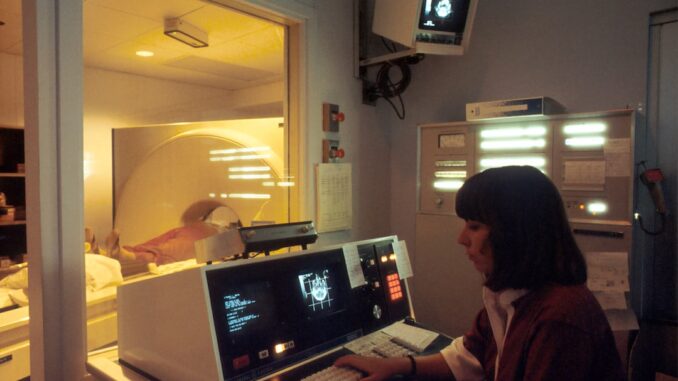
Advancements in Neuroimaging: A Synergistic Convergence of AI, Portable MRI, and Clinical Impact
Abstract
Neuroimaging has revolutionized the diagnosis and treatment of neurological disorders, providing unprecedented insights into brain structure and function. This research report explores the cutting-edge advancements in neuroimaging techniques, with a particular emphasis on the integration of artificial intelligence (AI) for image reconstruction and analysis, the development of portable magnetic resonance imaging (MRI) systems, and their combined impact on clinical practice. The report delves into the market dynamics of neuroimaging, identifies key players, analyzes technological innovations, and examines the regulatory landscape governing these transformative technologies. It further discusses the potential of these advancements to improve patient outcomes, particularly in underserved and resource-constrained settings, while acknowledging the challenges associated with validation, standardization, and ethical considerations. Finally, we offer an expert opinion on the potential risks of these systems, particularly around robustness, bias, and the deskilling of clinical experts.
1. Introduction
Neuroimaging encompasses a diverse range of techniques used to visualize the structure, function, and biochemistry of the brain and nervous system. These techniques are crucial for diagnosing and monitoring neurological disorders, guiding surgical interventions, and advancing our understanding of brain development, aging, and cognition. Traditional neuroimaging modalities, such as computed tomography (CT) and conventional MRI, have limitations in terms of accessibility, cost, portability, and radiation exposure. Recent advancements in AI, coupled with the miniaturization of MRI technology, are poised to overcome these limitations and usher in a new era of neuroimaging.
The convergence of AI and portable MRI systems has the potential to transform clinical practice by enabling rapid, point-of-care neuroimaging in diverse settings, including emergency departments, intensive care units, and remote rural areas. AI-powered image reconstruction algorithms can enhance image quality, reduce scan times, and facilitate automated image analysis, while portable MRI systems offer increased accessibility and affordability compared to conventional MRI scanners.
This research report aims to provide a comprehensive overview of these advancements, examining their technological underpinnings, clinical applications, market dynamics, and regulatory landscape. It will also address the challenges and opportunities associated with their implementation and explore their potential impact on patient care and neurological research.
2. Recent Developments in Neuroimaging Techniques
2.1 AI-Powered Image Reconstruction and Analysis
Artificial intelligence, particularly deep learning, has emerged as a powerful tool for enhancing neuroimaging techniques. AI algorithms can be trained to perform a variety of tasks, including image reconstruction, image segmentation, lesion detection, and disease classification. In image reconstruction, AI algorithms can significantly reduce scan times by reconstructing high-quality images from undersampled data [1]. This is particularly beneficial for MRI, where long scan times can lead to patient discomfort and motion artifacts. Generative adversarial networks (GANs) and convolutional neural networks (CNNs) are commonly used for this purpose [2]. For example, a study by Knoll et al. (2020) demonstrated that a deep learning-based reconstruction algorithm could reduce MRI scan times by a factor of four without compromising image quality [3].
AI-powered image analysis tools can also automate the process of identifying and quantifying pathological changes in neuroimaging data. This can improve diagnostic accuracy, reduce inter-rater variability, and facilitate large-scale neuroimaging studies. For instance, AI algorithms can be trained to detect subtle brain atrophy patterns associated with Alzheimer’s disease or to identify white matter lesions indicative of multiple sclerosis [4]. Automated segmentation of brain structures, such as the hippocampus and amygdala, is also crucial for volumetric analysis and longitudinal monitoring of disease progression.
2.2 Portable MRI Systems
Conventional MRI scanners are typically large, expensive, and require specialized infrastructure, limiting their accessibility in many healthcare settings. Portable MRI systems offer a compact and affordable alternative, enabling point-of-care neuroimaging in a wider range of clinical environments. These systems are typically based on low-field MRI technology, which reduces the size and cost of the scanner while still providing clinically useful images [5].
One notable example is the Swoop® portable MRI system developed by Hyperfine, which is designed to be easily transported and operated at the patient’s bedside [6]. This system utilizes a low-field magnet and AI-powered image reconstruction algorithms to produce diagnostic-quality images of the brain. Portable MRI systems have the potential to improve access to neuroimaging in emergency departments, intensive care units, and remote rural areas, where conventional MRI scanners are not readily available [7]. They also offer the advantage of faster scan times and reduced patient transport, leading to improved workflow efficiency and patient comfort. The trade-off, however, is generally lower image resolution compared to traditional high-field MRI, requiring careful consideration of the clinical application.
2.3 Multimodal Neuroimaging
Combining different neuroimaging modalities can provide a more comprehensive picture of brain structure and function. For example, simultaneous EEG-fMRI allows researchers to correlate electrical brain activity with hemodynamic responses [8]. PET-MRI combines the high sensitivity of PET with the excellent anatomical resolution of MRI, enabling the simultaneous assessment of metabolic activity and structural changes [9]. These multimodal approaches can provide valuable insights into the pathophysiology of neurological disorders and improve diagnostic accuracy. New advances are focusing on simultaneous acquisition and AI-driven integration of multimodal data to improve clinical understanding.
3. Clinical Impact on Diagnosis and Treatment of Neurological Disorders
3.1 Stroke Diagnosis and Management
Rapid neuroimaging is crucial for the diagnosis and management of acute stroke. CT scans are typically used to rule out hemorrhagic stroke, while MRI can provide more detailed information about the extent of ischemic damage. Portable MRI systems offer the potential to accelerate stroke diagnosis in emergency departments and mobile stroke units, allowing for faster initiation of thrombolytic therapy or endovascular thrombectomy [10]. AI-powered image analysis tools can also assist in the rapid assessment of stroke severity and the identification of patients who are most likely to benefit from reperfusion therapies [11].
3.2 Traumatic Brain Injury (TBI)
Neuroimaging plays a critical role in the diagnosis and management of TBI. CT scans are commonly used to detect acute intracranial hemorrhage and skull fractures, while MRI can identify more subtle brain injuries, such as diffuse axonal injury and contusions. Portable MRI systems can facilitate the rapid assessment of TBI patients at the point of injury, enabling faster triage and treatment decisions [12]. AI algorithms can also be used to automatically detect and quantify TBI lesions, improving diagnostic accuracy and reducing inter-rater variability [13].
3.3 Neurodegenerative Diseases
Neuroimaging is essential for the diagnosis and monitoring of neurodegenerative diseases, such as Alzheimer’s disease, Parkinson’s disease, and multiple sclerosis. MRI can detect brain atrophy, white matter lesions, and other structural changes associated with these diseases. PET scans can measure metabolic activity and amyloid deposition in the brain, providing insights into the underlying disease processes [14]. AI-powered image analysis tools can assist in the early detection of neurodegenerative diseases by identifying subtle brain changes that may not be apparent to the human eye [15]. Longitudinal neuroimaging studies can also be used to track disease progression and assess the effectiveness of therapeutic interventions.
3.4 Brain Tumors
Neuroimaging is indispensable for the diagnosis, staging, and treatment planning of brain tumors. MRI provides detailed anatomical information about the tumor location, size, and relationship to surrounding structures. Advanced MRI techniques, such as diffusion-weighted imaging and perfusion imaging, can provide information about tumor cellularity and angiogenesis [16]. AI algorithms can assist in the segmentation of brain tumors, the prediction of tumor grade, and the assessment of treatment response [17]. Portable MRI may have limited immediate applicability in detailed tumor characterization but could play a role in monitoring for hydrocephalus or gross changes post-operatively in resource-limited environments.
3.5 Pediatric Neuroimaging
Neuroimaging is widely used in pediatrics to diagnose and manage a variety of neurological conditions, including congenital malformations, developmental delay, seizures, and brain tumors. Portable MRI systems offer several advantages in the pediatric population, including reduced anxiety and the ability to perform scans at the bedside without the need for sedation [18]. AI-powered image reconstruction algorithms can also reduce scan times, minimizing the need for sedation in young children. Careful consideration must be given to radiation exposure when using CT in pediatric patients, further emphasizing the value of MRI when feasible.
4. Market Size and Growth
The global neuroimaging market is experiencing significant growth, driven by the increasing prevalence of neurological disorders, the aging population, and technological advancements in neuroimaging techniques. According to a report by Grand View Research, the global neuroimaging market size was valued at USD 8.49 billion in 2022 and is projected to reach USD 13.45 billion by 2030, growing at a CAGR of 5.9% from 2023 to 2030 [19].
The key factors driving market growth include the rising incidence of stroke, TBI, and neurodegenerative diseases, as well as the increasing demand for non-invasive diagnostic procedures. The growing adoption of AI-powered neuroimaging tools and portable MRI systems is also contributing to market expansion. Furthermore, government initiatives to improve healthcare infrastructure and increase access to neuroimaging services are expected to further fuel market growth.
The COVID-19 pandemic had a mixed impact on the neuroimaging market. While the pandemic led to a temporary decline in elective neuroimaging procedures, it also highlighted the importance of neuroimaging in the diagnosis and management of COVID-19-related neurological complications. The pandemic also accelerated the adoption of telemedicine and remote monitoring technologies, which may further drive the demand for portable neuroimaging solutions.
5. Key Players in the Neuroimaging Market
The neuroimaging market is highly competitive, with a number of established players and emerging companies vying for market share. Some of the key players in the neuroimaging market include:
- Siemens Healthineers: A leading provider of medical imaging equipment, including MRI, CT, and PET/CT scanners.
- GE Healthcare: Another major player in the medical imaging market, offering a comprehensive portfolio of neuroimaging solutions.
- Philips Healthcare: A global leader in healthcare technology, providing a wide range of neuroimaging equipment and software.
- Canon Medical Systems: A leading provider of CT, MRI, and ultrasound systems.
- Hyperfine: A pioneering company specializing in portable MRI systems.
- NeuroLogica Corporation (a subsidiary of Samsung): A provider of mobile CT scanners and other neuroimaging solutions.
- Fujifilm Healthcare: Offers a diverse portfolio including AI-driven image analysis tools.
These companies are investing heavily in research and development to develop innovative neuroimaging technologies and expand their product portfolios. They are also actively pursuing collaborations and partnerships to strengthen their market position and expand their reach.
6. Technological Advances in Neuroimaging
6.1 Ultra-High Field MRI
Ultra-high field MRI scanners (7 Tesla and above) offer improved signal-to-noise ratio and spatial resolution compared to conventional MRI scanners. This allows for the visualization of finer details of brain structure and function, enabling the detection of subtle pathological changes that may not be visible on lower-field scanners [20]. Ultra-high field MRI is being used in research settings to study brain connectivity, neurotransmitter activity, and the pathophysiology of neurological disorders.
6.2 Advanced Diffusion Imaging
Diffusion imaging techniques, such as diffusion tensor imaging (DTI) and diffusion kurtosis imaging (DKI), can provide information about the microstructure of white matter. These techniques are used to study white matter integrity in neurological disorders, such as multiple sclerosis, TBI, and Alzheimer’s disease [21]. Advanced diffusion imaging techniques, such as neurite orientation dispersion and density imaging (NODDI), can provide more detailed information about the orientation and density of neuronal fibers, enabling a more comprehensive assessment of white matter pathology.
6.3 Molecular Neuroimaging
Molecular neuroimaging techniques, such as PET and single-photon emission computed tomography (SPECT), use radioactive tracers to visualize specific molecules in the brain. These techniques can be used to study neurotransmitter systems, receptor binding, and metabolic activity. Molecular neuroimaging is used in research settings to study the pathophysiology of neurological disorders and to develop new diagnostic and therapeutic agents [22].
6.4 Functional Near-Infrared Spectroscopy (fNIRS)
fNIRS is a non-invasive neuroimaging technique that uses near-infrared light to measure changes in blood flow in the brain. fNIRS is a portable and relatively inexpensive technique that can be used to study brain activity in real-world settings [23]. fNIRS is being used in research settings to study cognitive processes, social interactions, and motor control.
7. Regulatory Landscape
The regulatory landscape for neuroimaging devices and software is complex and varies across different countries. In the United States, the Food and Drug Administration (FDA) regulates the safety and effectiveness of medical devices, including neuroimaging equipment. Manufacturers of neuroimaging devices must obtain FDA clearance or approval before marketing their products in the United States [24].
AI-powered neuroimaging software is also subject to regulatory oversight. The FDA has issued guidance on the regulation of AI/ML-based software as a medical device, emphasizing the need for transparency, validation, and ongoing monitoring. The European Union also has regulations governing medical devices, including neuroimaging equipment and software. The Medical Device Regulation (MDR) sets out stricter requirements for the safety and performance of medical devices, including AI-based devices.
Data privacy and security are also important considerations in the regulation of neuroimaging. The Health Insurance Portability and Accountability Act (HIPAA) in the United States and the General Data Protection Regulation (GDPR) in the European Union set out requirements for the protection of patient data. Neuroimaging data must be stored and processed securely to protect patient privacy.
8. Challenges and Opportunities
8.1 Validation and Standardization
One of the major challenges in the field of neuroimaging is the lack of standardization across different imaging centers and equipment. This can lead to variability in image quality and quantification, making it difficult to compare results across different studies. Efforts are underway to develop standardized neuroimaging protocols and quality control procedures to improve the reproducibility and comparability of neuroimaging data. AI-driven tools require rigorous validation on diverse datasets to ensure generalizability and prevent bias.
8.2 Data Privacy and Security
Neuroimaging data contains sensitive information about individuals, including their brain structure, function, and cognitive abilities. Protecting the privacy and security of neuroimaging data is essential to prevent unauthorized access and misuse. Secure data storage and transmission protocols must be implemented to ensure the confidentiality of patient information. The use of federated learning, where AI models are trained on decentralized data without sharing the raw data, is a promising approach to address data privacy concerns.
8.3 Ethical Considerations
The use of neuroimaging raises a number of ethical considerations, including the potential for discrimination based on brain characteristics, the misuse of neuroimaging data for employment or insurance purposes, and the impact of neuroimaging on individual autonomy and identity. Careful consideration must be given to these ethical issues to ensure that neuroimaging is used responsibly and ethically. As AI advances, it is essential to address potential biases in algorithms and ensure fairness in their application to neuroimaging data.
8.4 Access and Affordability
Neuroimaging services are not readily accessible in many parts of the world, particularly in low- and middle-income countries. The high cost of neuroimaging equipment and the lack of trained personnel are major barriers to access. Portable MRI systems and AI-powered image analysis tools offer the potential to reduce the cost of neuroimaging and improve access in underserved areas. However, efforts are needed to ensure that these technologies are affordable and accessible to all who need them.
8.5 Integration with Clinical Workflow
The successful implementation of new neuroimaging technologies requires seamless integration with existing clinical workflows. This includes training healthcare professionals on the use of new equipment and software, developing efficient data management systems, and ensuring that neuroimaging results are readily available to clinicians. AI-powered tools should be designed to augment, rather than replace, the expertise of clinicians, providing decision support and improving diagnostic accuracy.
9. Expert Opinion on Potential Risks
While AI and portable imaging offer transformative potential, there are risks that require careful consideration:
- Robustness and Generalizability: AI models, particularly deep learning models, can be brittle and prone to failure when applied to data that differs significantly from the training data. This is a major concern in neuroimaging, where patient populations and imaging protocols can vary widely across different centers. Overfitting to specific datasets can lead to inaccurate diagnoses and treatment decisions in real-world clinical settings. Rigorous validation on diverse datasets and the use of techniques like transfer learning and domain adaptation are crucial to ensure the robustness and generalizability of AI-powered neuroimaging tools.
- Bias and Fairness: AI algorithms can perpetuate and amplify existing biases in the data they are trained on. If the training data is not representative of the population being served, the AI model may make biased predictions that disproportionately harm certain groups. For example, if the training data for a stroke detection algorithm is primarily from older white males, the algorithm may be less accurate when applied to younger patients or patients from other racial or ethnic groups. Addressing bias in AI requires careful attention to data collection, preprocessing, and model evaluation. It also requires ongoing monitoring and auditing to identify and mitigate bias in real-world clinical settings.
- Deskilling of Clinical Experts: The increasing reliance on AI-powered neuroimaging tools may lead to the deskilling of clinical experts, such as radiologists and neurologists. If clinicians become overly reliant on AI-generated results, they may lose their ability to critically evaluate images and make independent judgments. This could have negative consequences for patient care, particularly in complex cases where AI algorithms may not be reliable. To prevent deskilling, it is essential to ensure that AI tools are used to augment, rather than replace, the expertise of clinicians. Training programs should emphasize the importance of critical thinking and independent judgment, even when using AI-powered tools.
- Over-reliance on low-field imaging: Portable MRI offers clear accessibility benefits, but it’s essential to avoid over-reliance, particularly if lower image quality leads to missed diagnoses or incomplete characterization of conditions. Training is needed to ensure clinicians can differentiate between what can and cannot be adequately assessed using this technology.
10. Conclusion
Neuroimaging is undergoing a period of rapid innovation, driven by the convergence of AI and portable MRI technologies. These advancements have the potential to transform clinical practice by improving access to neuroimaging, accelerating diagnosis, and enhancing treatment planning. AI-powered image reconstruction algorithms can improve image quality and reduce scan times, while portable MRI systems offer increased accessibility and affordability. The combination of these technologies has the potential to improve patient outcomes in a variety of neurological disorders, including stroke, TBI, neurodegenerative diseases, and brain tumors. As with any new technology, it’s crucial to be aware of potential biases and ensure correct usage in a real clinical setting. The neuroimaging market is expected to continue to grow in the coming years, driven by the increasing prevalence of neurological disorders, the aging population, and technological advancements. Despite the challenges associated with validation, standardization, and ethical considerations, the future of neuroimaging is bright, with the potential to revolutionize the diagnosis and treatment of neurological disorders and improve the lives of millions of people.
References
[1] Wang, S., et al. “Deep learning for fast magnetic resonance imaging: A review.” Magnetic Resonance Imaging, 68 (2020): 116-132.
[2] Zhu, B., et al. “Image reconstruction by domain-transform manifold learning.” Nature, 555.7697 (2018): 487-492.
[3] Knoll, F., et al. “Assessment of the generalization of learned image reconstruction across anatomy with a prospective human study.” Magnetic Resonance in Medicine, 84.1 (2020): 435-447.
[4] Bron, E. E., et al. “Standardized evaluation system for Alzheimer’s disease neuroimaging: The MIRIAD toolbox.” NeuroImage, 61.4 (2012): 1337-1350.
[5] Cooley, C. Z., et al. “Low-field MRI: physics, hardware, and clinical applications.” American Journal of Roentgenology, 216.1 (2021): 18-35.
[6] Hyperfine. (n.d.). Swoop Portable MR Imaging System. Retrieved from https://hyperfine.io/
[7] Sheth, K. N., et al. “Feasibility of Portable Magnetic Resonance Imaging in the Intensive Care Unit.” JAMA Neurology, 77.6 (2020): 728-734.
[8] Laufs, H., et al. “Electroencephalographic signatures of attentional and cognitive default-mode processing in humans.” Proceedings of the National Academy of Sciences, 100.19 (2003): 11053-11058.
[9] Shah, N. J., et al. “Advances in simultaneous PET/MRI imaging.” Journal of Nuclear Medicine, 54.9 (2013): 1481-1492.
[10] Tayal, A. H., et al. “Prospective Feasibility Study of Low-Field Portable MRI for Acute Stroke Imaging in Mobile Stroke Units.” Stroke, 52.1 (2021): 309-312.
[11] Yoo, A. J., et al. “Artificial intelligence in stroke imaging.” Stroke, 51.4 (2020): 1302-1310.
[12] Diaz-Arrastia, R., et al. “Diagnosis and Management of Traumatic Brain Injury.” JAMA, 318.4 (2017): 363-376.
[13] Bigler, E. D. “Quantitative Magnetic Resonance Imaging in Traumatic Brain Injury.” Journal of Neurotrauma, 30.1 (2013): 1-20.
[14] Jack, C. R., Jr., et al. “NIA-AA Research Framework: Toward a biological definition of Alzheimer’s disease.” Alzheimer’s & Dementia, 14.4 (2018): 535-562.
[15] de Lange, E. E., et al. “Machine learning in neuroimaging for neurodegenerative disorders: current status and challenges.” European Radiology Experimental, 5.1 (2021): 1-11.
[16] Law, M., et al. “Gliomas: diagnosis and grading using perfusion imaging.” Journal of Magnetic Resonance Imaging, 20.5 (2004): 815-828.
[17] Akkus, Z., et al. “Deep learning for brain MRI segmentation: state of the art and future directions.” Journal of Digital Imaging, 30.4 (2017): 449-459.
[18] Krishnamoorthy, K. S., et al. “Portable Low-Field Magnetic Resonance Imaging of the Neonatal Brain.” Pediatric Neurology, 115 (2021): 1-8.
[19] Grand View Research. (2023). Neuroimaging Market Analysis Report By Modality (MRI, CT, PET, SPECT, Ultrasound), By Application (Neurology, Spine, Oncology), By End-use (Hospitals, Diagnostic Centers), By Region, And Segment Forecasts, 2023 – 2030. Retrieved from https://www.grandviewresearch.com/industry-analysis/neuroimaging-market
[20] Marques, J. P., Kober, T., Krueger, G., van der Zwaag, W., Van de Moortele, P. F., & Gruetter, R. (2010). MP2RAGE, a self bias-field corrected sequence for improved segmentation and T1-mapping at high field. NeuroImage, 49(2), 1271–1281.
[21] Alexander, A. L., Lee, J. E., Lazar, M., Field, A. S. (2007). Diffusion tensor imaging of the brain. Nature Reviews Neuroscience, 8(4), 327-39.
[22] Villemagne, V. L., Rowe, C. C. (2011). Molecular imaging of Alzheimer’s disease: amyloid-β and beyond. The British journal of radiology, 84(Spec No 2), S271–S280.
[23] Ferrari, M., Quaresima, V. (2012). A brief review on the history of human functional near-infrared spectroscopy (fNIRS) development and fields of application. NeuroImage, 63(2), 921-35.
[24] U.S. Food and Drug Administration. (n.d.). Medical Devices. Retrieved from https://www.fda.gov/medical-devices
The discussion of bias in AI algorithms is critical. As these algorithms become more integrated into neuroimaging, how can we proactively ensure diverse datasets are used for training, thereby mitigating potential disparities in diagnosis and treatment across different demographic groups?
That’s a fantastic point! Addressing bias proactively is key. One promising avenue is collaborative data sharing initiatives, ensuring datasets reflect the global population. We can also explore techniques like federated learning to train models across diverse, decentralized datasets without compromising privacy. What other strategies do you think are important?
Editor: MedTechNews.Uk
Thank you to our Sponsor Esdebe
This is a great overview of the advances and challenges in neuroimaging. The discussion of deskilling clinical experts is particularly important. To prevent this, perhaps integrating AI training directly into medical education and continuing professional development could ensure clinicians maintain core skills while leveraging new technologies.
Thank you for your comment! I completely agree that integrating AI training into medical education and professional development is crucial. Perhaps simulation-based training could allow clinicians to practice interpreting AI-enhanced images and making informed decisions, thereby retaining core skills and leveraging the tech effectively. What do you think about the use of simulators?
Editor: MedTechNews.Uk
Thank you to our Sponsor Esdebe
Deskilling, really? If clinicians are so easily replaced by algorithms, were they *skilled* to begin with? Perhaps the focus should be on augmenting expertise, not fearing obsolescence. How about robust training in understanding AI limitations *and* human cognitive biases?
This is a very important discussion point. I agree completely; augmentation is the key. Training should definitely incorporate an understanding of human cognitive biases. This is perhaps more broadly applicable in many areas of medicine and worth exploring in depth. Thanks for raising this!
Editor: MedTechNews.Uk
Thank you to our Sponsor Esdebe
Regarding the integration of AI with portable MRI, how can we best address concerns about clinicians potentially over-relying on lower image quality and subsequently missing subtle but crucial diagnostic details? What training strategies could mitigate this risk?
That’s a key point! Standardized reporting templates tailored to portable MRI could be beneficial. These templates would guide clinicians, prompting them to consider specific, potentially subtle, features despite lower resolution, ensuring nothing is missed. This approach could also aid in consistent communication across teams. What are your thoughts?
Editor: MedTechNews.Uk
Thank you to our Sponsor Esdebe
Deskilling? If AI augmentation means fewer billable hours staring at the same scan, sign me up! Maybe we can finally focus on, you know, *patients* instead of just pixels?
That’s a great perspective! Re-focusing on patients is definitely the goal. If AI can handle some of the more tedious tasks, clinicians can invest more time in direct patient interaction and personalized care. This could really revolutionize how we approach neurological care.
Editor: MedTechNews.Uk
Thank you to our Sponsor Esdebe
Deskilling, you say? Sounds like the robots are coming for our jobs! On the bright side, maybe I can finally convince my boss that “diagnosing” Netflix shows counts as professional development using fNIRS…
That’s hilarious! fNIRS for Netflix diagnostics – I love the creativity! Perhaps with the right algorithm, we can quantify the precise neurological impact of a plot twist or a cliffhanger. Imagine the insights into viewer engagement! It opens up some interesting perspectives on user experience.
Editor: MedTechNews.Uk
Thank you to our Sponsor Esdebe