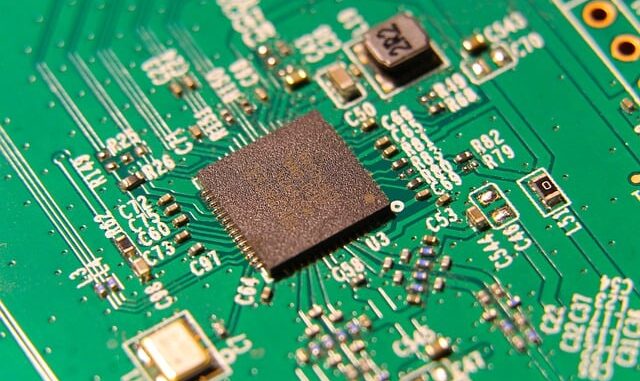
Advancements and Future Trajectories in Continuous Glucose Monitoring Technology
Abstract
Continuous Glucose Monitoring (CGM) technology has revolutionized diabetes management, offering real-time glucose data and insights beyond traditional blood glucose monitoring (BGM). While initially developed for individuals with type 1 diabetes, CGM’s utility has expanded to encompass a broader range of applications, including gestational diabetes management, improved glycemic control in type 2 diabetes, and even personalized nutrition optimization. This research report provides a comprehensive overview of CGM technology, delving into its evolution, underlying principles, diverse types, accuracy metrics, clinical benefits, limitations, and emerging trends. We explore the current state-of-the-art CGM systems, analyzing their performance characteristics and addressing challenges related to sensor longevity, calibration requirements, and data interpretation. Furthermore, we examine the advancements in non-invasive CGM technologies, integration with closed-loop systems (artificial pancreas), and the potential of CGM data for predictive analytics and personalized healthcare. The report aims to provide an in-depth understanding of the technological landscape and future directions of CGM, offering insights for researchers, clinicians, and industry stakeholders involved in diabetes care and beyond.
1. Introduction
Diabetes mellitus is a chronic metabolic disorder characterized by hyperglycemia resulting from defects in insulin secretion, insulin action, or both. The global prevalence of diabetes is steadily increasing, posing a significant burden on healthcare systems worldwide [1]. Effective glycemic control is crucial for preventing or delaying the onset of long-term complications associated with diabetes, including cardiovascular disease, nephropathy, neuropathy, and retinopathy [2].
Traditionally, blood glucose monitoring (BGM) using fingerstick blood samples has been the standard method for assessing glycemic control. While BGM provides a snapshot of glucose levels at a specific point in time, it fails to capture the dynamic fluctuations that occur throughout the day and night [3]. Moreover, frequent fingersticks can be inconvenient and painful, leading to reduced adherence to monitoring schedules.
Continuous Glucose Monitoring (CGM) has emerged as a transformative technology in diabetes management, offering a continuous stream of glucose data and providing valuable insights into glucose trends, patterns, and variability. CGM systems consist of a small sensor inserted subcutaneously that measures glucose levels in the interstitial fluid (ISF). The sensor transmits data wirelessly to a receiver or smartphone, allowing users to track their glucose levels in real-time and make informed decisions about insulin dosing, diet, and exercise [4].
This research report aims to provide a comprehensive overview of CGM technology, exploring its underlying principles, diverse types, accuracy metrics, clinical benefits, limitations, and emerging trends. The report will also delve into the advancements in non-invasive CGM technologies, integration with closed-loop systems, and the potential of CGM data for predictive analytics and personalized healthcare.
2. Principles of CGM Technology
CGM systems work by measuring glucose levels in the interstitial fluid (ISF), which surrounds the cells beneath the skin. The ISF glucose concentration is generally correlated with blood glucose levels, but with a time lag of approximately 5-15 minutes [5].
The basic components of a CGM system include:
- Sensor: A small, flexible sensor is inserted subcutaneously, typically in the abdomen or upper arm. The sensor contains an enzyme, glucose oxidase, which reacts with glucose in the ISF. This reaction produces hydrogen peroxide, which is then oxidized at an electrode, generating an electrical current proportional to the glucose concentration.
- Transmitter: The transmitter is attached to the sensor and wirelessly transmits glucose data to a receiver or smartphone at regular intervals, typically every 5-15 minutes.
- Receiver/Smartphone: The receiver or smartphone displays glucose readings, trends, and alerts. It also stores historical glucose data for analysis and reporting.
2.1 Enzymatic Biosensors
The most prevalent type of CGM sensor utilizes an enzymatic biosensor based on glucose oxidase (GOx). GOx catalyzes the oxidation of glucose to gluconic acid, producing hydrogen peroxide (H2O2) as a byproduct. The generated H2O2 is then electrochemically oxidized or reduced at an electrode, generating a measurable electrical current proportional to the glucose concentration. The reaction steps are as follows:
- Glucose + O2 → Gluconic acid + H2O2 (catalyzed by GOx)
- H2O2 → 2H+ + O2 + 2e- (Electrochemical oxidation)
The GOx enzyme is typically immobilized on the surface of the electrode to ensure stability and prevent enzyme leaching. Different immobilization techniques, such as cross-linking, entrapment, and adsorption, are employed to optimize enzyme activity and sensor performance.
2.2 Challenges in Enzymatic Biosensors
Despite their widespread use, enzymatic biosensors face several challenges:
- Oxygen Dependence: The GOx reaction requires oxygen, and variations in local oxygen tension in the subcutaneous tissue can affect sensor accuracy. Some sensors incorporate oxygen electrodes or employ alternative enzymes, such as glucose dehydrogenase (GDH), which are less oxygen-dependent, to mitigate this issue.
- Biofouling: The sensor surface can be susceptible to biofouling, the accumulation of proteins, cells, and other biological materials that can impede glucose diffusion and reduce sensor sensitivity. Anti-fouling coatings and surface modifications are used to minimize biofouling.
- Inflammatory Response: The insertion of the sensor into the subcutaneous tissue triggers an inflammatory response, which can affect sensor performance and longevity. Biocompatible materials and anti-inflammatory agents are incorporated into the sensor design to minimize the inflammatory response.
3. Types of CGM Systems
CGM systems can be broadly categorized into two main types: real-time CGM (rt-CGM) and intermittently scanned CGM (is-CGM), also known as flash glucose monitoring (FGM).
3.1 Real-Time CGM (rt-CGM)
rt-CGM systems continuously display glucose readings in real-time and provide alerts for high and low glucose levels. Users can view their glucose trends and patterns and receive notifications when their glucose levels are rising or falling rapidly. rt-CGM systems typically require calibration with fingerstick blood glucose measurements at regular intervals.
3.2 Intermittently Scanned CGM (is-CGM) / Flash Glucose Monitoring (FGM)
is-CGM systems, such as the Abbott FreeStyle Libre, do not automatically transmit glucose data to a receiver or smartphone. Users must actively scan the sensor with a reader or smartphone to view their glucose readings. is-CGM systems provide retrospective glucose data, including glucose trends and patterns, but do not provide real-time alerts for high and low glucose levels in the same way that rt-CGM systems do, although alarms based on trends are becoming more available. These systems generally require less frequent calibration compared to rt-CGM systems, with some systems requiring no calibration at all.
The key differences between rt-CGM and is-CGM systems are summarized in Table 1.
Table 1: Comparison of rt-CGM and is-CGM Systems
| Feature | rt-CGM | is-CGM/FGM |
| —————– | ——————————————— | ——————————————– |
| Glucose Readings | Continuous, Real-Time | Intermittent, Scanned |
| Alerts | High/Low Glucose Alerts | Trend-Based Alerts (becoming more common) |
| Calibration | Typically Requires Regular Calibration | Less Frequent or No Calibration Required |
| Data Transmission | Automatic, Wireless | Manual Scanning Required |
3.3 Implantable CGM
Implantable CGM systems are designed to be implanted under the skin for longer durations, typically up to several months. These systems offer the advantage of reduced sensor insertions and potentially improved accuracy due to less exposure to external factors. However, they require a minor surgical procedure for insertion and removal.
Dexcom Stelo is an example of an over-the-counter, continuous glucose monitoring system (CGM) designed for individuals who do not use insulin, including those with prediabetes. It is also approved for users who manage type 2 diabetes with oral medications. Users can wear the Stelo sensor for up to 15 days, providing them with insights into how their bodies respond to food and exercise.
4. Accuracy Metrics for CGM Systems
Accuracy is a critical performance characteristic of CGM systems. Several metrics are used to assess CGM accuracy, including:
- Mean Absolute Relative Difference (MARD): MARD is the most commonly used metric for evaluating CGM accuracy. It represents the average absolute difference between CGM glucose readings and reference blood glucose measurements, expressed as a percentage. Lower MARD values indicate better accuracy. Generally, a MARD value of less than 10% is considered acceptable for clinical use.
- Clarke Error Grid Analysis (EGA): EGA is a graphical tool that assesses the clinical significance of CGM errors. It divides the glucose range into five zones (A, B, C, D, and E) based on the potential impact of CGM errors on clinical decision-making. Zone A represents clinically accurate readings, while zones D and E represent readings that could lead to dangerous clinical decisions.
- Consensus Error Grid (CEG): CEG is a modified version of EGA that addresses some of the limitations of the original EGA. CEG provides a more refined assessment of CGM accuracy and clinical relevance.
- Root Mean Square Error (RMSE): RMSE is another statistical measure of CGM accuracy that quantifies the average magnitude of the errors between CGM glucose readings and reference blood glucose measurements.
Several factors can affect CGM accuracy, including:
- Sensor Calibration: Accurate sensor calibration is essential for ensuring CGM accuracy. Inaccurate calibration can lead to systematic errors in glucose readings.
- Inter-Individual Variability: CGM accuracy can vary between individuals due to differences in physiological factors, such as skin thickness, hydration status, and inflammatory response.
- Sensor Insertion Site: The sensor insertion site can also affect CGM accuracy. Areas with poor blood flow or excessive subcutaneous fat may result in less accurate readings.
- Sensor Age: CGM accuracy may decline over time as the sensor ages due to biofouling, enzyme degradation, or other factors.
5. Clinical Benefits of CGM
CGM offers several clinical benefits compared to traditional BGM, including:
- Improved Glycemic Control: CGM provides real-time glucose data and alerts, enabling users to make more informed decisions about insulin dosing, diet, and exercise, leading to improved glycemic control, as measured by HbA1c levels [6].
- Reduced Hypoglycemia: CGM alerts can warn users of impending hypoglycemia, allowing them to take corrective action before their glucose levels drop too low. This is particularly beneficial for individuals with hypoglycemia unawareness [7].
- Increased Time in Range (TIR): TIR refers to the percentage of time that glucose levels are within a target range, typically 70-180 mg/dL. CGM allows users to track their TIR and make adjustments to their diabetes management plan to increase their TIR [8]. Increased TIR has been shown to correlate strongly with reduced risk of long term complications.
- Enhanced Understanding of Glucose Patterns: CGM provides a comprehensive view of glucose trends and patterns, allowing users to identify factors that affect their glucose levels, such as meals, exercise, stress, and medications [9].
- Personalized Diabetes Management: CGM data can be used to personalize diabetes management plans based on individual needs and preferences. Healthcare providers can use CGM data to tailor insulin regimens, dietary recommendations, and exercise plans to optimize glycemic control [10].
- Improved Quality of Life: CGM can reduce the burden of diabetes management and improve quality of life by providing real-time glucose data, reducing the need for frequent fingersticks, and empowering users to take control of their diabetes [11].
6. Limitations of CGM
Despite its numerous benefits, CGM also has some limitations:
- Cost: CGM systems can be expensive, which may limit access for some individuals.
- Calibration Requirements: Some CGM systems require regular calibration with fingerstick blood glucose measurements, which can be inconvenient and painful.
- Sensor Lag: There is a time lag between ISF glucose levels and blood glucose levels, which can lead to inaccuracies, especially during periods of rapid glucose change.
- Skin Irritation: Some individuals may experience skin irritation or allergic reactions at the sensor insertion site.
- Data Overload: The continuous stream of glucose data can be overwhelming for some users, especially those new to CGM. Education and support are essential to help users interpret and utilize CGM data effectively.
- Accuracy Limitations: CGM accuracy is not perfect, and readings may sometimes deviate from actual blood glucose levels. It is important for users to understand the limitations of CGM and to confirm readings with a fingerstick blood glucose measurement when necessary, especially when making critical treatment decisions.
- Sensor Failures: Sensors can sometimes fail or malfunction, requiring replacement. This can be frustrating for users and may interrupt data collection.
7. Future Trends in CGM Technology
CGM technology is rapidly evolving, with several promising advancements on the horizon:
7.1 Non-Invasive CGM
Non-invasive CGM (ni-CGM) is a highly sought-after technology that aims to measure glucose levels without requiring skin penetration. Several approaches are being explored, including:
- Spectroscopic Techniques: Spectroscopic techniques, such as Raman spectroscopy, near-infrared (NIR) spectroscopy, and mid-infrared (MIR) spectroscopy, measure the interaction of light with glucose molecules in the skin or other tissues. These techniques can potentially provide non-invasive glucose measurements, but they are still in the early stages of development.
- Reverse Iontophoresis: Reverse iontophoresis uses a small electrical current to extract ISF through the skin for glucose measurement. This technique has shown some promise, but it can be affected by skin impedance and other factors.
- Ultrasonic Techniques: Ultrasonic techniques use sound waves to measure glucose levels in the skin or other tissues. These techniques are non-invasive and potentially low-cost, but they are still under development.
The development of accurate and reliable non-invasive CGM systems remains a significant challenge, but the potential benefits are enormous.
7.2 Integration with Closed-Loop Systems (Artificial Pancreas)
Closed-loop systems, also known as artificial pancreas systems, integrate CGM with an insulin pump and a control algorithm to automatically regulate insulin delivery based on real-time glucose data. These systems aim to mimic the function of a healthy pancreas and provide automated glycemic control [12].
Several hybrid closed-loop systems are currently available, which require some user input, such as meal announcements. Fully automated closed-loop systems, which require no user input, are under development.
The integration of CGM with closed-loop systems has the potential to significantly improve glycemic control and reduce the burden of diabetes management.
7.3 Miniaturization and Extended Sensor Lifespan
Future CGM systems are expected to be smaller, more discreet, and have longer sensor lifespans. Miniaturization will improve user comfort and reduce the visibility of the device. Extended sensor lifespans will reduce the frequency of sensor insertions and improve convenience [13].
7.4 Enhanced Data Analytics and Predictive Capabilities
CGM data is a rich source of information that can be used to develop advanced data analytics and predictive capabilities. Machine learning algorithms can be trained to identify patterns in CGM data and predict future glucose levels, allowing users to take proactive steps to prevent hyperglycemia or hypoglycemia [14].
CGM data can also be integrated with other health data, such as activity data, sleep data, and dietary information, to provide a more comprehensive view of an individual’s health and well-being.
7.5 Integration with Mobile Health (mHealth) Platforms
Future CGM systems are expected to be seamlessly integrated with mobile health (mHealth) platforms, allowing users to access their glucose data, track their progress, and communicate with their healthcare providers through their smartphones or other mobile devices. mHealth platforms can also provide personalized education and support to help users manage their diabetes more effectively [15].
8. Ethical Considerations
The increasing use of CGM technology raises several ethical considerations:
- Data Privacy and Security: CGM data is sensitive personal information that must be protected from unauthorized access and misuse. Robust data privacy and security measures are essential to safeguard user data.
- Data Ownership and Control: Users should have control over their CGM data and be able to decide how it is used and shared.
- Equity of Access: CGM technology should be accessible to all individuals who could benefit from it, regardless of their socioeconomic status or geographic location.
- Algorithmic Bias: The algorithms used to analyze CGM data and make treatment recommendations should be free from bias and should not discriminate against any particular group of individuals.
- Over-Reliance on Technology: It is important to avoid over-reliance on technology and to maintain a balance between technology and human judgment in diabetes management.
9. Conclusion
Continuous Glucose Monitoring (CGM) technology has revolutionized diabetes management, offering real-time glucose data and insights beyond traditional blood glucose monitoring (BGM). CGM systems provide numerous clinical benefits, including improved glycemic control, reduced hypoglycemia, increased time in range, enhanced understanding of glucose patterns, personalized diabetes management, and improved quality of life.
Despite its limitations, CGM technology is rapidly evolving, with several promising advancements on the horizon, including non-invasive CGM, integration with closed-loop systems, miniaturization, extended sensor lifespan, enhanced data analytics, and integration with mobile health platforms. These advancements have the potential to further improve diabetes management and reduce the burden of this chronic disease.
As CGM technology continues to advance, it is important to address the ethical considerations related to data privacy, data ownership, equity of access, algorithmic bias, and over-reliance on technology. By addressing these challenges, we can ensure that CGM technology is used responsibly and effectively to improve the health and well-being of individuals with diabetes.
References
[1] International Diabetes Federation. IDF Diabetes Atlas, 10th ed. Brussels, Belgium: International Diabetes Federation, 2021.
[2] American Diabetes Association. Standards of Medical Care in Diabetes—2023. Diabetes Care 2023;46(Suppl 1):S1–S291.
[3] Clarke, W. L., & Foster, R. B. (2012). A history of blood glucose meters and strips. Diabetes Technology & Therapeutics, 14(Suppl 1), S-4-S-17.
[4] Heinemann, L., & Freckmann, G. (2015). Continuous glucose monitoring in people with diabetes: an overview of systems, evaluation and interpretation of results. Diabetologia, 58(3), 408-417.
[5] Rebrin, K., Steil, G. M., Van Antwerp, W. P., & Mastrototaro, J. J. (1999). Subcutaneous glucose predicts plasma glucose independent of insulin: implications for continuous glucose monitoring. American Journal of Physiology-Endocrinology and Metabolism, 277(3), E561-E571.
[6] Beck, R. W., Riddlesworth, T. D., Ruedy, K. J., Ahmann, A. J., Bergenstal, R. M., Kollman, C., … & Tamborlane, W. V. (2017). Continuous glucose monitoring versus usual care in patients with type 2 diabetes uncontrolled on oral agents. Annals of Internal Medicine, 167(6), 365-374.
[7] Grunberger, G., Abelseth, J. M., Bailey, T. S., Bode, B. W., Handelsman, Y., Hellman, R., … & Zimmerman, R. S. (2016). Consensus statement by the American Association of Clinical Endocrinologists and American College of Endocrinology on the Comprehensive Type 2 Diabetes Management Algorithm—2016 Executive Summary. Endocrine Practice, 22(3), 383-384.
[8] Bergenstal, R. M., Beck, R. W., Riddlesworth, T., Freckmann, G., Haller, M. J., Pratley, R. E., … & Dubose, S. N. (2013). Glycemic variability: a consensus statement of the American Diabetes Association and the European Association for the Study of Diabetes. Diabetes Care, 36(11), 3641-3648.
[9] Battelino, T., Danne, T., Bergenstal, R. M., Amiel, S. A., Beck, R., Biester, T., … & Group, C. T. (2019). Clinical targets for continuous glucose monitoring data interpretation: recommendations from the international consensus on time in range. Diabetes Care, 42(8), 1593-1603.
[10] Vigersky, R. A., McMahon, C. (2019). The importance of glucose metrics other than HbA1c in diabetes. Clinical Diabetes, 37(2), 117-125.
[11] Polonsky, W. H., Hessler, D. M., Ronsley, R., Beebe, C. A., Wagner, J., & Lawrence, D. B. (2021). Continuous glucose monitoring and quality of life among individuals with type 1 and type 2 diabetes: A systematic review and meta-analysis. Journal of Diabetes Science and Technology, 15(4), 953-962.
[12] Bekiari, E., Kitsios, K., Thabit, H., Tauschmann, M., Akturk, H. K., & Weghuber, D. (2018). Artificial pancreas treatment for outpatients with type 1 diabetes: systematic review and meta-analysis. BMJ, 361, k1310.
[13] Christiansen, M. P., Garg, S. K. (2020). Continuous glucose monitoring: current use and future directions. Diabetes Technology & Therapeutics, 22(S1), S-1-S-14.
[14] Patek, S. D., Shah, A., Rice, M., Sayuk, G., & Hood, M. (2019). Application of machine learning techniques for prediction of glucose levels in patients with type 1 diabetes using continuous glucose monitoring data. Journal of Diabetes Science and Technology, 13(2), 254-263.
[15] Quinn, C. C., Gruber-Baldini, A. L., Shardell, M., Terrin, M. L., & Rebok, G. W. (2011). Mobile diabetes intervention study: feasibility of a randomized controlled trial. Diabetes Technology & Therapeutics, 13(4), 377-385.
Beyond the advancements in non-invasive methods, how might the integration of CGM data with other wearable sensor data (e.g., sleep trackers, activity monitors) contribute to a more holistic and personalized understanding of an individual’s metabolic health?