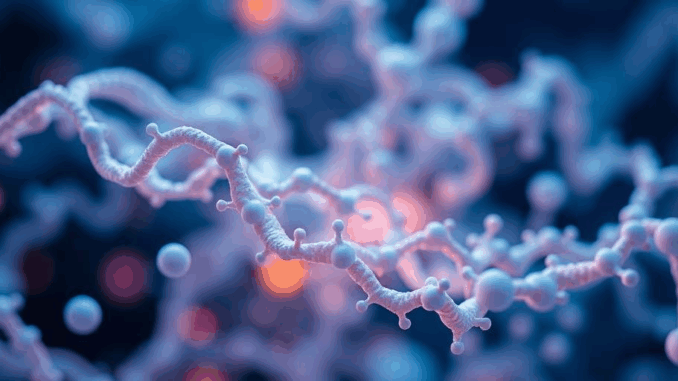
Abstract
Proteomics, the large-scale study of proteins, has emerged as a pivotal field in biological and medical research. Its ability to analyze the proteome – the entire set of proteins expressed by an organism or system – provides a functional readout that complements and extends genomic and transcriptomic information. This report offers a comprehensive review of proteomics, encompassing its fundamental principles, diverse techniques, and wide-ranging applications. We delve into established methodologies such as two-dimensional gel electrophoresis, mass spectrometry, and protein microarrays, as well as cutting-edge technologies like affinity proteomics, single-cell proteomics, and advanced mass spectrometry imaging. Furthermore, we explore the significant impact of proteomics in various domains, including biomarker discovery, drug target identification, personalized medicine, and systems biology. The report also critically examines the inherent challenges associated with proteomics research, such as sample complexity, dynamic range limitations, and data analysis bottlenecks. Finally, we discuss the future directions of proteomics, focusing on emerging technologies, the integration of multi-omics data, and the potential for personalized healthcare.
Many thanks to our sponsor Esdebe who helped us prepare this research report.
1. Introduction
The post-genomic era has witnessed a paradigm shift in biological sciences, with a growing recognition that genes are merely blueprints and that the functional molecules of life are proteins. Proteomics, defined as the comprehensive analysis of proteins, plays a crucial role in deciphering the complexity of cellular processes. Unlike genomics, which provides a static view of genetic potential, proteomics offers a dynamic snapshot of protein expression, modification, and interactions. This dynamic nature of the proteome reflects the influence of various factors, including developmental stage, environmental stimuli, and disease states. Therefore, proteomics provides valuable insights into biological pathways and disease mechanisms that are not accessible through genomics alone.
The applications of proteomics are diverse and far-reaching. In drug discovery, proteomics helps identify novel drug targets and predict drug efficacy. In personalized medicine, proteomic biomarkers can stratify patients based on their disease subtypes and predict their responses to treatment. In systems biology, proteomics contributes to the construction of comprehensive models of cellular function. Despite the immense potential of proteomics, several challenges must be addressed to fully realize its capabilities. These challenges include the complexity of the proteome, the limited sensitivity of some techniques, and the lack of standardized workflows.
Many thanks to our sponsor Esdebe who helped us prepare this research report.
2. Proteomics Techniques: A Comprehensive Overview
2.1. Sample Preparation
Effective sample preparation is paramount in proteomics research. This crucial step involves isolating and purifying proteins from biological samples, removing interfering substances, and ensuring that the proteins are compatible with downstream analytical techniques. A myriad of sample preparation methods exist, each tailored to specific sample types and research objectives. These methods include:
- Cell Lysis: Disrupting cell membranes to release intracellular proteins, achieved through mechanical methods (e.g., sonication, homogenization) or chemical methods (e.g., detergents, chaotropic agents).
- Protein Extraction: Separating proteins from other biomolecules, such as lipids, nucleic acids, and carbohydrates. Common extraction methods include precipitation, liquid-liquid extraction, and solid-phase extraction.
- Protein Fractionation: Reducing sample complexity by separating proteins based on physical or chemical properties, such as size, charge, or hydrophobicity. Fractionation techniques include gel electrophoresis, chromatography, and ultracentrifugation.
- Protein Digestion: Enzymatically cleaving proteins into smaller peptides, typically using trypsin. This step is essential for mass spectrometry-based proteomics, as peptides are more readily analyzed than intact proteins.
The selection of appropriate sample preparation techniques depends on several factors, including the nature of the biological sample, the abundance of target proteins, and the requirements of downstream analytical methods. Furthermore, it’s critical to minimize protein degradation, modification, and contamination during sample preparation to ensure accurate and reliable proteomic analyses. Advances in microfluidics and automation are streamlining sample preparation, improving reproducibility, and reducing sample handling errors.
2.2. Separation Techniques
Separation techniques are essential for resolving the complexity of proteomic samples. These techniques separate proteins or peptides based on their physical and chemical properties, allowing for the analysis of individual components. The two most common separation techniques in proteomics are:
- Two-Dimensional Gel Electrophoresis (2D-PAGE): This technique separates proteins based on their isoelectric point (pI) in the first dimension and their molecular weight in the second dimension. 2D-PAGE can resolve thousands of proteins in a single gel, making it a powerful tool for visualizing complex proteomes. However, 2D-PAGE is labor-intensive, has limited sensitivity, and is not well-suited for hydrophobic proteins.
- Liquid Chromatography (LC): This technique separates peptides or proteins based on their affinity for a stationary phase. LC is highly versatile and can be coupled to various detectors, including mass spectrometers. Different LC modes exist, including reversed-phase LC (RPLC), size-exclusion LC (SEC), and ion-exchange LC (IEX). RPLC is the most widely used LC mode in proteomics due to its high resolution and compatibility with mass spectrometry.
2.3. Mass Spectrometry (MS)
Mass spectrometry (MS) is the cornerstone of modern proteomics. MS measures the mass-to-charge ratio (m/z) of ions, providing information about the identity and abundance of proteins. Several MS platforms are used in proteomics, each with its own strengths and limitations. The most common MS platforms are:
- Matrix-Assisted Laser Desorption/Ionization Time-of-Flight (MALDI-TOF) MS: This technique involves embedding peptides or proteins in a matrix, which is then ionized by a laser. The ions are accelerated through a time-of-flight tube, and their m/z is determined based on their flight time. MALDI-TOF MS is commonly used for analyzing intact proteins and identifying post-translational modifications.
- Electrospray Ionization (ESI) MS: This technique involves spraying a solution of peptides or proteins into a fine mist, which is then ionized by an electric field. ESI MS is typically coupled to LC, allowing for the analysis of complex mixtures of peptides. Different types of mass analyzers can be coupled to ESI sources, including quadrupole, time-of-flight, and Orbitrap mass analyzers.
MS-based proteomics can be performed using two main approaches:
- Bottom-Up Proteomics: This approach involves digesting proteins into peptides prior to MS analysis. The resulting peptide fragments are then identified and quantified using MS/MS sequencing. Bottom-up proteomics is the most widely used approach in proteomics due to its high sensitivity and ability to identify a large number of proteins.
- Top-Down Proteomics: This approach involves analyzing intact proteins without prior digestion. Top-down proteomics provides information about protein isoforms and post-translational modifications that are lost in bottom-up proteomics. However, top-down proteomics is technically challenging and requires specialized instrumentation.
2.4. Protein Microarrays
Protein microarrays are high-throughput platforms for analyzing protein expression, interactions, and modifications. Protein microarrays consist of thousands of antibodies, proteins, or peptides immobilized on a solid support. These arrays can be used to detect and quantify proteins in complex samples.
There are two main types of protein microarrays:
- Antibody Microarrays: These arrays use antibodies to capture and detect specific proteins. Antibody microarrays are commonly used for measuring protein expression levels.
- Reverse-Phase Protein Microarrays (RPPAs): These arrays involve spotting lysates onto the array. Antibodies are then used to probe the array and determine the amount of protein present.
Protein microarrays offer several advantages over traditional methods, including high throughput, low sample consumption, and the ability to analyze multiple proteins simultaneously. However, protein microarrays are limited by the availability of high-quality antibodies and the potential for cross-reactivity.
2.5. Affinity Proteomics
Affinity proteomics utilizes specific binding reagents, such as antibodies or aptamers, to isolate and enrich target proteins from complex samples. This approach is particularly useful for studying low-abundance proteins or protein complexes. Affinity proteomics can be combined with mass spectrometry to identify and quantify interacting proteins or to characterize post-translational modifications.
2.6. Single-Cell Proteomics
Single-cell proteomics has emerged as a powerful approach for studying cellular heterogeneity. This technique allows for the analysis of protein expression in individual cells, providing insights into cell-to-cell variability and cellular responses to stimuli. Single-cell proteomics is technically challenging due to the small amount of protein available in each cell. However, recent advances in microfluidics and mass spectrometry have enabled the development of sensitive and robust single-cell proteomics platforms. These technologies are revolutionizing our understanding of cellular biology and disease.
Many thanks to our sponsor Esdebe who helped us prepare this research report.
3. Applications of Proteomics
3.1. Biomarker Discovery
Proteomics plays a crucial role in biomarker discovery, identifying proteins that can serve as indicators of disease or treatment response. By comparing the proteomes of healthy and diseased individuals, researchers can identify proteins that are differentially expressed in disease states. These proteins can then be further validated as diagnostic, prognostic, or predictive biomarkers.
Proteomics-based biomarker discovery has been successfully applied in a wide range of diseases, including cancer, cardiovascular disease, and neurological disorders. For example, proteomics studies have identified several promising biomarkers for early detection of cancer, prediction of drug response, and monitoring of disease progression. However, the translation of proteomics-based biomarkers into clinical practice requires rigorous validation and standardization.
3.2. Drug Target Identification
Proteomics is a valuable tool for identifying novel drug targets. By analyzing the proteomes of cells or tissues treated with different drugs, researchers can identify proteins that are modulated by the drug. These proteins can then be further investigated as potential drug targets.
Proteomics-based drug target identification has led to the discovery of several successful drugs. For example, proteomics studies have identified the epidermal growth factor receptor (EGFR) as a drug target in cancer. EGFR inhibitors, such as gefitinib and erlotinib, have become important therapies for treating lung cancer and other cancers.
3.3. Personalized Medicine
Proteomics is paving the way for personalized medicine, tailoring treatment strategies to individual patients based on their unique protein profiles. Proteomic biomarkers can be used to stratify patients based on their disease subtypes, predict their responses to treatment, and monitor their disease progression.
Personalized medicine using proteomics has shown great promise in cancer treatment. For example, proteomics can be used to identify patients who are likely to respond to specific chemotherapy regimens or targeted therapies. This information can help clinicians make more informed treatment decisions and improve patient outcomes.
3.4. Systems Biology
Proteomics is an integral component of systems biology, which aims to understand complex biological systems by integrating data from different ‘omics’ disciplines. Proteomics provides a functional readout of gene expression, complementing genomic and transcriptomic data. By integrating proteomics data with other ‘omics’ data, researchers can construct comprehensive models of cellular function and disease mechanisms.
Many thanks to our sponsor Esdebe who helped us prepare this research report.
4. Challenges in Proteomics
Despite the significant advances in proteomics technologies, several challenges remain. These challenges include:
- Sample Complexity: The proteome is highly complex, consisting of thousands of proteins with a wide range of abundances. This complexity makes it difficult to analyze the entire proteome in a single experiment.
- Dynamic Range Limitations: The dynamic range of protein abundances in biological samples is vast, spanning several orders of magnitude. This makes it difficult to detect and quantify low-abundance proteins, which may be important biomarkers or drug targets.
- Data Analysis Bottlenecks: The large amount of data generated by proteomics experiments poses a significant challenge for data analysis. Sophisticated bioinformatics tools are needed to process and interpret the data.
- Protein Identification and Quantification Accuracy: Confidence in peptide identifications is not 100% certain and can be impacted by post-translational modifications and sequence similarity. Quantification can also be affected by incomplete digestion and ion suppression.
- Reproducibility Issues: Inter-laboratory variability in experimental protocols and data analysis methods can lead to reproducibility issues in proteomics research. Standardization of protocols and development of reference materials are needed to improve reproducibility.
Many thanks to our sponsor Esdebe who helped us prepare this research report.
5. Future Directions
The field of proteomics is rapidly evolving, with continuous advancements in technologies and applications. Some of the key future directions in proteomics include:
- Advancements in Protein Analysis Technologies: Next-generation mass spectrometers with increased sensitivity, resolution, and throughput are being developed. These instruments will enable the analysis of even more complex proteomes and the detection of low-abundance proteins.
- Integration of Multi-Omics Data: Integrating proteomics data with other ‘omics’ data, such as genomics, transcriptomics, and metabolomics, will provide a more holistic understanding of biological systems. This integration will require the development of sophisticated bioinformatics tools and data integration strategies.
- Development of New Proteomics Applications: Proteomics is being applied to an increasing number of areas, including plant biology, environmental science, and food science. These new applications will drive further innovation in proteomics technologies and methodologies.
- Expanding Single-Cell Proteomics: Further advances are needed to improve throughput and sensitivity of single-cell proteomics to study more cells and to identify proteins with lower expression levels. Improved methods for isolating and processing single cells will also be important.
- Artificial Intelligence (AI) in Proteomics: Leveraging AI and machine learning approaches to improve protein identification, quantification, and biomarker discovery. This is particularly relevant in handling large and complex datasets generated by proteomics experiments.
- Standardization and Automation: Implementing standardized protocols and automated workflows to improve data reproducibility and reduce human error in proteomics experiments.
Many thanks to our sponsor Esdebe who helped us prepare this research report.
6. Conclusion
Proteomics has emerged as a powerful tool for studying protein expression, function, and interactions. The advancements in proteomics technologies and methodologies have revolutionized our understanding of biological systems and disease mechanisms. The applications of proteomics are diverse and far-reaching, including biomarker discovery, drug target identification, personalized medicine, and systems biology. Despite the challenges associated with proteomics research, the field is rapidly evolving, with continuous advancements in technologies and applications. The future of proteomics holds great promise for improving human health and well-being through the development of new diagnostics, therapeutics, and personalized medicine strategies. The continued integration of proteomics with other ‘omics’ disciplines and the adoption of AI and automation will further accelerate progress in this field. Proteomics promises to be at the forefront of biomedical advances in the future, delivering more personalized and effective healthcare strategies.
Many thanks to our sponsor Esdebe who helped us prepare this research report.
References
- Aebersold, R., & Mann, M. (2003). Mass spectrometry-based proteomics. Nature, 422(7004), 198-207.
- Anderson, L., & Seilhamer, J. (2008). A proteomics roadmap for biomarker discovery. Journal of Proteome Research, 7(7), 2145-2158.
- Carragher, N. O., & Kessler, B. M. (2012). Cancer proteomics: from identification to validation. Chemical Society Reviews, 41(7), 2571-2581.
- Geyer, P. E., Kulak, N. A., Pichler, G., Holdt, L. M., Teupser, D., & Mann, M. (2016). Proteomics of individual cells: technology and applications. Nature Methods, 13(2), 167-176.
- Graumann, J. (2013). Biological and technical variation in proteomics experiments. Journal of Proteomics, 93, 465-477.
- Gygi, S. P., Rist, B., Gerber, S. A., Tureček, F., Gelb, M. H., & Aebersold, R. (1999). Quantitative analysis of complex protein mixtures using isotope-coded affinity tags. Nature Biotechnology, 17(10), 994-999.
- Hanash, S. M. (2003). Disease proteomics. Nature, 422(6928), 226-232.
- Horgan, R. P., & Kenny, D. (2011). ‘Omic’ technologies: genomics, transcriptomics, proteomics and metabolomics. The Obstetrician & Gynaecologist, 13(3), 189-195.
- Ispas, G. D., Ifa, D. R., & Cooks, R. G. (2011). Tissue analysis by mass spectrometry. Chemical Reviews, 111(6), 3737-3765.
- Mann, M., & Jensen, O. N. (2003). Proteomic analysis. Trends in Cell Biology, 13(12), 634-639.
- Parker, C. E., Domon, B., & Salek, J. W. (2015). Proteomics and metabolomics: the state of the art. Journal of Proteome Research, 14(12), 4875-4876.
- Paulo, J. A., O’Connell, J. D., Gaun, A., Bailey, D. D., & Gygi, S. P. (2015). Single-pot, solid-phase-enhanced sample-preparation (SP3) approach for bottom-up proteomics. Nature Protocols, 10(7), 985-995.
- Pennington, S. R., & Dunn, M. J. (2001). Proteomics: from protein sequence to function. Proteomics, 1(1), 3-9.
- Petricoin, E. F., Ardekani, A. M., Hitt, B. A., Levine, P. J., Fusaro, V. A., Steinberg, S. M., … & Liotta, L. A. (2002). Use of proteomic patterns for early detection of ovarian cancer. The Lancet, 359(9306), 572-577.
- Ramaker, R., Ruijter, W., & Beekwilder, J. (2019). From omics to functions: how to interpret plant metabolomics and proteomics data. Current Opinion in Biotechnology, 56, 164-171.
- Steen, H., & Mann, M. (2004). The ABCs of proteomics. Nature Reviews Molecular Cell Biology, 5(9), 699-711.
Affinity proteomics sounds like a super targeted approach! I wonder, with the rise of personalized medicine, how close are we to having readily available “protein profiles” that can guide individual treatment plans? It feels like something out of a sci-fi movie!
That’s a great question! The potential for readily available protein profiles in personalized medicine is definitely exciting. While we’re not quite at ‘sci-fi’ levels yet, advances in high-throughput proteomics and data analysis are rapidly accelerating the development of clinically relevant protein biomarkers. Standardizing these profiles and integrating them into treatment workflows will be key!
Editor: MedTechNews.Uk
Thank you to our Sponsor Esdebe
The report mentions AI’s potential in proteomics. Could AI algorithms also address the challenge of standardizing protocols across different labs, thus improving the reproducibility of proteomics experiments and the reliability of biomarker discovery?
That’s a fantastic point! Using AI to standardize protocols is a really interesting avenue to explore. It could help us minimize variability and ensure greater consistency in biomarker validation across different research environments. What other applications could AI have in addressing standardization?
Editor: MedTechNews.Uk
Thank you to our Sponsor Esdebe
The mention of dynamic range limitations is interesting. Addressing this through novel sample preparation or data analysis techniques could significantly enhance the detection of low-abundance, but potentially critical, proteins. What emerging technologies are most promising in overcoming this hurdle?
That’s a great question! There’s a lot of exciting work being done to improve dynamic range. Developments in mass spectrometry, such as selected reaction monitoring (SRM) and data-independent acquisition (DIA), are proving very promising for more comprehensive coverage. Improved fractionation methods, like multi-dimensional liquid chromatography, are also proving valuable. It’s an area with lots of progress!
Editor: MedTechNews.Uk
Thank you to our Sponsor Esdebe