210.
- Levin, D. L., Rao, V. M., & Parker, L. (2014). Radiation exposure in pediatric CT: is there a role for dose-reduction software?. American Journal of Roentgenology, 202(1), 1-3.
- Martens, J. W., Nieboer, D., Ruizendaal, L., Schroder, F. H., van der Kwast, T. H., & Bangma, C. H. (2016). Prostate cancer imaging with gallium-68-PSMA PET/CT: a systematic review. European urology, 69(5), 873-882.
- Moonen, C. T., Despres, D., Golay, X., Hyacinthe, J. N., de Certaines, J., & Grenier, N. (1990). Functional magnetic resonance imaging of the human brain. Magnetic resonance in medicine, 14(3), 550-554.
- Narayanan, D. L., & Eskey, C. J. (2013). Ultrasound contrast agents: an update. Clinical imaging, 37(3), 488-498.
- Rudin, S., & Bednarek, D. R. (2017). A brief history of X-ray tubes and related components. Journal of applied clinical medical physics, 18(1), 20-33.
- Szczepankiewicz, F., Nilsson, M., Topgaard, D., Westin, C. F., & Knutsson, H. (2015). Mapping compartment-specific diffusion kurtosis. Magnetic resonance in medicine, 73(1), 226-236.
- Weinmann, H. J., Gombas, B. B., Vogler, H., Viertel, U., & Radue, E. W. (1984). Contrast enhancement in MRI by Gd-DTPA. American Journal of Roentgenology, 142(3), 619-624.
Molecular imaging probes targeting specific biomarkers? Sounds like something straight out of science fiction! Imagine the possibilities for early disease detection. Are we on the cusp of personalized medicine revolution, or are there still significant hurdles to overcome before this becomes mainstream?
That’s a great point! The potential for early detection is truly exciting. While molecular imaging probes hold immense promise, challenges like probe specificity and cost-effectiveness need addressing before widespread clinical adoption. Overcoming these hurdles will be key to realizing the personalized medicine revolution. Your comment extends that discussion perfectly!
Editor: MedTechNews.Uk
Thank you to our Sponsor Esdebe
The discussion on AI-driven image analysis is compelling. The potential for deep learning to improve diagnostic accuracy and personalize treatment is significant, but ensuring algorithm reliability and addressing ethical concerns will be crucial for successful clinical integration.
Thanks for highlighting the importance of reliability and ethics in AI image analysis! It’s true that ensuring responsible algorithm development is paramount. We need robust validation frameworks and ongoing monitoring to build trust and prevent unintended consequences as we integrate these powerful tools into clinical workflows. The point about trust is really something to consider!
Editor: MedTechNews.Uk
Thank you to our Sponsor Esdebe