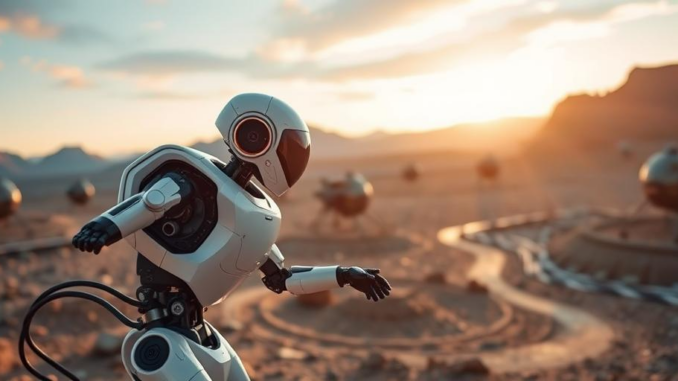
Abstract
Robotics is rapidly transforming numerous sectors, and this research report offers a comprehensive overview of its current state and future trajectories, tailored for experts in the field. Beyond the well-documented applications in manufacturing and logistics, the report delves into cutting-edge advancements across diverse domains, including healthcare, agriculture, exploration, and security. Specific attention is given to the ethical and societal implications arising from increased robot autonomy and integration into human environments. Furthermore, the report critically examines the challenges hindering widespread robot adoption, such as cost, energy efficiency, and the need for robust human-robot interaction. Finally, the paper concludes by discussing future research directions and potential breakthroughs that could revolutionize the field of robotics in the coming years.
Many thanks to our sponsor Esdebe who helped us prepare this research report.
1. Introduction
Robotics, the interdisciplinary field encompassing the design, construction, operation, and application of robots, has witnessed exponential growth in recent decades. This growth is fueled by advancements in artificial intelligence (AI), sensor technology, materials science, and computer processing power. Once confined to automated assembly lines, robots are now being deployed in increasingly complex and unstructured environments. This research report aims to provide a comprehensive overview of the current state of robotics, addressing advancements across multiple sectors, highlighting ethical considerations, and forecasting future research directions. It is targeted toward robotics experts seeking a broad understanding of the field’s trajectory, rather than a deep dive into any single sub-discipline.
Many thanks to our sponsor Esdebe who helped us prepare this research report.
2. Core Technological Advancements
2.1 Sensors and Perception
The ability of robots to perceive their environment is paramount to their functionality. Advancements in sensor technology are enabling robots to gather increasingly detailed and accurate information about their surroundings. These advancements include:
- LiDAR (Light Detection and Ranging): Solid-state LiDAR systems have become significantly smaller, cheaper, and more energy-efficient. This has broadened their applicability, particularly in autonomous vehicles and mobile robotics. Furthermore, advancements in waveform processing and signal processing algorithms have improved the accuracy and robustness of LiDAR data in challenging environments, such as those with dense vegetation or adverse weather conditions [1].
- Computer Vision: Deep learning has revolutionized computer vision, enabling robots to identify objects, recognize faces, and understand scenes with remarkable accuracy. Convolutional Neural Networks (CNNs) and their variants have become the workhorses of robot vision, and ongoing research focuses on improving their robustness to adversarial attacks and their ability to generalize to unseen data [2]. Furthermore, the development of efficient neural network architectures allows for deployment on resource-constrained platforms.
- Tactile Sensors: The integration of tactile sensors, including capacitive, resistive, and piezoelectric sensors, is allowing robots to interact with objects and environments in a more nuanced way. Advanced tactile sensing systems can provide information about force, pressure, texture, and temperature, enabling robots to perform tasks requiring dexterity and precision, such as grasping fragile objects or performing surgical procedures [3].
- Multimodal Sensing: Combining data from multiple sensors, such as LiDAR, cameras, and tactile sensors, provides robots with a more complete and robust understanding of their environment. Sensor fusion techniques, including Kalman filters and Bayesian networks, are used to integrate the data from different sensors and create a unified representation of the world [4].
2.2 AI and Machine Learning
AI and machine learning are playing an increasingly crucial role in robotics, enabling robots to learn from data, adapt to changing environments, and perform complex tasks without explicit programming. Key advancements in this area include:
- Reinforcement Learning (RL): RL algorithms allow robots to learn optimal control policies through trial and error. Deep reinforcement learning, which combines RL with deep neural networks, has shown promising results in tasks such as robot locomotion, manipulation, and navigation [5]. However, challenges remain in terms of sample efficiency, exploration, and generalization to unseen environments. Simulation-to-reality transfer learning is a key area of research to reduce the amount of real-world data needed for training.
- Imitation Learning: Imitation learning allows robots to learn from human demonstrations. This approach can be particularly useful for tasks that are difficult to define mathematically or for which a large amount of training data is not available. Recent advancements in imitation learning include techniques for handling noisy or incomplete demonstrations and for learning from multiple human demonstrators [6].
- Natural Language Processing (NLP): NLP is enabling robots to understand and respond to human language. This is crucial for human-robot interaction and for enabling robots to perform tasks based on natural language instructions. Transformer-based models have significantly improved the performance of NLP systems, and ongoing research focuses on developing more robust and context-aware language understanding capabilities [7].
- Explainable AI (XAI): As robots become more autonomous, it is increasingly important to understand their decision-making processes. XAI techniques aim to make the reasoning behind robot actions more transparent and understandable to humans. This can help to build trust in robots and to identify potential biases or errors in their decision-making [8].
2.3 Actuation and Control
The physical capabilities of robots are determined by their actuation and control systems. Advancements in these areas include:
- Soft Robotics: Soft robots are constructed from flexible materials, allowing them to deform and adapt to their environment. This makes them well-suited for tasks requiring compliance, such as grasping delicate objects or navigating confined spaces. Research in soft robotics focuses on developing new materials, actuators, and control strategies for soft robots [9].
- Advanced Actuators: New types of actuators are enabling robots to achieve greater speed, power, and precision. These include hydraulic actuators, pneumatic actuators, and electric motors. Research focuses on improving the efficiency and reliability of these actuators and on developing new types of actuators, such as shape memory alloy actuators and electroactive polymer actuators [10].
- Decentralized Control: Decentralized control algorithms allow robots to operate autonomously without relying on a central controller. This can improve the robustness and scalability of robot systems. Recent advancements in decentralized control include techniques for coordinating the actions of multiple robots and for adapting to changes in the environment [11].
2.4 Energy Efficiency and Power Management
Power management is becoming increasingly important as robots are deployed in more remote and autonomous settings. Here are some key points.
- Lightweight Materials: Development of composite materials and alloys reduce the mass of the robotic system reducing power requirements and increasing performance.
- Efficient motors and drivers: The use of new electric motors and motor controllers leads to higher efficiency and lower power consumption.
- Power Harvesting: Harvesting energy from the environment such as sunlight, vibrations, and even temperature gradients. By using these natural sources, robots are able to operate for extended periods without battery changes.
- Advanced Power Management System: These include smart charging systems, power storage optimisation, and predictive consumption models to maximise the life of batteries [12].
Many thanks to our sponsor Esdebe who helped us prepare this research report.
3. Applications Across Various Sectors
3.1 Healthcare
Robotics in healthcare extends beyond assistive robots for geriatric care. Surgical robots, like the da Vinci Surgical System, offer enhanced precision and minimally invasive procedures [13]. Rehabilitation robots aid in physical therapy, enabling patients to regain motor skills. Automated dispensing systems improve medication management and reduce errors. Further, robots are being developed for diagnostic imaging and drug delivery, targeting specific areas of the body with increased accuracy [14].
3.2 Agriculture
The agriculture sector is facing increasing pressure to produce more food with fewer resources. Robots are helping to address these challenges by automating tasks such as planting, harvesting, and weeding. Precision agriculture techniques, enabled by robots and sensors, allow farmers to optimize the use of water, fertilizer, and pesticides, leading to increased yields and reduced environmental impact [15]. Autonomous tractors and drones are also being used to monitor crops and to apply treatments in a targeted manner.
3.3 Manufacturing and Logistics
Robots have long been used in manufacturing to automate tasks such as welding, painting, and assembly. However, recent advancements in AI and sensor technology are enabling robots to perform more complex and flexible tasks. Collaborative robots (cobots) are designed to work alongside human workers, improving safety and productivity. In logistics, robots are being used to automate tasks such as warehouse picking, packing, and delivery. Autonomous mobile robots (AMRs) are able to navigate complex warehouse environments without the need for fixed infrastructure [16].
3.4 Exploration and Security
Robots are invaluable for exploration in environments that are too dangerous or inaccessible for humans. Space exploration robots, such as rovers and orbiters, are used to gather data about other planets and celestial bodies. Underwater robots are used to explore the ocean depths and to inspect underwater infrastructure. In security, robots are being used for surveillance, bomb disposal, and search and rescue operations. Drones are also being used for border patrol and to monitor critical infrastructure [17].
Many thanks to our sponsor Esdebe who helped us prepare this research report.
4. Ethical and Societal Implications
The increasing autonomy and integration of robots into human environments raise a number of ethical and societal concerns. These include:
- Job Displacement: The automation of tasks by robots has the potential to displace human workers in a variety of industries. It is important to consider the social and economic consequences of job displacement and to develop strategies for retraining and supporting workers who are affected [18].
- Bias and Discrimination: Robots are trained on data that may contain biases, leading them to make discriminatory decisions. It is important to address these biases in the data and in the algorithms used to train robots [19].
- Privacy and Surveillance: Robots equipped with cameras and sensors can collect vast amounts of data about human behavior. It is important to consider the privacy implications of this data collection and to develop regulations to protect human privacy [20].
- Autonomous Weapons: The development of autonomous weapons systems raises serious ethical concerns. Many argue that these weapons should be banned, as they could lead to unintended consequences and could lower the threshold for armed conflict [21].
- Human-Robot Relationship: As robots become more advanced, questions arise about the nature of human-robot interactions. Issues such as emotional attachment to robots, dependence, and the potential for manipulation need careful consideration [22].
Many thanks to our sponsor Esdebe who helped us prepare this research report.
5. Challenges and Limitations
Despite the significant advancements in robotics, several challenges and limitations hinder widespread adoption:
- Cost: The cost of robots and their associated infrastructure can be prohibitive for many organizations. Continued research and development are needed to reduce the cost of robots and to make them more accessible to a wider range of users.
- Complexity: Programming and maintaining robots can be complex, requiring specialized skills. Simplified programming tools and more user-friendly interfaces are needed to make robots easier to use [23].
- Energy Efficiency: Robots can consume significant amounts of energy, particularly when performing complex tasks. Improving the energy efficiency of robots is crucial for reducing their environmental impact and for enabling them to operate for longer periods of time [24].
- Safety: Ensuring the safety of humans working alongside robots is paramount. Robust safety protocols and sensor systems are needed to prevent accidents [25].
- Reliability: Robots must be reliable and able to operate without failure for extended periods of time. Improved materials and manufacturing processes are needed to enhance the reliability of robots [26].
Many thanks to our sponsor Esdebe who helped us prepare this research report.
6. Future Research Directions
The field of robotics is rapidly evolving, and several key research directions hold promise for future breakthroughs:
- Human-Robot Collaboration: Research on human-robot collaboration focuses on developing robots that can work seamlessly alongside humans, adapting to their needs and preferences [27]. This includes research on shared control, task allocation, and communication. More intuitive and natural forms of interaction, such as gesture and gaze recognition, are also areas of focus.
- Swarm Robotics: Swarm robotics explores the collective behavior of large numbers of robots working together to achieve a common goal [28]. This approach is inspired by the behavior of social insects, such as ants and bees. Research focuses on developing algorithms for decentralized control, communication, and coordination.
- Modular Robotics: Modular robotics involves the construction of robots from interchangeable modules. This allows robots to be easily reconfigured for different tasks and environments. Research focuses on developing new types of modules, as well as algorithms for self-assembly and self-reconfiguration [29].
- Robotics and Edge Computing: Integrating robotics with edge computing allows robots to process data locally, reducing latency and improving autonomy. This is particularly important for applications where real-time decision-making is critical, such as autonomous driving and industrial automation. Research focuses on developing efficient algorithms for edge computing and on integrating them with robot control systems [30].
- Bio-Inspired Robotics: Bio-inspired robotics draws inspiration from the natural world to design and build robots. This approach can lead to robots that are more efficient, adaptable, and robust. Research focuses on studying the biomechanics of animals and plants and on translating these principles into robotic designs [31].
Many thanks to our sponsor Esdebe who helped us prepare this research report.
7. Conclusion
Robotics is a dynamic and rapidly evolving field with the potential to transform numerous aspects of human life. While significant progress has been made in recent years, several challenges remain. Overcoming these challenges will require continued research and development, as well as careful consideration of the ethical and societal implications of robotics. As the field advances, it is crucial to foster collaboration between researchers, industry professionals, and policymakers to ensure that robotics is developed and deployed in a responsible and beneficial manner. The future of robotics promises to be one of innovation, collaboration, and societal transformation.
Many thanks to our sponsor Esdebe who helped us prepare this research report.
References
[1] Weinmann, M., Jutzi, B., & Hinz, S. (2015). Semantic point cloud interpretation based on optimal neighborhoods, relevant features and efficient classifiers. ISPRS Journal of Photogrammetry and Remote Sensing, 105, 46-59.
[2] LeCun, Y., Bengio, Y., & Hinton, G. (2015). Deep learning. Nature, 521(7553), 436-444.
[3] Dahiya, R. S., Metta, G., Valle, M., & Sandini, G. (2010). Tactile sensing–from humans to humanoids. IEEE Transactions on Robotics, 26(1), 1-20.
[4] Luo, R. C., Yih, C. C., & Su, K. L. (2002). Multisensor fusion and integration: state-of-the-art challenges and future research directions. IEEE Transactions on Industrial Informatics, 8(1), 2-16.
[5] Sutton, R. S., & Barto, A. G. (2018). Reinforcement learning: An introduction. MIT press.
[6] Hussein, A., Gaber, M. M., Elyan, E., & Jayne, C. (2017). Imitation learning: A survey of learning methods. ACM Computing Surveys (CSUR), 50(2), 1-35.
[7] Vaswani, A., Shazeer, N., Parmar, N., Uszkoreit, J., Jones, L., Gomez, A. N., … & Polosukhin, I. (2017). Attention is all you need. Advances in neural information processing systems, 30.
[8] Barredo Arrieta, A., Díaz-Rodríguez, N., Del Ser, J., Bennetot, A., Tabik, S., Barbado, A., … & Herrera, F. (2020). Explainable Artificial Intelligence (XAI): Concepts, taxonomies, opportunities and challenges toward responsible AI. Information Fusion, 58, 82-115.
[9] Rus, D., & Tolley, M. T. (2015). Design, fabrication and control of soft robots. Nature, 521(7553), 467-475.
[10] Carpi, F., & De Rossi, D. (2004). Dielectric elastomer actuators: New perspective for mechatronics. Mechatronics, 14(7), 769-788.
[11] Bullo, F., Cortés, J., Martínez, S., & Egerstedt, M. (2009). Distributed control of robotic networks. Princeton University Press.
[12] Koubaa, A., Qureshi, B., Boulila, W., Cheikhrouhou, O., Ghazouani, M., Jemai, A., & Abdennebi, M. (2022). Energy-Efficient Mobile Robotics: A Systematic Literature Review. IEEE Access, 10, 59638-59667.
[13] Lanfranco, A. R., Castellanos, A. E., Desai, J. P., & Meyers, W. C. (2004). Robotic surgery: a current perspective. Annals of surgery, 239(1), 14-21.
[14] Yang, G. Z., Bellingham, J., Dupont, P. E., Fischer, P., Ikkala, O., Jacobstein, N., … & Nelson, B. J. (2017). The grand challenges of Science Robotics. Science Robotics, 2(2), eaam6944.
[15] Tillett, N. D. (1996). Sensing for precision agriculture. Computers and electronics in agriculture, 14(1), 49-61.
[16] Boysen, N., Briskorn, D., & Emde, E. (2019). Warehousing with autonomous mobile robots: classification and literature review. European Journal of Operational Research, 271(1), 1-19.
[17] Murphy, R. R. (2014). Disaster robotics. MIT press.
[18] Frey, C. B., & Osborne, M. A. (2013). The future of employment: How susceptible are jobs to computerisation?. Technological Forecasting and Social Change, 114, 254-280.
[19] O’Neil, C. (2016). Weapons of math destruction: How big data increases inequality and threatens democracy. Broadway Books.
[20] Solove, D. J. (2008). Understanding privacy. Harvard University Press.
[21] Sharkey, N. (2012). Killing made easy: From remote control to autonomous weapons. Philosophical Transactions of the Royal Society B: Biological Sciences, 367(1600), 2878-2887.
[22] Turkle, S. (2011). Alone together: Why we expect more from technology and less from each other. Simon and Schuster.
[23] Billingsley, J. (2022). Robotics: A very short introduction. Oxford University Press.
[24] Shah, D., & Agogino, A. M. (2007). An energy aware framework for human robot collaborative design. IEEE/ASME Transactions on Mechatronics, 12(6), 626-636.
[25] Villani, V., Pini, F. G., Levratti, A., & Pellegrinelli, S. (2018). Survey on human–robot collaboration in manufacturing applications. International Journal of Production Research, 56(1-2), 246-275.
[26] ElMaraghy, H. A. (2005). Reliability and maintainability issues in flexible manufacturing systems. Journal of Intelligent Manufacturing, 16(6), 607-620.
[27] Ajoudani, A., Rossi, S., Biagiotti, L., Grioli, G., Rocco, P., & Prattichizzo, D. (2018). Force estimation in human-robot collaboration: Towards safe and intuitive physical interaction. IEEE Robotics and Automation Letters, 3(3), 2067-2074.
[28] Brambilla, M., Ferrante, E., Birattari, M., & Dorigo, M. (2013). Swarm robotics: a review from the swarm engineering perspective. Journal of Autonomous and Multi-Agent Systems, 26(1), 1-41.
[29] Yim, M., Shen, W. M., Salemi, B., Rus, D., Moll, M., & Lipson, H. (2007). Modular self-reconfigurable robot systems [grand challenges in robotics]. IEEE Robotics & Automation Magazine, 14(1), 43-52.
[30] Shi, W., Cao, J., Zhang, Q., Li, Y., & Xu, L. (2016). Edge computing: Vision and challenges. IEEE Internet of Things Journal, 3(4), 637-646.
[31] Kim, S., Laschi, C., & Trimmer, B. (2013). Soft robotics: a bioinspired approach to design and fabrication of flexible robots. Trends in biotechnology, 31(5), 287-294.
The discussion on ethical implications is critical. The potential for bias in robot training data, as highlighted, needs careful consideration to avoid discriminatory outcomes. What methods can ensure fairness and equity in AI-driven robotic systems, especially in areas like hiring or law enforcement?
You’re absolutely right, the ethical implications are key! The challenge of ensuring fairness in AI-driven robotics, especially in sensitive areas like hiring and law enforcement, is significant. Exploring methods for auditing training data and implementing bias detection algorithms are vital steps. Perhaps human oversight and explainable AI can also play a role?
Editor: MedTechNews.Uk
Thank you to our Sponsor Esdebe
The discussion of energy efficiency highlights a critical need. What advancements in battery technology or alternative energy sources could further extend robotic operational time and reduce environmental impact, especially in remote deployments?